FindTB
"Missing Patients" are a huge challenge for any Tuberculosis eradication program.These are patients who do not show up on TB surveillance programs because either they do not seek care or are not reported adequately.Since TB incidence rates depend on many factors like low socio-economic status, poor living conditions, proximity to other patients etc.(a lot of which are spatially co-related) ,based on the location of reported cases, we to spatially predict the geographical areas that should have a higher probability of TB patients.Our solution geo tags the current patients and predicts the incidence rates that should be expected from areas within the city/villages, villages within a district and districts within a state.Any underreporting from such areas flags out "missing patients". This can help programs streamline funding towards areas of under reporting instead of carpet investing in activities across all geographies.This increases the case detection rate and eventually TBincidence.
“Every year, approximately 10 million people fall ill with tuberculosis, but only 6 million are identified. The rest are “missing”– undiagnosed, untreated or unreported to the health systems. Missing TB cases and drug-resistant TB are challenges in fighting the disease, and pose a threat to global health security.” - The Global Fund
Despite significant progress in diagnosis and treatment of tuberculosis over the past 2 decades, millions of patients with tuberculosis go unreported every year.Organizations like the Global Fund, WHO, STOP TB partnership have implemented different strategies for finding missing patients. These included: i) reaching missing people through expanded private sector engagement; ii) community engagement/active case finding/paediatric TB and contact tracing; iii) facility based screening etc
Factors that influence TB include socio economic factors, poor housing conditions and proximity to patients among other factors. We have observed in the field that officials use nonformal methods like board pins and maps to mark areas in which patients reside and then plan awareness campaigns and other methods to find the "missing patients" . Our solution creates a statistical probability map on top of a geographical map to predict where these patients are most likely to be found, thus streamlining efforts and resources.
The end beneficiary is the TB patient and the society as a whole, but the direct user of the application are program managers of National Tuberculosis programs and other NGOs who are the decision makers of WHERE to intervene with TB interventions. We will help identify the localities and locations where the current interventions themselves can be carried out with maximum ROI.
Our solution has 2 parts.
1) The FindTB app : This application is a mobile app which can be used by the frontline health worker, in this case the DOTS provider who is entrusted with the daily observation that the patient takes his medication, to maintain the profile of the patient and GEO TAG his address.
2) The FindTB portal : The portal is a web application which collects all the geotags from an area of interest and based on the same creates a probability map on top of a regular map to identify the probable incidence (per 100,000) of the disease in other areas within the map (exhibit below). If the number of reported cases are lower than the number of predicted cases, there is a high probability that those areas can be explored further to find more TB patients. Every time more patients are added to the map from the mobile apps, the probability map changes based on new information . This dynamic nature of the map can not only be used to identify new areas which would deliver more TB patients , but also time trends can be seen in terms of which areas are the ones where most MDR_TB patients are identified and appropriate action be initiated.
This technique has been previously employe in the mining industry to identify how far from a mine should they keep digging to find the required mineral content.
- Prevent infectious disease outbreaks and vector-borne illnesses
- Prototype
- New application of an existing technology
This technology has previously used in the mining industry for predicting mineral levels in the soil around a mine to allow decisions of mine expansion. We are simply repurposing the same for disease mapping.
In a resource constrained setting, it is optimal that all resources be targeted well. Additionally, in the age of intense mobile penetration and GIS capabilities, the task of identifying areas to intervene based on proven parameters should not be missed.
Kriging is the core technique being used here. It is one of several methods that use a limited set of sampled data points to estimate the value of a variable over a continuous spatial field. An example of a value that varies across a random spatial field might be average monthly ozone concentrations over a city, or the availability of healthy foods across neighborhoods. It uses the spatial correlation between sampled points to interpolate the values in the spatial field: the interpolation is based on the spatial arrangement of the empirical observations, rather than on a presumed model of spatial distribution.
- Indigenous Knowledge
- Behavioral Design
- Social Networks
We have field experience from India and Bangladesh. What was observed was that people already use a manual similar technique wherein they use paper maps and board pins to locate the patients address in a small area of jurisdiction to see the spatial patterns of distribution of the disease and then make admin decisions on the same. We are simply taking that existing behavior and making it easy to implement and read.
- Infants
- Elderly
- Rural Residents
- Peri-Urban Residents
- Urban Residents
- Very Poor/Poor
- Low-Income
- Middle-Income
- Minorities/Previously Excluded Populations
- Refugees/Internally Displaced Persons
- Bangladesh
- India
- Bangladesh
- India
Currently we have developed the POC on dummy data variables and are approaching the Central TB Division of India to create a POC from real data on the ground. After the Pilot we intend to "
1) Within 1 yr : Train the central govt team to operate the software. Train at least all 35 state level teams to operate the software, who in turn would be encouraged to train the district and below people based on our own training materials. India in itself has a burden of 1.7 million new and relapse cases (2015)
2) Within 5 yrs: We intend to use the same model in Bangladesh, Sri Lanka, Myanmar and Sierra leone. These are countries we have current inroads into. We intend to move faster if we get a buy in from key multilaterals and partnerships like END TB and STOP TB. Just Bangladesh has about 200,000 new and relapse cases (2015)
1) Within 1 yr : Train the central govt team to operate the software. Train at least all 35 state level teams to operate the software, who in turn would be encouraged to train the district and below people based on our own training materials. India in itself has a burden of 1.7 million new and relapse cases (2015)
2) Within 5 yrs: We intend to use the same model in Bangladesh, Sri Lanka, Myanmar and Sierra leone. These are countries we have current inroads into. We intend to move faster if we get a buy in from key multilaterals and partnerships like END TB and STOP TB. Just Bangladesh has about 200,000 new and relapse cases (2015)
In the next year, some foreseeable challenges:
Data : We have created a POC based on dummy data but we need real field level data to make a stronger case for the same.
Financial : We would need funds not only to build a level of automation in the software, but also training materials in video format wherein in India, multilingual support is expensive.
In the next 5 years:
we intend to put additional layers on top which include air pollution, availability of fresh food, density of govt schemes etc to also include other factors which influence the entire prognosis of the disease as well. In such a case, the decision makers would be more than just the TB department and the solutions would need to be created in convergence of other govt departments and NGOs.
Expanding into new countries will also have its own challenges
Data : Currently we have developed the POC on dummy data variables and are approaching the Central TB Division of India to create a POC from real data on the ground
Financial : We are using mixed methods to raise money for the development of the software
Since members of our team work within the MoHFW in India, we should be able to address some of the political will issues but we will also reach out to the Global Fund and other stakeholders to generate buy in
- Not registered as any organization
We are currently 2 people working on the same. Both members part time. We have a development team who we consult from time to time pro bono for inputs on the software.
Debanshu is a Public Health expert and works with the MoHFW in India with the eHealth division as a Public Health Consultant. In the past he has worked extensively in TB and HIV in India and Bangladesh and has covered almost all corners of both nations on field. He has also worked for The Global Fund as a client. He brings in the Public health planning and operations expertise as well as his knowhow of the TB implementation system.
Aditya is a Senior Scientist at the Center for Science and Environment with the statistical expertise for geospatial analysis. He has extensive knowledge on the core technology and brings in the understanding of the feasibility and limitations of the same. He has used the core technology in the past for mining exercises.
We intend to partner with the Global Fund and the Central TB division in India. We have worked with them in the past and are currently engaging them in the discussion regarding:
1) CTD : To provide real data from pilot to test the software
2) GF : Funding , implementation support and design inputs for human centered design of app and portal
Key Customers : 1) Managers of National TB programs of the govt 2) Managers and Country directors of TB programs of multilaterals like Global Fund
Business Model : The software will be open source and free to use . However, for maintaining the data on the server, storing time trends, there will be a subscription fee to number of patients registered. But ideally we intend to fund the development through raising grants and then recurring costs will be only on the maintenance.
Once the model is proven, the same technology can be used to identify water scarcity within cities, heat zones, air pollution, etc, all from crowdsourced data. We intend to build that capability and then monetize the same with commercial applications of it.
Once the model is proven, the same technology can be used to identify water scarcity within cities, heat zones, air pollution, etc, all from crowdsourced data. We intend to build that capability and then monetize the same with commercial applications of it.
Each of the products can generate dashboards for municipalities which can be sold. They can also be connected to other map based applications. For eg: What if your Uber can decide the best route not on traffic but on air quality? These real time data collection points can influence products on how we experience our own cities to be healthy while we do it.
Visibility, Mentorship and Funding. Getting selected in a platform like this definitely opens more doors for conversations with potential clients. Mentorship will go a long way to engage us in more red team discussions and challenging us to come up with more innovative approaches to the same issue or repurpose the tech for newer avenues. Funding is definitely the nudge needed for the leap of faith.
- Business model
- Technology
- Funding and revenue model
- Monitoring and evaluation
- Media and speaking opportunities
Global Fund, STOP TB Partnership and END TB Partnership : To access their geographies. These partnerships have deep faith of the government and are known for their commitment towards TB. They can help with inputs on the design and feasibility of the product as well as implementation political will in their regions of influence.
PATH : Has been working in public health tech in many influential ways. They can provide further technical expertise and suggest revenue models for good.
AI will be built in to the application in the next version.
Mobile app : The patient management mobile application which the DOTS provider will be using will have capabilities to identify which patients are at a higher risk of "low compliance" to drug adherence based on alternative sources of information like income, family size, occupation etc.
Web Portal : The accuracy of prediction will be improve based on inputs from the ground. At the same time we would be able to map migrating populations, factors influencing surge of MDR TB cases etc
Densely populated slums are also the hubs for disease transmission. The app can use social media influencers in areas of high predictive incidence to initiate awareness campaigns and amplify the program efforts
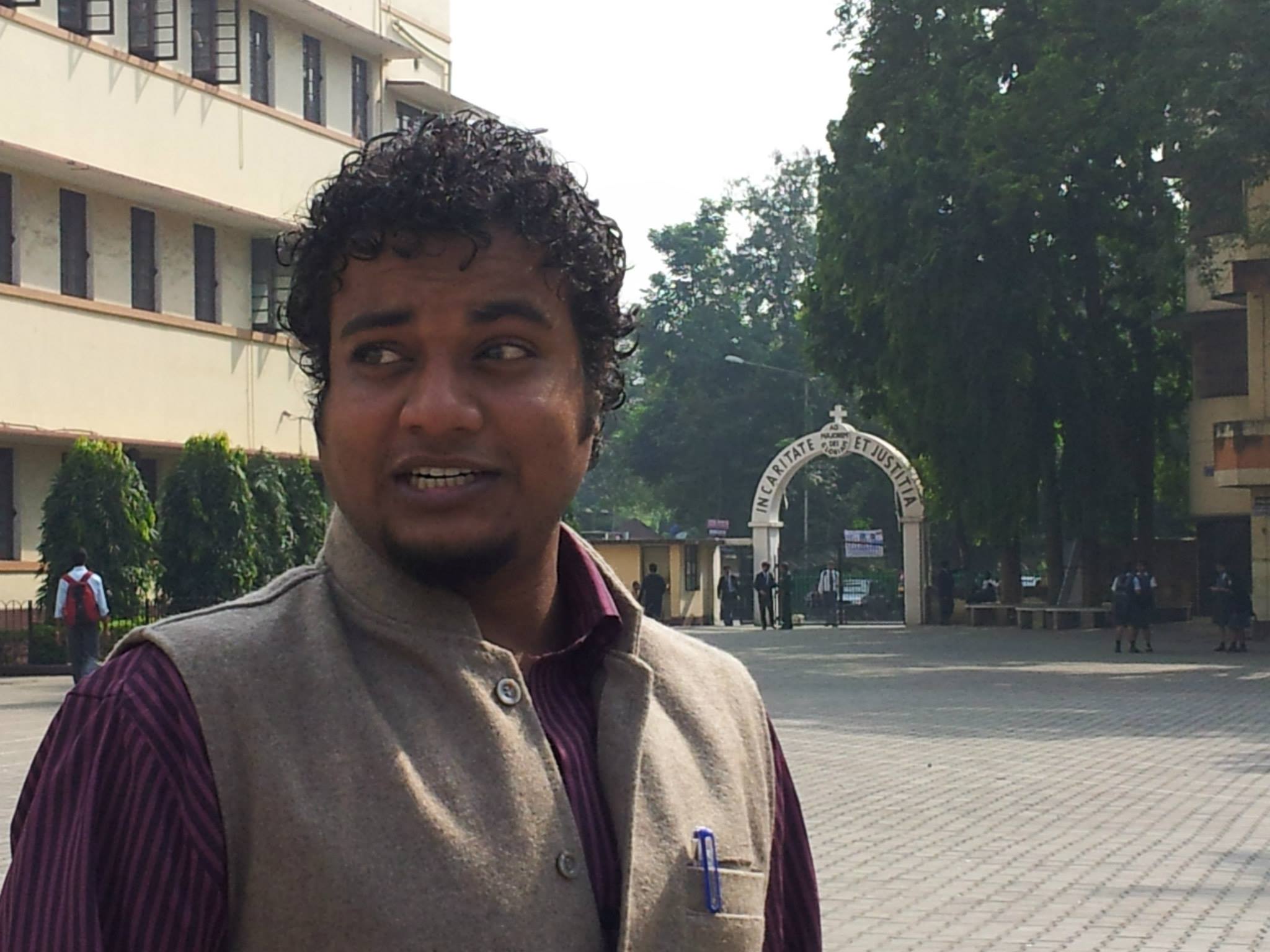
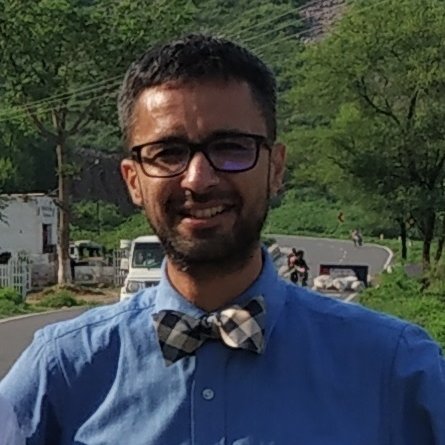
Founder-Director