ChemistryMap
Currently, only major or very expensive elements are recovered in metallurgical recycling of scrap. This is due to the complex nature of scraps - they contain easily >30 elements in significant portions while mined ores contain 10-15. If we want to recover all elements, we need to be able to PREDICT how each and every element interact with each other when injected in a metallurgical aggregate. Today we cannot. We only have approximately half the thermodynamic data needed- after 100 years of research! Here, we use experimentally known data, quantum mechanically calculated data and machine learning to create the ChemistryMap - the tool to help metallugists navigate in chemical space. Together with our state-of-the-art modelling tools we will be able to predict where each and every element will end up during metallurgical recycling - and thus how to recover them.
In a circular economy, products should be recycled 100%. The smartphone, car and household waste have probably in common that they contain roughly half of the periodic table of elements. A different way of putting the idea of a circular economy: We want to be able to recover each and every element from products by 100%.
There is a simple technical reason why this is impossible to date: Engineers do not know how to do it! Today, it is only possible to predict how smelter operation affects the partitioning of the most dominant elements (e.g. Cu,Fe,Zn,Pb,S,O in copper smelting) between slag, liquid metal and liquid sulfide since thermochemical data for these elements has been evaluated by scientists based on 100 years of experiments. For many minor elements experimental data is missing. This is due to the combinatoral catastrophy of chemical space: Each element adds a dimensionality. This generation does not have time to wait until all experiments are conducted and evaluated. Therefore, we will identify new routes to enable quantative estimates of ALL elemental interactions in the periodic table - and thus how we can recover each and every element during recycling.
Engineers around the world aready use our products to understand metallurgical processes, to improve ressource and energy efficiency or design completely new processes and materials. Today they are able to model the flow of the major elements in a complete metallurgical process. We will enable them to expand this to all elements in the periodic table. Ultimately, this will help avoiding landfill of residues and decrease the need to mine ores - both will help the quality of life of all people living close to metallurgical plants and mines.
We interact lively with engineers and scientists to improve our databases and to enable an ever growing group of users access to our databases through various interfaces.
We are using quantum mechanically calculated data from open databases generated in the Materials Genome Initiative (materialsproject.org, oqmd.org, aflowlib.org). We own and have access to the largest thermochemical databases relevant for metallurgical applications (approx. 10000 phases known). We benchmark the quantum mechanically calculated data against these and use machine learning models to extrapolate the quantum mechanically calculated data from 0K to relevant temperatures. This results in much larger databases - our latest development contains data for 100000 phases! We have so far focused on metallic systems and will continue with slag and sulfide systems.
At the same time, we have recently developed thermochemistry-based process models that are able to describe the dynamic, non-equilibrium processes in pyrometallurgical reactors. This enables us to readily include the new thermochemical data covering the whole periodic table of elements in these process models.
- Enable recovery and recycling of complex products
- Prototype
- New technology
There are several innovations:
- Use of high throughput quantum mechanically calculated and (over decades) humanly curated data extracted from experiments in machine learning of materials' thermochemical properties.
- First time high throughput scaling of high throughput quantum mechanically calculated data from 0K to relevant temperatures.
- First time use of quantum mechanically calculated data in metallurgical applications to predict behavior of rare elements.
We have created machine learning models relying on regression and
artificial annealing to scale thermodynamic data from 0K to relevant
temperatures.
We use our software tools FactSage and SimuSage for process modelling. Here, a metallurgical aggregate is sub-divided into smaller zones that are assumed to be in equilibrium. Material flow between these zones is modelled, resulting in a description of a complex, non-equilibrium process based on connected local equilibria method.
- Artificial Intelligence
- Machine Learning
- Big Data
Most metallurgical companies already use our software products and the associated thermodynamic databases to model how adjusting processing parameters of their smelter, converter or fumer will affect the distribution of the major elements between metal, slag, sulfide and gas phase in the process. "I would like to order a database that contains reliable thermdynamic data for all elements in the periodic table" is a regular customer request, that we cannot serve so far. We therefore expect that our new database will be used in metallurgical industry and that regulatory push will force metallurgical companies to increase the amount of recycled elements while decreasing the amount of residues currently landfilled. Thus, we hope that 50 years from now desasters like the red mud spilling in Brazil in 2018 will not repeat.
- Rural Residents
- Germany
- Germany
We serve a few thousand scientists and engineers with our software products and databases.
We will continue to do so.
How many people benefit from the solution? I find it hard to give numbers, so let me give examples:
- A person living close to a metallurgical plant that can decrease the amount of pollution to the environment as well as the amount of landfill residue.
- A metallurgical company that can use the ChemistryMap to tailor the flow of elements in the plant, thereby increasing productivity and flexibility in handling complex ressources and scraps while decreasing energy consumption and production of hazardous by-products.
- A worker in a metallurgical company that can be better protected from dangerous working conditions by engineers who better understand the processes.
- A consumer who cares for sustainable production of their electronic devices - as well as efficient recycling of the same.
Within the next year, we want to finish the development of a thermodynamic database of all metallic systems. We will then continue the work on oxide slag, sulfides, and the gas phase resulting in a complete database that allows estimating thermochemistry of all elements in metallurgical processes five years from now.
We will continue with salts that are relevant for the energy transition (e.g. solar salt, phase change materials as thermal storage).
We need funding to develop the databases.
We need attention of more and more decision makers in companies.
We need the regulatory push from all nations to make it attractive for companies to invest in education of their personel and software products needed for the recycling of ever larger amounts of materials.
We are actively and often successfully applying for funding of national and EU projects. The latest project QuaResPro enables us to develop a prototype application (a thermochemistry based model of a lead smelter including the thermochemical description of several elements based on quantum mechanical methods).
- Other e.g. part of a larger organization (please explain below)
The solution is developed by a team that works for GTT-Technologies. GTT-Technologies is a small enerprise based in Germany. The main software product FactSage is a Co-development of GTT-Technologies and Thermfact, Canada.
Two full-time staff persons are working on this, assissted by two student workers, the former Managing Director of GTT-Technologies and myself who is investing every "free" minute in the development.
We understand limitation of experimental results as well as of quantum mechanical calculations.
We have access to all data needed.
We already sell the software tools and databases used by metallurgists to navigate in chemical space spanned by the main components of the application. Our clients are the main users of our solution which would allow them to additionally treat the minor elements from complex ressources and scraps.
We develop the process model of the lead smelter together with the Helmholtz Institute for Ressource Technology (Prof. Reuter).
The main software product FactSage is a Co-development of GTT-Technologies and Thermfact, Canada.
We have sales representatives and agents all around the world.
We already sell software and databases to the metallurgical industry. Our software is already used by all major steel, copper, aluminium, magnesium and lead producers.
For smaller and less R&D active potential customers we will sell ready-made process models or consulting that will make our ChemistryMap available.
The solution developed here is scalable to other sectors, e.g. energy conversion (searching for new battery materials, heat storage salts etc.).
Most thermodynamic databases have been developed using at least partly public funding. As the database developed based on machine learning and quantum mechanics becomes more and more mature, database license sales will be able to sustain further developments and support.
1. We want to raise awareness for the challenges associated with navigating in ~100 dimensional chemical space. Thus we want to attract smart young people to this exciting field.
2. We want to learn about other ways of doing the machine learning. We have access to the best possible data, we have a very exciting challenge and we already have excellent results using our home-written machine learning algorithms.
3. We want the public to be more aware of the challenges that metallurgists face and we want more metallurgists to be aware of the opportunities that good thermochemical data and software give.
- Technology
- Distribution
- Monitoring and evaluation
- Media and speaking opportunities
Developers of open quantum mechanical databases, i.e.: materialsproject.org, oqmd.org, aflowlib.org
We have already developed a machine learning algorithm for the scaling of thermochemical properties from 0K to relevant temperatures, so we know already about opportunities and challenges associated both with using experimentally based and quantum mechanically calculated data.
We want to include artificial intelligence to benchmark our calculation results for systems that are well covered in experiments against the experimental results. This requires calculations that go beyond standard computing ressources and porting our calculation abilities to cloud based solutions.
Our solution has the goal to equip engineers with a map of chemical space that will enable them to navigate elemental flow in metallurgical reactors. Therefore, it enables the metallurgical community to shift towards a more circular economy through zero waste. We believe that this has a huge impact since our target group are ALL metal producers and hence ALL metal that is used around the world.
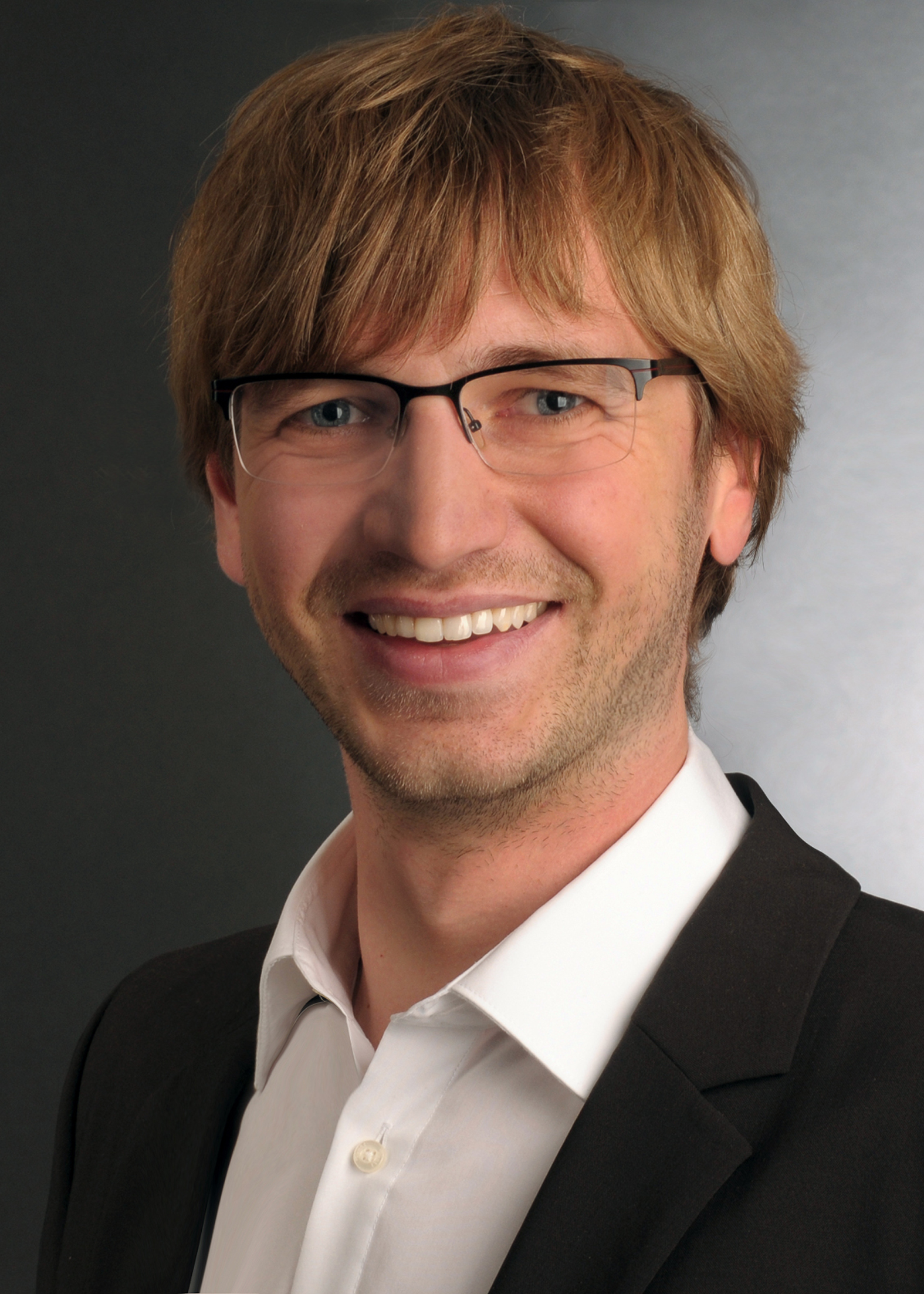
Managing Director