Waste recognition AI to improve & automate recycling
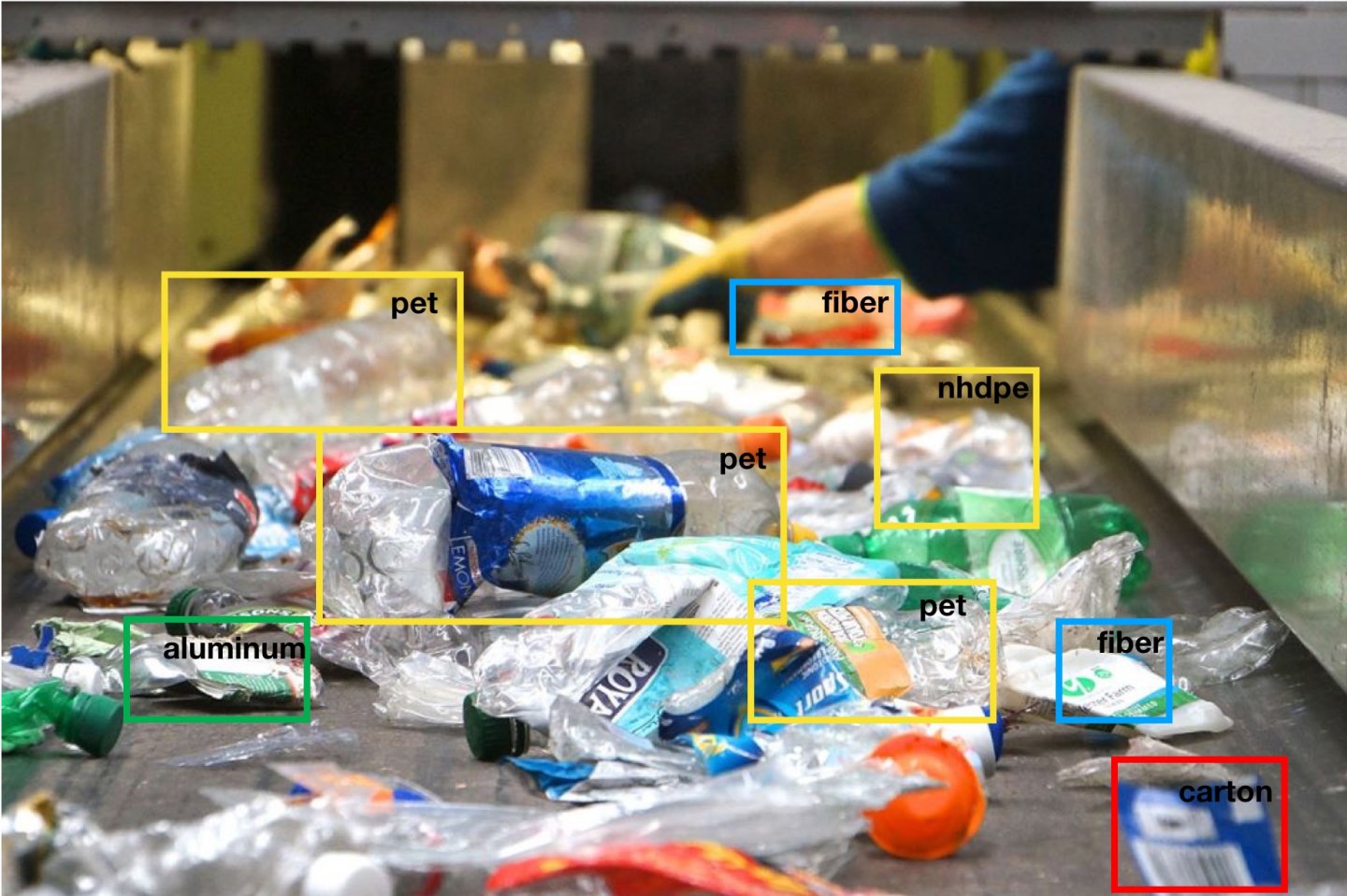
Our goal is to be the leader in computer vision for the waste management industry. Our waste recycling recognition API allows identification of rubbish types which can:
1. Provide insights for collection companies to inform and direct waste flows, saving time and resources (fuel)
2. Assist municipalities and local councils in targeted education and incentive schemes through analysis of waste collected and identification of contamination
3. Integrate with robotics at MRFs (Material Recovery Facilities) to automate the sorting process which is currently done manually by hard to recruit for workers due to the unpleasant nature of the task
4. Support EPR, Extended Producer Responsibility initiatives by tracking waste and automating the sorting process, allowing brands to reuse and recycle their packaging and products more effectively
We continue to explore where the technology can add value.
We are looking to contribute to the circular economy challenge, specifically the recycling of complex products.
Currently, recycling is broken. Owing to product design and inefficient waste management systems, “only 14% of the world’s plastic packaging is collected for recycling and just 2% is recycled” (WEF, 2019). Within the UK, “rejected recyclable waste has increased by 84% since 2011” (BBC, 2016). Developed countries in the west (US, Canada, UK) have traditionally sent their trash to China as it is costly to recycle locally. Two years ago China banned imports (2017) of rubbish which was then redirected to Southeast Asia which is also restricting imports. There is pressure to increase efficiency in recycling locally.
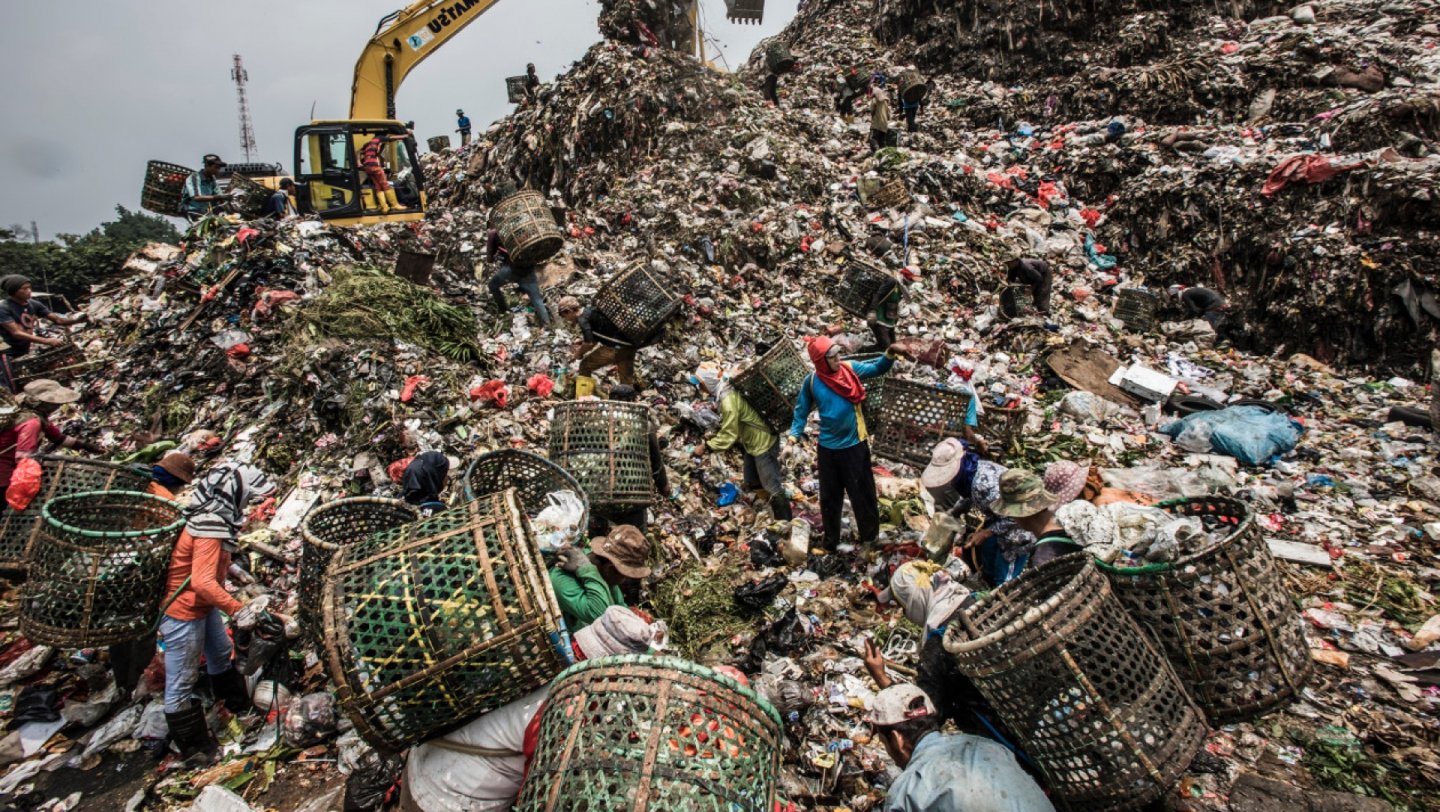
Research by VeoliaUK & Imperial College reveals the waste sector has the potential to single-handedly save over 200 m tonnes of carbon emissions btw the years 2023-2032.
There is an opportunity to develop cutting edge computer vision products for a variety of waste sorting tasks in robots, smart-bins and apps. In developed countries, this allows the introduction of automation for manual tasks in quality control and waste analysis. For developing countries, it will allow them to leapfrog to decentralised and cheaper systems to deal with increasing waste flows.
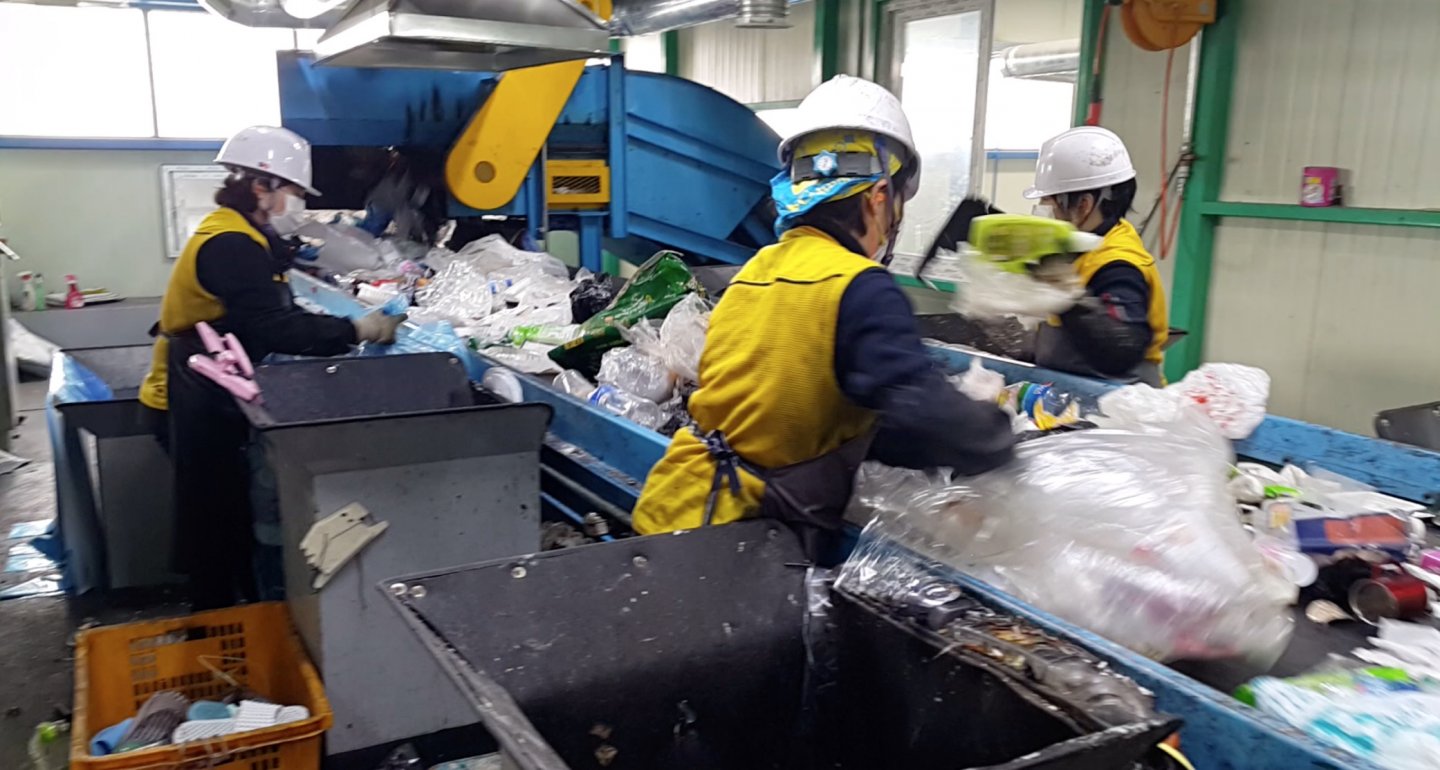
With significant advances in deep learning to solve complex visual tasks and the decline in cost of robotics, there is an opportunity to develop cutting edge computer vision products for a variety of waste sorting tasks in robots, smart-bins and apps in an economically viable way.
Computer vision (also known as machine vision) can analyse image and visual data inputs to label the item and inform the decision or action.
A quick demonstration of an early prototype can be found here
- Enable recovery and recycling of complex products
- Pilot
- New application of an existing technology
Waste recognition technology is largely limited to two use cases: smart bins and sorting of rubbish. The recognition software is embedded into existing machines, hardware, apps and platforms. Users must buy the full solution. For example, the waste sorting recognition software is embedded in sorting machines which cost up to $300,000 per unit. We believe we can provide not only better capabilities with our computer vision software but also reduce dependence on hardware. Our vision is to develop waste recognition APIs to be applied across the waste management sector that is not tied to a particular hardware or platform and that can be integrated with existing or new systems.
Drawing on the latest research in deep learning we apply this to computer vision problems to identify and classify objects and then react to what the machine “sees”. Our approach to deep learning is to reduce data requirements using transfer, active and semi-supervised techniques.
Pillars of our technology includes: 1. In-house proprietary DL framework - Based on PyTorch - Accent on deployment and R&D efficiency - Easy to integrate latest research 2. Proprietary research to reduce false-positives - Deployed in our waste demo - Best choice for our use-case, even among latest research ones
3. Hardware for custom-imaging Planned deployment through 2 key partnerships
Our capabilities: 1. Segmentation - The process of associating each pixel of an image with an object type 2. Image classification - Classify images belonging to one of pre-defined categories 3. Object detection - Detects the bounding box of a certain class (e.g. bottle, plastic, cardboard)
Our offering:
1. Recognition - We select and apply the best approach to train deep learning models General waste classifier - API: - Plastics classifier - PET vs any other clear plastic - Cans classifier - Can bottles vs any other aluminium - Glass classifier - Glass bottles vs pieces glass - Other: we developed new categories as per clients’ requests 2. Deployment - We deploy on cloud, on device or on premise 3. Maintenance - We track and optimise performance over time
- Artificial Intelligence
- Machine Learning
Our initial target market are Material Recovery Facilities in developed nations for the automation of human sorting of rubbish types as part of quality control. We have partnerships in the UK and South Korea to optimise the recycling process from collection to sorting. Our partner has identified this being a major operational issue as it is an unpleasant task and difficult to recruit for.
There is an opportunity to develop cutting edge computer vision products for a variety of waste sorting tasks in robots, smart-bins and apps. Deep learning techniques are particularly suited to this high-noise environment. A perfect deep learning/computer vision task is one where it takes humans less than a second to make a decision based on visual information. This is exactly what happens in the sorting process of rubbish or at collection points - a person looks at the rubbish and makes an expert decision in less than a second before taking action.
- Women & Girls
- Pregnant Women
- LGBTQ+
- Children and Adolescents
- Infants
- Elderly
- Rural Residents
- Peri-Urban Residents
- Very Poor/Poor
- Low-Income
- Middle-Income
- Minorities/Previously Excluded Populations
- Refugees/Internally Displaced Persons
- Persons with Disabilities
- Korea South
- United Kingdom
- Germany
- Sweden
- Korea South
- United Kingdom
- Germany
- Sweden
We are currently running 2 pilots with commercial partners and expect to have 3 B2B clients in one year.
In 5 years, we expect to have two large strategic partnerships with global leaders in waste management that operate 100+ facilities in each country as well as 50+ independent waste management companies.
We aim to contribute to UN sustainability environmental goals. Our goal is to be the leader in computer vision for the waste management sector.
Next year: Data collection, Choosing the exact use case to prioritise, Finding initial partnerships.
Next 5 years: Finding the right investors to fund our growth, establishing strategic partnerships with established players.
Gathering data - Partnering with MRFs, councils and innovative collection companies to gather data
Use case - Gaining insight from investors, advisors and industry experts as to how to navigate the product-market fit in the most effective way - Collaboration with university - current collaborations include Imperial College London, London Business School with 8 students on a project to help establish painpoints and solutions within the industry
Funding - Gaining insight from advisors and accelerators we are in (i.e London & Partners) regarding the next round of funding. We are lucky to have received initial interest from VCs and angel investors. We do not need further funding till mid 2020.
- For-Profit
6 full time
Our team has extensive experience building best-in-class computer vision for enterprises. Greyparrot was founded by ex-Blippar computer vision team with a track record of building world leading recognition systems - verified as no. 1 by Cambridge University, 2017. We believe in the transformative power of deep learning and its applications for social impact. Our founders:
Mikela was recognised as one of the top female digital leaders by Campaign and received the “TopWomen50” award by WeAreTheCity in 2018. She was appointed to the World Economic Forum’s Global Future Council to provide thought leadership on the impact and governance of emerging technologies including AR, VR and AI. Mikela is passionate about gender equality, sustainable economic models and deep technology.
Dr. Marco Paladini, Co-founder & Senior Engineer - CV
Marco has a PhD in computer vision from Queen Mary University of London. He was previously a robotics researcher at Ocado and a senior computer vision engineer at Blippar. Marco loves robotics and automation. He has publications in CVPR, ECCV and other leading computer vision and robotics journals and conferences.
Nikola Sivacki Co-founder & Senior Engineer – DL
Nikola has a background in electrical engineering and has spent the last 15 years specialising in neural networks (deep learning) and statistical machine learning. He was the principal contributor in multiple commercial projects including high-noise OCR systems and landmark recognition under difficult out-of-distribution scenarios. He is passionate about applying the latest research to solve challenging problems.
Waste composition analysis to direct waste flow and for targeted education/incentive schemes with Egbert Taylor - https://egberttaylor.com/
Next generation robotics in sorting waste with ACI Chemicals - http://acientech.com/index_en
We will have a B2B licensing model whereby our clients pay for the use of our API within their systems (eg. smartphone, smart-bins, robots). The pricing is based on a combination of factors: number of users/ systems, number of calls/requests per hour, level of customisation.
We expect to be loss making for the first 3 year of the business and reach profitability in 2022.
We are passionate about solving industry challenges that can lead to positive social impact. This is the ideal platform for us!
- Business model
- Funding and revenue model
We are open to suggestions, our ideal partners include:
Data partners & potential future clients: Large MRFs (Material Recovery Facilities) and Waste Management companies - Viridor, Remondis, Veolia, Bollegraaf, Suez, Biffa, Pellenc
Public/Governmental bodies invested in modernising waste management
Robotics partners who can integrate our software.
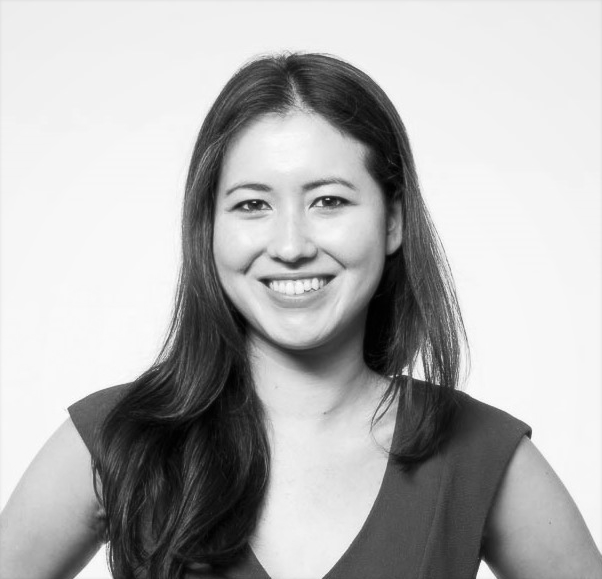
Head of Marketing & Operations