Disengage To Engage
- Nonprofit (may include universities)
Existing learning systems and related research typically focus their assessment on students’ active engagement with pre-defined effective strategies (e.g., help-seeking). However, there is a great potential for understanding and supporting students’ “strategic disengagement” (i.e., strategically choosing to not engage with a recommended behavior) during learning, often labeled as unproductive in current literature and technologies.
Through human-centered design with Priority Learners in Florida, we will develop an AI-empowered tutoring system that detects and adaptively supports middle-school students’ diverse “strategic disengagement” behaviors while learning algebra.
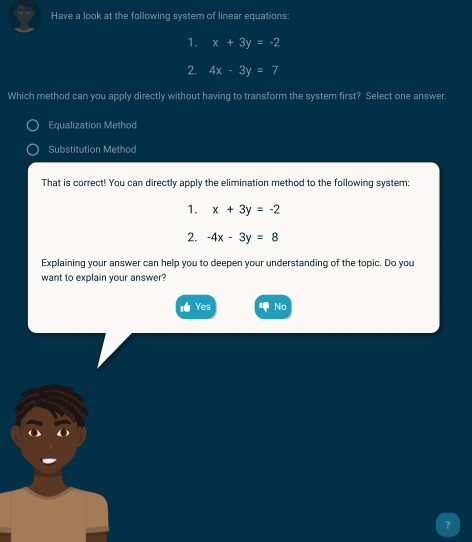
AI-based systems can provide opportunities for learners to engage or disengage with recommended actions (this is another platform of our own).
Specifically, we will do:
Phase 1 (Human-Centered Design of an AI-based System: 1-9th months): We will use human-centered, participatory approaches with Priority Learners in Florida to co-design an extended version of an Intelligent Tutoring System (ITS) for 6-7th graders to learn algebra. This system will facilitate engagement or strategic disengagement in metacognitive activities (e.g., self-explanation), recommended by a virtual agent. The agent will adaptively prompt students based on their changing knowledge states and engagement/disengagement patterns to promote strategic choice-making. This solution will leverage two existing, classroom-tested adaptive systems of our own that have served over 500 3rd-8th graders in the US and Germany where students could make strategic choices on using visual aids while learning math. In designing the new system, we will conduct participatory design sessions with Priority Learners to refine elements such as the recommendation algorithm, design of the agent, scaffolding messages, and interface design.
Throughout this phase, we will conduct iterative lab-based testing and collect multimodal data to assess Priority Learners’ (middle schoolers) engagement/disengagement behaviors. We will use the open-source Godot Engine (https://godotengine.org/) for implementation, incorporating best practices from our existing systems (one of which is built with Godot). By the end of this phase, the ITS will be ready for a classroom pilot, guiding students in making choices for strategic engagement/disengagement while learning algebra.
Phase 2 (Classroom Pilot: 10-18th months): We will conduct several pilot studies in US Florida classrooms with about 100-300 students. We will implement the software as a downloadable application for school devices. We will have consultation sessions with school teachers and administrators to tailor our solution (e.g., integration into their curriculum plan and technology). In each pilot study, we will measure students’ algebra learning (pre-post tests), capture strategic (dis-)engagement behaviors via multimodal data, and collect observational data. These data will be analyzed formatively such that the following pilot study evaluates improved algorithms and designs.
By the end of the project, our team will have developed a co-designed, iteratively-enhanced, pilot-tested AI-based ITS that detects and promotes students’ strategic disengagement behaviors while learning algebra step-by-step. This proposal is well-aligned with the Challenge as it overcomes limitations and biases in traditional assessment practices and provides a meaningful approach that addresses learners’ diverse characteristics and nuanced behaviors when learning with an AI system in the classroom.
In this project, we will conduct human-centered, participatory design with Priority Learners to create a solution that reflects their needs, backgrounds, and desires.
Our work will be focused on schools in Florida (Dr. Dever will be at the University of Florida in Gainesville, FL). In recent reports by the National Assessment for Educational Progress, Florida’s middle-school students have shown consistently low performance in science, reading, and mathematics since 1990, demonstrating significantly lower or at-average scores than the National public. In addition to this academic disparity in Florida students, the 2020-2023 US Census Bureau has reported that 29% of the population is considered poverty-level with a total of 48.8% identifying as races other than White only, in which 21.9% are Black or African American alone, 12.1% Hispanic or Latino, and 8.4% as two or more races.
Our solution aims to directly prioritize the Florida population to improve learning outcomes, achievement, and the lives of middle-school Priority Learners, providing comparable solutions for other states of similar demographics. To achieve this, our solution uniquely provides an AI-based technology that reflects students’ diverse backgrounds, contexts, and needs in its design that other popular technologies might not offer. Further, we aim to build a sustainable Researcher-Practitioner Ecosystem with education stakeholders (e.g., through having Student Researchers as the lead for the co-design process) so that our solution will not just be a one-time application but rather become the first step towards achieving a lasting impact on diverse students’ academic achievement.
Specifically, we will:
Empower Priority Learners by giving an active role in the Team as Student Researchers: in Phase 1, we will recruit a few high-school Priority Learners as “Student Researchers.” These Student Researchers will participate in and partly lead the design-research process under the guidance of the Team (e.g., they will conduct design sessions with other middle-school Priority Learners in Florida). By transferring the designer role to these Student Researchers, we aim to provide opportunities for Priority Learners to experience research and improve their self-esteem. Also, by letting them lead co-design work, we will create a safe environment for participating Priority Learners to share ideas. This inclusive strategy will help achieve the human-centered design of the solution that reflects their unique backgrounds, needs, and desires that otherwise may have been overlooked.
- Involve teachers during data analysis and interpretation: Through this work, we aim to create a “Researcher-Practitioner Ecosystem” in which teachers, learners, and researchers jointly and sustainably work together to advance research and practice. In classroom pilots, we will actively invite teachers who have experience teaching Priority Learners in the analysis and interpretation of the data to get their insights. For instance, these teachers can help inform if certain behaviors (e.g., long pause, or “silence”) by the learner are strategic or non-strategic. Such contextual knowledge from teachers will help us develop an authentic solution tailored to classrooms with Priority Learners.
Dr. Tomohiro Nagashima is an Assistant Professor in the Department of Computer Science at Saarland University, Germany. He has conducted human-centered, participatory research on AI-based tutoring systems in STEM with over 800 K-12 teachers and students with diverse backgrounds in the US, Japan, and Germany, providing sustainable access and opportunities for using advanced technology in the classroom. During the COVID-19 pandemic, his dedicated remote participatory research has resulted in a number of new partnerships with schools in rural, under-resourced areas in the US where access to technology and research participation opportunities is limited. With these experiences, Dr. Nagashima is suited to lead and achieve the proposed project, designing technology and its sustainable use with and for Priority Learners.
Dr. Man Su is a Postdoctoral Scholar in the Department of Computer Science at Saarland University, Germany. She has conducted design-based implementation research on integrating interactive technologies in K-16 learning and instruction in the US and Germany. Her works served Priority Learners, including Black, Hispanic/Latino/a/x/e, and Indigenous Americans who are underrepresented in most STEM fields. She has worked closely with local teachers, students, and researchers to co-develop frameworks, assessments, and software in STEM education. Dr. Su has extensive experience in designing and conducting professional development workshops on technology integration for teachers in the Northeastern and Southwestern US.
Dr. Daryn Dever is a Postdoctoral Scholar at the University of Central Florida in Orlando, FL USA and will be an Assistant Professor at the University of Florida starting July 2024. She has conducted research on game-based learning and intelligent tutoring systems within K-12 contexts. Dr. Dever has worked closely with local teachers to collect data on how these technologies can be used to provide individualized experiences to increase learning outcomes and interest within STEM domains. This has led to reciprocal outreach efforts with schools in which students are hosted at UCF to develop their interest in STEM topics and navigate future career opportunities in research. Dr. Dever is well-situated to continue networking within K-12, deploy developed technologies from this challenge within US schools, and provide academic and professional mentorship and opportunities for underrepresented students.
Additionally, we will recruit 2-3 “Student Researchers” to the Team from high schools in the community. Under supervision, they will conduct part of the data collection (e.g., co-design sessions with middle-school students) and participate in the data analysis, as well as in the design of the AI-based system. Providing Student Researchers agency to co-design our solution empowers Priority Learners, helping them gain experience in learning science research. Also, by letting Student Researchers who are themselves Priority Learners (high-school students) co-design the ITS, we can create a safe and friendly environment for participating Priority Learners (middle-school students) to share their voices freely, which will help us better understand their needs.
Further, we will recruit master’s students with backgrounds in Education, Computer Science, and Human-Computer Interaction in Germany and Florida to work on the extension of the ITS using Godot, co-design, and data analyses.
- Analyzing complex cognitive domains—such as creativity, collaboration, argumentation, inquiry, design, and self-regulation
- Grades 6-8 - ages 11-14
- Concept
We are at the Concept stage as we intend to co-design the technology with and for Priority Learners. However, we will base our solution on two existing adaptive tutoring systems, which have served over 500 middle schoolers in PA, CA, WA, and AZ in the USA and 30 elementary school students in Germany. We also have published and conducted analysis of existing data from these systems to develop a base model for strategic engagement and disengagement.
- Germany
- No, but we will if selected for this challenge
Our solution is innovative and novel in the following two main aspects: (1) a deep investigation of strategic disengagement, an important yet under-explored construct in AI-based learning systems, and (2) the active involvement of Student Researchers as a way to meaningfully design for and with Priority Learners, following human-centered approaches:
- (1) In the proposed solution, we will investigate and design for “strategic disengagement” in an AI-based learning system. Past research and existing solutions typically focus on learner “engagement” in their assessment and model building, and fail to capture important, strategic “disengagement” behaviors in the system (e.g., when learners choose NOT to engage with a recommended action by the system based on deep self-reflection). A deeper understanding, and practical application, of how learners engage and disengage in an AI-based learning system in our project will provide a blueprint for how future systems can incorporate strategic learner disengagement in better understanding and supporting student learning in a more personalized way. This new construct will also advance the scientific understanding of how students disengage to better engage during learning with technology.
- (2) Most traditional human-centered, participatory research methods for AI-based learning technology do not fully give agency to learners in the design process. In our proposed project, we will recruit high school Priority Learners as “Student Researchers,” who will be part of the research team. These Student Researchers will lead the design of an AI-based learning solution with other Priority Learners (middle-school students) in the community, following human-centered approaches. They will also join high-level discussions and participate in other research activities as main contributors to the team. Through these dedicated activities and mentorship, we aim to empower Student Researchers, providing an opportunity for them to experience research and design (such an opportunity would otherwise not be available to them). Further, we aim to deliver a safe and friendly environment for participating Priority Learners by giving an active role to Student Researchers as facilitators of co-design activities (i.e., Student Researchers will lead co-design with participating Priority Learners). This innovative model of stakeholder involvement will help us create a "Researcher-Practitioner Ecosystem" where practitioners and researchers can sustainably and effectively continue working together, providing access and opportunities for under-resourced communities to get involved in STEM learning and research.
With these innovative features, our solution will contribute a deep understanding of how we can effectively treat learner disengagement in AI-based learning systems. In doing so, we will uniquely have Priority Learners involved in the design/research process, creating the solution that is relevant for them.
Our solution focuses on developing an AI-empowered tutoring system for learning middle-school algebra. Specifically, we will build student models that capture each learner’s (patterns of) behaviors and estimate the level of skill mastery in a personalized way. These models not only detect and adapt their actions (e.g., giving recommendations through a virtual agent) based on students’ domain-level knowledge and skills in algebra but also get adapted to students’ strategic engagement/disengagement behaviors in the system. The student modeling approach has been commonly used in other major Intelligent Tutoring Systems (with which the researchers on the team have experience), having been successfully adopted in US classrooms.
While the kind of artificial intelligence employed in our solution does not fall under any distinct categories provided as examples (e.g., Machine Learning, Natural Language Processing), this modeling approach to achieving personalized support in a learning system is within the broad category of AI in Education (See the statement by the International Journal of Artificial Intelligence in Education, a leading journal in the area: https://link.springer.com/journal/40593/aims-and-scope). We will base our solution on some prior work on AI in Education, but the algorithm we will build will not rely on any third-party models. This algorithm will be implemented within the Godot Game Engine.
Regarding the frontend user interface of our solution, we will start the work based on the existing system we have built using the Godot Game Engine before (https://helenakilger.itch.io/multidivitutor) but will engage in iterative co-design with Priority Learners to extend it into a learning system for middle-school algebra.
The solution will be developed based on an existing tutoring system built with the Godot Engine (https://helenakilger.itch.io/multidivitutor, only in German at the moment), which has successfully been used with PreK-8 school classes (e.g., Kilger et al., 2024). As this existing tutor focuses on elementary math, we will co-design an extended version of the tutor that targets algebra learning, with some design principles informed by another existing tutoring system (Nagashima et al., 2023). This latter system has served over 500 middle-school students in the US (paper: https://tinyurl.com/Nagashima2023)
The Godot Game Engine provides flexible programming, allowing developers to freely design algorithms and define log data based on their needs. The developed software can be implemented and downloaded onto laptop or tablet devices for use and collection of data in the classroom context. To make sure that the technology works with our target group (6-8th grades), in Phase 2, we will have extensive discussions and consultations with local teachers and students, as well as school administrators so that the solution can be integrated into the school classroom context smoothly without any serious hurdles. Also, we will undergo iterative user testing to design software that is user-friendly for middle schoolers.
Finally, the research team is well equipped with skills to conduct school-based studies with ITSs. The team members have expertise and experience in conducting studies in US classrooms (Dever et al., 2023; Nagashima et al., 2023) and improving ways to make these school-based studies more meaningful for practitioners (Nagashima et al., 2024; Nagashima et al., 2021).
References:
Dever, D. A., Wiedbusch, M., Park, S., Llinas, A., Lester, J., & Azevedo, R. (2023). Assessing the complexity of game mechanic use during science learning. In Proceedings of the 12th Annual Games and Learning Alliance Conference (pp. 299-308). Dublin, Ireland. Cham: Springer Nature Switzerland. [link]
Kilger, H., Su, M., & Nagashima, T. (2024). Guiding children’s choice making in an Intelligent Tutoring System. In Proceedings of the Learning, Design and Technology (LDT ’24), Delft, Netherlands. ACM. [link]
Nagashima, T., Bonaventura, K., & Su, M. (2024). Parallel design: Achieving both researchers’ and practitioners’ goals in the design of an interactive learning system for mathematics. In Proceedings of the Learning, Design and Technology (LDT ’24), Delft, Netherlands. ACM. [link]
Nagashima, T., Zheng, B., Tseng, S., Ling, E., & Aleven, V. (2023). Promoting students’ self-regulated choices in learning with visual representations in intelligent tutoring software. In Proceedings of the Annual Meeting for the International Society of the Learning Sciences (ISLS2023), Montreal, Canada. [link]
Nagashima, T., Yadav, G., & Aleven, V. (2021). A framework to guide technology-based educational studies in the evolving classroom environment. In Proceedings of the Sixteenth European Conference on Technology Enhanced Learning (EC-TEL2021) [link]
Ensuring equity and combating bias is the top priority in our proposed work. Specifically, when we build intelligent models, we will aim to inclusively understand and address the varied backgrounds and needs of Priority Learners in Florida, US.
Our project employs a human-centered design approach to develop an AI-empowered adaptive tutoring system for middle-school students learning algebra. This system is unique in a way that treats students’ diverse “strategic disengagement” behaviors as resources to detect and understand why they choose not to engage with recommended behaviors in order to achieve better learning outcomes. Such disengagement is often labeled unproductive in current educational technologies and literature.
In Phase 1, we will collaborate with Priority Learners through participatory design sessions to co-design this system, ensuring it reflects their unique needs and contexts. This involves close interaction with high-school students who will take the role as Student Researchers, middle-school students who will function as Co-designers. This human-centered design approach will offer vital insights that enrich the development process and ensure the system is culturally and contextually relevant to Priority Learners.
To combat algorithmic bias, our development process features:
Iterative testing: we will conduct lab-based testing to refine the AI algorithms based on real student interactions and feedback.
Inclusion of diverse datasets: we will collect and analyze (multimodal) data with diverse student populations in Florida to ensure our training data is representative of the Priority Learners population to prevent bias and discrimination in the initial modeling process.
Sustained consultation with educators: we will consult with teachers who have firsthand experience with Priority Learners to inform our contextual understanding and data interpretation.
Transparent algorithms: we will make the algorithms used by our AI system visible to educators and researchers, allowing for checks that prevent discriminatory practices.
In Phase 2, we will pilot our system with Priority Learners from diverse classroom settings across Florida. We will closely monitor the data collection process to ensure the inclusiveness and fairness of the implementation. Insights gained from these pilot studies will inform continuous improvement, safeguarding the AI system adequately supports all learners without bias and prejudice.
In sum, our solution is deeply entwined with rigorous equity-focused practices that place Priority Learners’ needs at the center, involving continuous monitoring and sustained community engagement to prevent and address bias. We are confident that our efforts will ensure the AI implementation is accessible, equitable, and effectively adaptive for all learners.
3 full-time staff (Drs. Nagashima, Su, and Dever)
2-3 Student Researchers in Florida/USA (part-time; school students)
2-3 Master's students in Florida/USA and Saarland/Germany (student research assistants)
As detailed in other sections, we plan to conduct pilot use in Florida classrooms within one year of selection. To achieve this goal, we will do the following:
1) Build partnerships with middle schools in Florida early in the process: from the beginning of the funding term, we will reach out and start talking with schools in Florida so that we can plan pilot studies well in advance. This consultation process will also help us find and mitigate any geographic, cultural, organizational, and/or instructional issues that might prevent classroom use early in the process. Dr. Dever (based in Florida), together with Student Researchers, will lead this effort. Team members in Germany will also visit Florida schools several times during the design phase to build partnerships with schools.
2) Iterative lab-based testing during the design process: we will iteratively test the solution early in the design phase to ensure that the final solution is well suited for the classroom context. Early testing will mainly be conducted in the lab both in the US/Florida and Germany/Saarland.
3) Student Researchers as a liaison between schools and researchers: we will recruit a few high-school Priority Learners as Student Researchers in the research team. These Student Researchers will facilitate communications between high/middle schools and the research team as liaisons, making it possible to have frequent and open conversations between the two teams. Such open conversations will help us identify any potential issues and opportunities for the classroom pilot.
We will make our solution (ITS) publicly available at no cost through various channels, including platforms for downloading tablet apps (e.g., AppStore) and browser-based web applications to cover different needs and hardware situations in schools (e.g., temporary use). They will accompany instructions for teachers and learners on how to effectively use the software in the classroom. We will also actively promote the software at practitioner-oriented events (e.g., the US Department of Education’s EdGames Expo: https://ies.ed.gov/sbir/EdGamesExpo.asp).
We will make our research findings (e.g., scientific papers) accessible whenever possible, and also provide a more user-friendly approach (e.g., blog posts, short videos) to make findings accessible to a wider range of audience.
Finally, we plan to make design materials used in the project (e.g., worksheets for algebra problems) publicly available under a Creative Commons Attribution license (CC BY) as Open Educational Resources (OER) for education stakeholders with limited internet access. Dr. Nagashima has 10 years of experience as an OER advocate, instructional designer, and researcher and will lead these dissemination efforts.
The Solve Challenge provides an important funding opportunity for collaborating international early-career researchers (like us) to propose innovative work. All team members with doctorates were trained in the US, but based on the international experiences we have gained, we came to think that it is important for us to contribute our diverse perspectives to the US PreK-8 education (other major funding agencies would not allow such international innovative work). This funding will uniquely help us overcome this challenge that we have faced in conducting such international collaborative work to bring an impact in the classroom where diverse students learn.
- Product / Service Distribution (e.g. collecting/using data, measuring impact)
- Public Relations (e.g. branding/marketing strategy, social and global media)
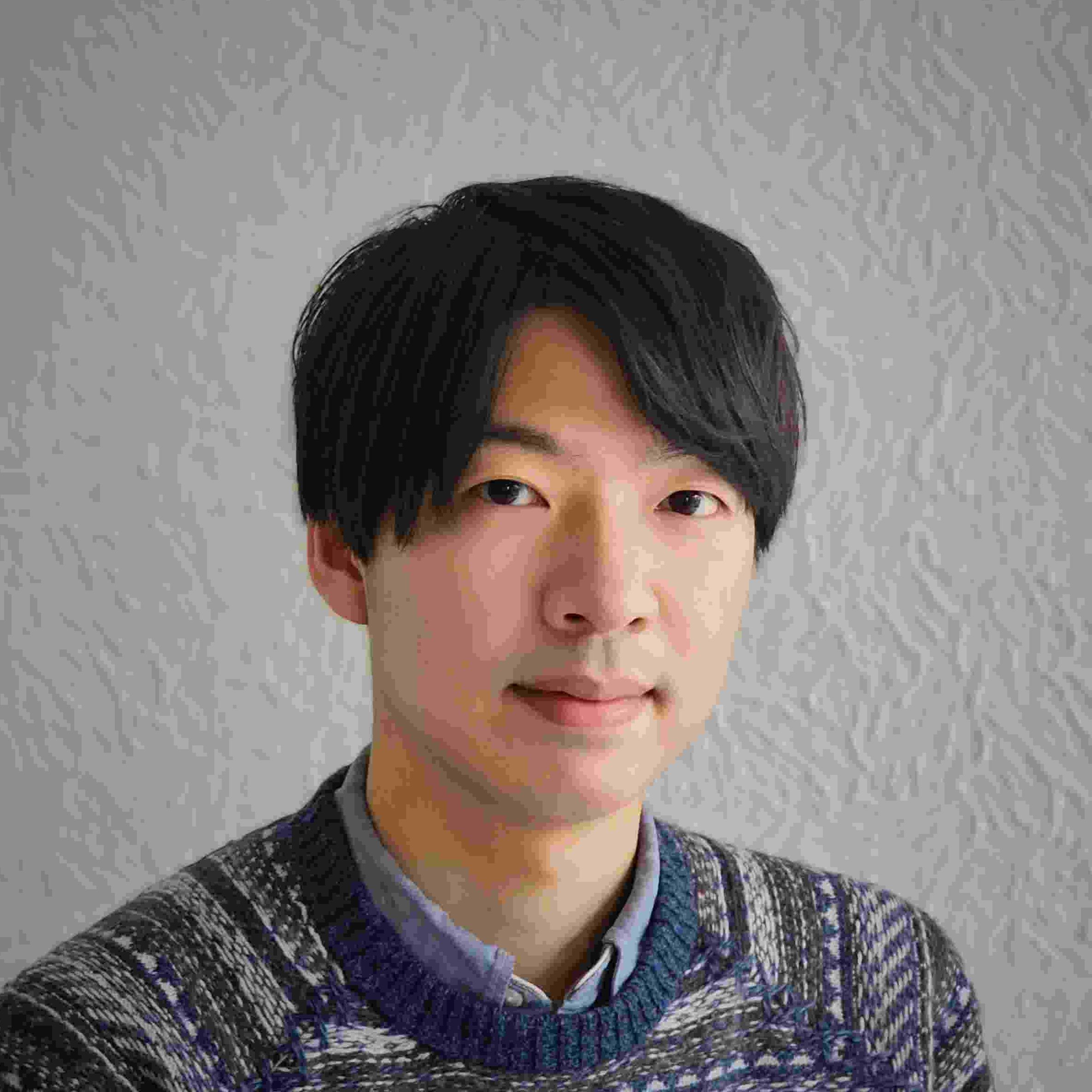
Assistant Professor
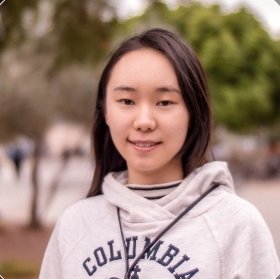
Postdoc Researcher
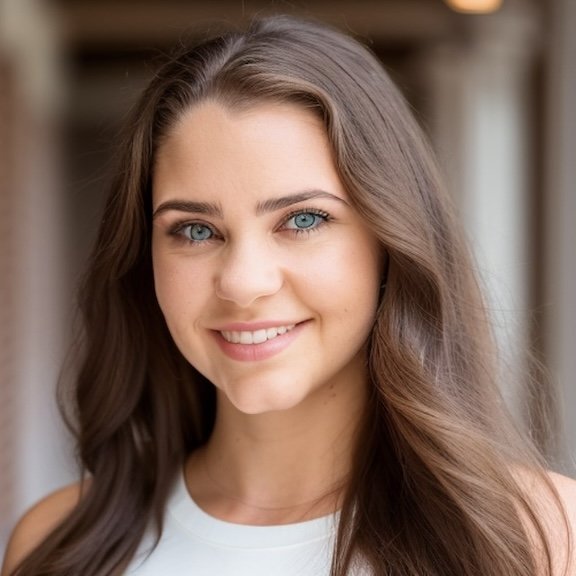
Postdoctoral Researcher