AI-Powered Oral Reading Fluency (ORF)
- India
- Nonprofit
Foundational Literacy and Numeracy (FLN) are indispensable skills, contributing significantly to a child's cognitive development and laying the groundwork for future achievements. It encompasses the attainment of fundamental skills, concepts, knowledge, and attitudes that assist children in navigating life. In Literacy, children initially ‘learn to read, and this lays the groundwork for them to ‘read to learn’. Considering the transformative phase a child undergoes during primary education, investing in foundational learning can unlock opportunities for building their career and life.
Recognizing the importance of foundational literacy and numeracy, the Government of India introduced the National Initiative for Proficiency in Reading with Understanding and Numeracy (NIPUN) Bharat policy. It aims to achieve a Global Minimum Proficiency of Oral Reading Fluency (with comprehension) of between 30-55 wpm all-encompassingfor 20 Indian languages by the end of Grade 3 by 2026–27. The National Education Policy 2020 outlines that oral reading fluency (ORF) is a key pillar for the FLN mission.
The Problem
Despite the acknowledged importance of quality foundational education, the situation is quite alarming. In India, according to the latest estimates of the Annual Status of Education Report (ASER),only 42.8% of Grade 5 students in India can read a Grade 2 level text. Based on the Foundational Learning Study (FLS) conducted by the NCERT in 2022, in the oral English language, only 21% of children across the country meet the Global Minimum Proficiency Levels; in Hindi, only 23% of children meet the Global Minimum Levels, so there's a lot of ground to be covered.
The NIPUN Bharat policy recommends that teachers should assess students on their reading fluency to track their progress regularly.
There are several reasons for low reading fluency. The high pupil-teacher ratio (PTR) in schools exacerbates this issue, widening the gap between the support the students need and the institutional support offered by the school system.
Challenges with the manual assessment process
Currently, reading assessments are conducted manually by teachers, which takes up to 20 minutes/student on average. Key reading fluency indicators such as reading speed (CWPM-Correct words read per minute) and correct words spoken are difficult to capture and verify for teachers and administrators when taken manually. Due to the lack of uniformity in the metrics of assessment, it can't be used to measure progress from one assessment to another.
There is no common repository of learning data for a single child, scaled up to school, district, state level, etc. Data might be scattered, might be manually recorded or simply may not be recorded for each child, which makes it difficult to track the progress of an individual student. Making it difficult to drive targeted pedagogical interventions.
Since assessments don't happen frequently enough and learning data is not organized, teachers are ill-equipped to implement personalized remediation. The time taken in assessment cuts down on the time for remediation and regular monitoring support for teachers in high PTR classrooms and schools. Providing personalized feedback and remediation to students and teachers becomes difficult to conduct at scale.
ORF, an AI-powered tool is for governments, teachers, and even students to aid in improving Oral Reading Fluency by conducting reliable, verifiable, scalable, and easy-to-use reading assessments. ORF aims to serve populations underserved by mainstream technology, including public schools, in low-resource settings.
Modules
Built and deployed, used by 120K teachers: This module automates the assessment of ORF and shows as outputs the CPWM (Correct Words Read Per Minute), Missed Words, Incorrect Words, Correct Words, and Extra Words with high accuracy (95% precision for recognizing correct words read) and with high efficiency (<10 sec taken to generate a report with 10K simultaneous assessments). The tool has been built for testing in three languages so far (Gujarati, Hindi, and English) and produces accurate results even in noisy environments. We use noise cancellation tools to address the challenge of voice overlap, which is likely to happen in schools and classrooms where this tool is used. The assessments are geotagged to generate verified and unbiased output.
The first version developed and piloted: Currently, a student-wise learning report is shown to the teacher on her phone shortly after the child provides a voice recording. In addition to this, we are building dashboards that aggregate learning data at the school, block, district, and State-level so that the State Education Ministry can plan granular policy actions. This will lead to informed decisions and educational strategies with Block Resource Centers (BRCs) based on the insights shown on the dashboard.
Prototyped: The tool is today able to capture extremely granular learning data, including CWPM, missed words, incorrect words, correct words, extra words, and pause time. We are working on using the data to create a personalized remediation tool that suggests specific reading practice and/or levelled texts to every child, personalized to his/her performance data from the ORF assessment. For example, the tool might present some phonological awareness practice to the child or specific high-frequency word reading practice, depending on the insights gleaned from the recording of her reading. This tool is being developed in different modes so that it may be used in low-resource settings as a teacher planning tool and also as a student-facing self-practice tool.
Technology:
The technology uses Deep Learning at its core. Audio is recorded and processed. During processing, denoising is an important step. The denoised recording is passed through multiple Automatic Speech Recognition(ASR) models which output transcripts with timestamps. Multiple outputs are used as an ensemble in the Combining Module to improve overall performance. Each transcript is compared with the target paragraph, and the best alignment is obtained. Finally, using the best alignment, metrics such as hits (correct matches), substitutions (incorrect matches), insertions (new spoken words), and deletions (missed words) are computed and reported.
Deployment:
Our ORF technology has been built in a modular manner so that it can be deployed both as a standalone smartphone app. It can be integrated into existing Partner/ government tech infrastructure. We are working on developing an offline version that can be used in low-network environments.
The ORF tool aims to serve populations currently underserved by mainstream technology, including schools under the ambit of public education, to help students achieve Global Minimum Proficiency Levels in oral reading fluency. ORS works as a bridge between the current school ecosystem and students by providing accurate data. A correct diagnosis is the first step toward improvement. ORF supports the following stakeholders to work towards positive improvements in each student through accurate and real-time diagnosis of oral reading fluency.
Student (Primary): Students with alarming levels of low reading proficiency in rural and semi-urban India struggle with below-average standard-based learning outcomes. Low reading proficiency has a longer-term effect on a student's success. They lack access to quality resources and personalized support tailored to their learning needs. This leads to students never moving from learn-to-read to read-to-learn, which results in them not reaching their full potential and their education being incomplete. Our ORF tool aims to improve key learning metrics such as CWPM, Vocabulary and Comprehension for each child in their language of instruction (L1) as well as English (L2) so that they may become confident and independent learners for life
Teacher (Primary): In schools with high Pupil-Teacher Ratio (PTR) and 800,000 single-teacher schools, teachers struggle to implement the assess-plan-teach cycle for each student in their class in a personalized manner due to limited support and time constraints. Teachers end up teaching standardized content even though students in their class have very different learning levels. The ORF tool Equip teachers with 1. Automated assessments 2. Student specific learning reports 3. Leveled remediation material so that they are able to implement personalized teaching effectively. ORF generates personalized reports for teachers and administrators, offering insights into individual student performance and progress within their class or jurisdiction. This tool would especially be game-changing in extremely backward areas where teachers themselves are not able readers, especially in English (L2)
School: Due to the lack of set metrics for reading assessment, most schools are dependent on teacher competence in language to assess reading, leading to incorrect diagnosis and inconsistent evaluation practice. As ORF tools get integrated into the school system as a foundational learning and numbers platform, it makes sure assessment and support in remediation to improve reading competency. This allows schools to focus on improvement in each student learning journey
Administration: Data provided from ORF assessments can provide valuable input in education policy formation and changes at the district or state level. With the help of data-driven insights, ORF aims to influence policy and education strategies. Vidya Samiksha Kendra VSK at the state level utilizes ORF data to strategize school visits and interventions, leading to informed decision-making and education strategies within blocks and clusters with block resource centers (BRCs)
About Wadhwani AI Wadhwani AI is an independent, non-profit, applied AI institute developing and deploying AI solutions with a mission to create social impact at scale across the global south. Inaugurated by the Prime Minister of India in 2018, we work closely with governments and global partners to solve complex problems affecting developing countries, where AI can play a transformative role. Our 200+ strong multi-disciplinary team is working on 30+ AI solutions across domains such as education, healthcare, and agriculture. Our operations are based in India and we have a US-based 501c3.
Our team consists of experts from diverse backgrounds, including those in education, technology, research, and community engagement. This multidisciplinary approach allows us to bring together diverse perspectives and skill sets to tackle complex challenges comprehensively
We have established partnerships with government agencies, educational institutions, NGOs, and other stakeholders working in the education sector. These partnerships enable us to collaborate closely with local communities and stakeholders to understand their needs and co-design solutions that are contextually relevant and effective.
Education Practice at Wadhwani AI The Education team at Wadhwani is composed of members from different states and towns in India, including Tripura, Bihar, Uttar Pradesh, Karnataka and Gujarat, under the leadership of Manoj Karnik. Manoj chose to spend two years teaching in a Municipal School as a Teach For India fellow and then moved into a Strategic Partnership role at Akanksha Foundation - an NGO that runs multiple high-performing schools for underprivileged children in a PPP format. He thus brings with him more than 10 years of experience working very closely with the kind of schools that we hope to equip with our ORF technology. He bolstered his on-ground experience with a MPP and MBA from the National University of Singapore and especially helps the team navigate the nuances of working with regulatory bodies and governments.
Within the team, we have access to a diverse set of skills, including Machine Learning, Engineering, Design, Instructional Pedagogy, Data Analysis, Training, Deployment and Monitoring & Evaluation. Design-led research is deeply embedded into the team’s way of working, with frequent field visits organized at every stage of the product development process. Through our network of partner organizations, we have so far been able to visit, pilot the solution and incorporate insights from rural schools in Gujarat, Bihar, Andhra Pradesh and Maharashtra. This diversity of experience has ensured that we tailor the solution to fit the needs of different states while also identifying the common problems that exist across the length and breadth of the country.
To ensure that we are always in close proximity to the schools and children we want to serve, some members of our team are stationed full-time within the State Vidya Samiksha Kendra's (School Performance Monitoring Centers), such as the one in Gujarat. This enables us to get real-time feedback from our users (teachers) and our beneficiaries (students), while also giving us a deployment pipeline into the State.
- Ensure that all children are learning in good educational environments, particularly those affected by poverty or displacement.
- 4. Quality Education
- 17. Partnerships for the Goals
- Pilot
As explained above our solution is split into three modules: 1. Automated Assessments 2. Data Dashboards 3. Personalized Remediation. While Assessments and Data Dashboards have been piloted in a few states, the Personalized Remediation module is currently in Prototype stage, which is why we have selected Pilot in the previous answer. More details follow.
A. Automated ORF Assessment (Pilot)
- Large Deployment in Gujarat: The first version of the solution with the Assessment module has been implemented in Gujarat in partnership with the State Government. The solution is integrated with the state’s ed-tech platform, G-Shala, along with private ed-tech partner SwiftChat, both of which are widely used by teachers across the state as educational content applications. With this state wide deployment in Gujarat, we have conducted more than 3 million assessments for 2.6 million students across 22K schools with the help of about 120K teachers. Going forward, ORF assessments will be integrated with the programmatic wrapper of Periodic Assessment Tests (PAT) in Gujarat with the new academic session.
- Small Pilots in Maharashtra and Bihar:
- A. In partnership with an NGO in Maharashtra: pilot assessments were conducted with 240 students, with potential for broader implementation.
- B. In partnership with the CSR arm of a corporation in Bihar: pilot assessments were conducted with 700 students, and conversations are on for state-wide deployment.
B. Data Dashboard (Pilot)
A dashboard has been developed and is in use in Gujarat in their Vidya Samiksha Kendra (School Performance Monitoring Centre). The dashboard shows key data points about the usage of the ORF tool split by district, school and grade (so that administrators might motivate low usage districts to ramp up their usage) and key learning metrics (such as CWPM) split by Grade so that targeted pedagogical initiatives might be implemented. We are now working to incorporate more useful and granular metrics on this dashboard.
C. Personalized Remediation (Prototype)
This module is currently in the prototyping stage, and we are on track to pilot it in Gujarat state at the beginning of the new academic year from June to August 2024.
As we complete our pilot phase, we are now looking forward to the growth phase to implement ORF across India in more than 15 Indian languages and multiple states. Solve’s recognition, positioning, value, team, partners, and network will put us at a place where we can navigate through this phase with better clarity. While fundraising is a part of our goal, it is not the only reason for our interest in applying to Solve. Here is how we feel Solve can help us:
Financial Support: Securing funding is critical to scaling ORF and reaching communities across India. Machine learning-based solutions require investment in technical infrastructure and have recurring computational costs. The compute and infra cost skyrocket when used on a large scale. Solve's platform can help connect with potential CSR, donors, and philanthropic organizations inclined towards supporting innovative Tec solutions for underserved communities. Through leveraging Solve’s network, we can gain access to resources necessary for scaling our solution, invest in technology infrastructure, and sustainably increase our impact through scale and innovation.
Technical Expertise: The audio recordings were transcribed by us with open-sourced Indian language ASR models up till now. As we expand to more Indian languages, we are likely to find gaps with the existing ASR models and will need expertise in Deep Learning to help us tweak such models to serve our needs. Similarly, for the remediation module, which is still in the prototype stage, we would require help in creative brainstorming to identify the right points at which both ML and Engg can be used to make the remediation truly personalized. Solve would be able to provide access to the best minds in Deep Learning and Engineering globally to help us meet these challenges.
Monitoring & Evaluation: For the growth of ORF, we must understand its impact and rectify it according to response and understanding that will be enhanced through effective monitoring and evaluation. Solve can offer knowledge on how to develop strong monitoring & evaluation frameworks in order to measure and improve our progress and also show stakeholders the impact made by ORF.
Network Expansion: Strategic partnerships are essential in scaling up our ORF and enhancing its range of operations. Through Solve’s networking platform, that brings together innovators with similar interests, supporters, government entities or departments, and relevant organizations, we can create new alliances and explore how we can work together with other initiatives, thus making our efforts have a bigger impact
- Financial (e.g. accounting practices, pitching to investors)
- Product / Service Distribution (e.g. delivery, logistics, expanding client base)
- Technology (e.g. software or hardware, web development/design)
So far, technology led solutions for testing Oral Reading Fluency in India have only focused on making data collection digital, but have not considered the errors in human judgment that persist when the assessment is administered by a teacher or an enumerator. The assessor needs to be proficient in the language themselves to identify correctly or incorrectly read words, and they also need to be very careful with recording the time to calculate CWPM accurately. Our AI driven solution recognizes the limitations of current solutions and is designed to automate the assessment of oral reading skills completely by leveraging ASR models to identify correct words, missed words, and incorrect words and generate a CWPM metric. The solution thus completely reduces the administrative and cognitive load on the teacher or the enumerator, recognizing that the main role of the teacher is to provide the encouragement and motivation for the children to complete the assessment task. Using ASR models also opens up various possibilities to do extremely granular analysis, for eg by aggregating word-level information across multiple students at school, district, or state levels, we are able to estimate the difficulty of each word at a granular location and grade level, and also create a learning path (from easy, medium, to difficult words) for students to build their vocabulary depending on their grade and location. This kind of analysis is not possible without using AI as a core part of the solution. By automating assessments and recommending personalized remediation, we want teachers to focus on actually scaffolding and motivating the children instead of spending the majority of their time in conducting the assessments and compiling data.
Specifically:
Our solution can create near real-time report for each assessment
Our solution can be built in any Indian language, opening access to states which do not use English or Hindi as their medium of instruction
Our solution is modularized and can be integrated with any application or website.
Our solution has the capability to record granular data which can be used to produce child specific remediation recommendations. For example, pause time can be used to analyze whether the child understands punctuation or not.
Our solution can be used as a teacher-assisted tool or as a direct student facing tool so that learning can continue at home on any low-cost smartphone that is accessible to the child.
Our solution has been designed to work in noisy environments and for low network settings.
The ORF, AI-powered tool aimed at improving students reading fluency is designed to improve the fluency and comprehension of children’s reading. It is for students from class 2nd to 8th, delivering timely and accurate data to teachers. We are working on building ORF to help teachers remediate students and improve their reading skills. This implies that there will be some impactful effects of using this ORF tool
Real-time assessment is an essential feature of our tool. Once the students read aloud, our ORF (Oral Reading Fluency) tool analyzes their voice and provides accurate results on their CWPM (correct words per minute), missed words, correct words, and incorrect words immediately. This data allows teachers to have a wholesome view of individual students ' reading competency. Teachers can work on a customized plan based on the student's level.
Personalized feedback and remediation: Based on the real-time results, teachers can work on personalized support for each student and assist them in improving reading competency at their own pace.
Teacher Support: ORF provides teachers with an immediate diagnosis of each student. Which helps them to be more strategic about their teaching process. Re-assessing the results helps show the effectiveness of the teaching process for increasing reading competency and catering to the diverse needs of students. Implementing this technology helps improve the efficacy of classroom teaching plans and pedagogy.
Broader Educational Impact: ORF works towards enhancing students' reading ability with a direct impact on their learning outcomes. Moving from “learn to read to read” to “read to learn," every student can unlock most of its potential in academics and life ahead.
Our driving motivation is to significantly improve foundational literacy outcomes among primary school students in underserved communities, leading to enhanced learning outcomes and increased opportunities for success in education and beyond.
Goals for the next two years
- We aim to conduct 100 million assessments in public schools across 15 Indian languages and add AI based remediation features to improve the impact of assessments on learning outcomes.
- We want to play a significant role in the Government of India’s target to achieve a Global Minimum Proficiency of 35–50 wpm for oral reading in Grade 3 by 2026–27 in these regions
- We want to be the tool of choice for national assessments, Periodic Assessment Tests (PATs), and semester tests so that we are able to create one source of learning data for each child for foundational literacy
Use Case
We are expanding on this answer for the core use case that has been built and deployed at a sufficient scale. First we ask the student to read/record a target paragraph. Next, we automatically transcribe the audio and provide a list of correct and incorrect words, along with an overall score. Currently we have developed this solution for Gujarati, English and Hindi. Summary of the Technical Approach
- 1. The audio recording provided by the student is normalised and denoised using the Facebook denoiser
- 2. ASR models are used to get transcripts and character level timestamps. We currently use an ensemble model using two or more publicly available ASR models for Indian languages. A lot of effort has gone into fine tuning these publicly available models using large data sets of annotated recordings.
- 3. We perform alignment between the target paragraph and the output transcript. Multiple alignment inputs are combined to get the final list of hits (correctly read words), substitutions (misread words), insertions (extra spoken words) and deletions (missed words).
- Finally, scores such as Correct Words Read Per Minute (CWPM) are computed.
Model Performance
On our current Gujarati model, we have fine-tuned the ensemble model and alignment to achieve a low Character Error Rate of 8.24% and Word Error Rate of 27.38%. The model has a 95% precision rate of recognizing Hits (Correctly read words) and 88% precision rate of recognizing Deletions (Missed Words).
- A new technology
As the ORF is a new technology. To begin where we did ground research to understand the need of the government and teachers and worked on making the product that acts as a catalyst between the different stakeholder in the school ecosystem.
ORF Evaluation: A total of 295 audio recordings and 40,851 words were assessed by the teachers. Table 1 provides a comparison of words assessed by the AI model and gold standard teachers. The accuracy was calculated by summing up the diagonal value and dividing it by the total number of words. The overall accuracy of the AI model was 87.4%. The sensitivity of the model was 82.4% for identifying correctly pronounced words and 99.1% for incorrectly pronounced words. The positive predictive value (precision) was 99.7% for identifying correctly pronounced words, and 65.5% for incorrectly pronounced words. Table 2 provides deep dive into the different metrics to assess the performance of the AI model.
Table 1: Comparison of the words assessed by the AI model and gold standard teachers.
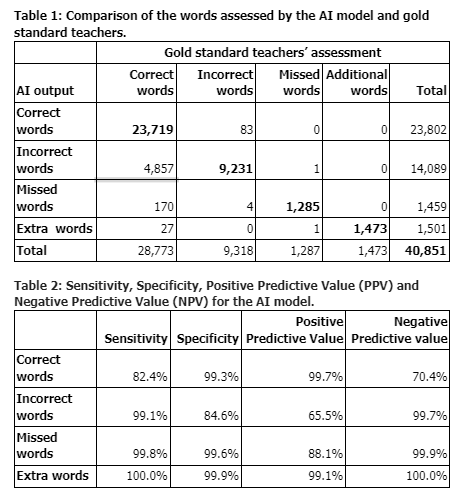
2. Benchmarking: We compare the ASR technology in our Oral Reading Fluency solution with openly or commercially available alternatives. Specifically, we compare our model against.
Google speech API V1, Google speech API V2, AWS Transcribe API, Microsoft Azure API, and Whisper V3 (OpenAI). Both performance and cost are compared. We also state the achievements and limitations of each system. Our model is ~50 times cheaper than the cheapest speech API. Furthermore, the server or inference costs of our model deployed on the phone can be thought to be 0 $. All our models are trained on non-overlapping data. In end-to-end model performance on the overlapping test set, we replace our ASR model with the target API to compute metrics. Our model comfortably outperforms the best Google and AWS APIs on all metrics. Additional pros and cons of the commercial models and our system are listed below.
Commercial Models/API: Coverage of 100s of languages. It can lead to quick prototyping. Easy to scale with low latency.
Our system: Better in performance as compared to any other commercially available APIs. This is expected, as we can fine-tune and adapt our models to similar data. However, some annotations will be required for fine-tuning. More than 50x cheaper for inference than any other commercial API.
3. User Feedback: We continuously engage with the direct user for feedback. We are in direct touch with teachers using ORF on the ground. This helps us understand the effectiveness, ease and impact on the ground. Multiple schools are using ORF continuously to measure the change. There are schools in Gujrat where the teachers have printed out the results to work on remediation.
4. External Evaluation: Our model has been tested against annotated data and has a 27.38% Word Error Rate and 8.24% Character Error Rate. Aside from internal evaluation, we are in process of getting a third party to do an impact evaluation of the pilot going on in Gujarat
- Artificial Intelligence / Machine Learning
- India
- India
All our team members are full-time staff at Wadhwani AI.
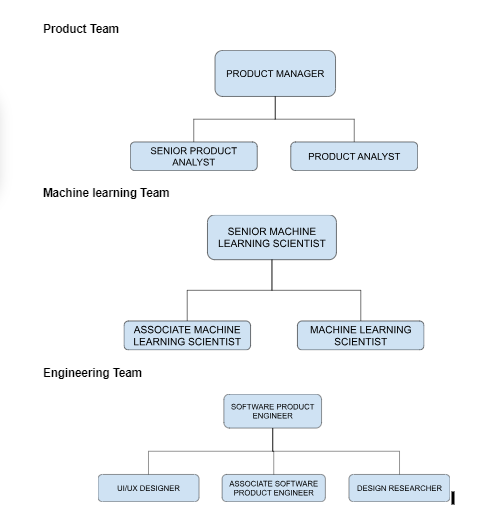
Senior-Product Manager
Manoj Karnik
50%
Senior-Product Analyst
Kartavya Purohit
50%
Associate-Product Analyst
Samarpita Debnath
70%
Senior ML Scientist
Makarand Tapaswi
10%
Associate ML Scientist
Jatin Agrawal
100%
Associate ML Scientist
Ayush Deva
50%
Associate ML Scientist
Vivek Pandey
30%
Software Product Engineer
Shubham Rastogi
60%
Associate Software Product Engineer
Prabhsharan Singh
80%
Associate Software Product Engineer
Dev Kumar
70%
Associate Designer Researcher
Daksha Dixit
60%
Design Intern
Nehal Mehta
100%
Strategic Partnerships
Janice Masih
100%
Partnerships and Fundraising
Yamini
100%
Senior MEL
Malay Shah
5%
Junior MEL
Dr Prachi
25%
We have been working on this solution for about 15 months. We have deployed ORF in Gujrat since last 5 month.
Navigating work and well-being, diversity, and inclusion are woven into our organizational fabric. With a distinct focus on women in the workplace, we pioneer initiatives prioritizing well-being and career continuity.
For female staff, we have introduced a groundbreaking no-questions-asked work-from-home day monthly, recognizing unique needs during menstrual cycles. Expecting mothers benefit from a supportive transition back to work with a 3-month work-from-home option and full-year performance pay.
Our commitment extends beyond maternity, embracing those returning from sabbaticals or career breaks. We offer childcare benefits to all employees, wherein they can get daycare expenses reimbursed for two kids up to 6 years of age. Our culture fosters care, learning, and collaboration, allowing seamless integration and growth. As an added incentive, refer a female candidate and, upon selection, receive an additional referral bonus. Work-life harmony is our priority, as evidenced by unlimited time off. WIAI is a workplace that thrives on diversity, inclusion, and genuine care.
As an NGO focused on AI/ML, our business model is dependent on grants and funding from philanthropic organizations, government agencies, and other stakeholders. Stakeholders involved are committed to investing in advanced technology in the social sector to accelerate impact in underserved communities. Our key customers and beneficiaries include students, teachers, schools, and educational policymakers in these communities.
Products and Services:
We implement ORF in Partnerships with Government Stakeholders, NGOs, and Social Enterprises. The ORF solution can be easily integrated with any existing government/partner tech platform that is used by teachers. It is easy-to-use software that requires minimal to no training for teachers to adopt the technology.
Revenue Model:
As a not-for-profit organization, our revenue model is primarily based on grants and funding from philanthropic organizations, government agencies, and other donors who are committed to supporting technology and educational initiatives. We may also generate revenue through fee-based services for private schools and Ed-Tech platforms. We will provide models as a service (fost per to cover expenses for maintenance and upgrade. We have already received requests to use the model on a per assessment basis.
our focus remains on grants and funding to make sure of the accessibility, affordability, and sustainability of our programs for underserved communities.
- Government (B2G)
Through the partnership with ConviGenius, our finding requirements are for improving the product by adding new features for remediation, 15 new languages for Scale, and post-deployment.
As we develop models for remediation and different languages we will deploy via CG for public schools. CG and/or the state government cover the compute and infrastructure costs in this deployment setup.
For private schools and Edatech platforms, we will provide models as a service (cost per unit) covering the cost of maintenance and upgrade. We have already received requests to use the model on a per-assessment basis. Further, we will also raise funds for R&D, in which we have received interest and are in conversation with two donors: Meta and BMGF
Senior associate partnerships and fundraising