HumekaFL
- Rwanda
- Not registered as any organization
Birth Asphyxia (BA) is a severe condition characterized by insufficient supply of oxygen to a newborn during the delivery. Timely intervention by medical teams, a few minutes after birth, is critical for rescuing infants from BA. BA is recognized as a prominent contributor to neonatal mortality with 2.3 million deaths recorded globally in 2022 (WHO, 2024) which is approximately 6300 deaths every day (UNICEF, 2024). Although there has been a decline in neonatal deaths over the past two decades, the developing world, particularly sub-Saharan Africa and southern Asia, continues to experience the highest under-five (<5) mortality rates. In Rwanda, BA accounts for 39% of neonatal deaths. Similarly, BA was reported to be the cause of 16.2% of admissions to Neonatal Intensive Care Units (NICUs) in Ghana, 12.5% in Ethiopia, 22% in Uganda, and a staggering 51.3% in Nigeria. Furthermore, it is projected that around one million survivors of BA grapple with neurocognitive challenges, and a subset of them may even develop motor disabilities later in life. Typically, these enduring disabilities become evident around the age of 18 months. These action-calling facts have prompted the United Nations (UN) to prioritize the end of preventable deaths of newborns and children under 5 years of age, with all countries aiming to reduce neonatal mortality and under‑5 mortality through the Sustainable Development Goals (SDGs) 3.2.
HumekaFL is developing a low-cost and easy-to-use (requiring no training) diagnostic tool integrating a mobile application (compatible with Android and iPhone smartphone platforms) and a wearable device for nurses and pediatricians to help nurses and pediatricians in detecting the presence of neonatal birth asphyxia using the newborn cry or whimper, coupled with other health indicators like heartbeat rate, temperature, and the birthing parent’s clinical history. Our solution will complement established evidence-based healthcare methods such as the Appearance, Pulse, Grimace, Activity, and Respiration (APGAR) score or other predictions modalities like images of the baby. Our solution is AI-based and not only helps detect the presence but also the severity, mild, moderate or severe of birth asphyxia, thus facilitating its early detection and treatment. Our wearable device embedding our AI model automatically records the baby’s voice or acquires the baby's image and audio feedback to health professionals for decision-making. Unlike existing solutions for AI-based detection of birth asphyxia, HumekaFL directly incorporates privacy and security concerns by design ensuring that the health data utilized to train our AI models remains securely stored within the premises of healthcare facilities and institutions.
HumekaFL aims to eradicate neonatal mortality and help save newborns’ lives all over the world, by helping health professionals flag early any potential disease that can be linked to birth asphyxia and endanger the new baby’s life in the future. Our solution can also contribute to diagnosing potential diseases or anomalies on the mother’s side that increase risks of birth asphyxia and research in healthcare. Birth asphyxia mortality is more prevalent in the developing world, specifically sub-saharan Africa which bears the highest mortality rates globally. Thus, we will primarily focus our effort in decreasing newborn mortality in the developing world; however, HumekaFL’s solution can be scaled easily in the global north to improve healthcare delivery and healthcare cost in these regions as well. For example, it may reduce diagnostic costs for low-income families in the United States, as it can serve as a substitute or screening tool for utilizing expensive existing diagnostic methods such as Magnetic Resonance Imaging (MRI) or Near-Infrared Spectroscopy (NIRS). In Africa, where such sophisticated methods may require prohibitive expense, healthcare professionals may rely on evidenced-based interventions to detect birth asphyxia that can be prone to errors in early detection of BA due their subjectivity both in detection and interpretation and limited assessment time. HumekaFL leverages AI’s potential to enable timely, accurate detection of BA and drastically reduce the cost of diagnostic technologies. Specifically, it adopts a federated learning paradigm, which preserves the privacy of data used to train our BA diagnosis model and use the model to predict the presence and severity of BA. Thus, HumekaFL does not require its users to give up their privacy in exchange for the low cost benefits of an AI-powered solution. Combined with user-friendly mobile software and wearable technologies, we are providing a unique solution that can be used by both clinicians and non-clinicians such as postpartum mothers and verified caregivers. Our solution’s affordability and robustness will highly impact resource constrained medical settings such as those in Africa and Asia to improve their newborn care healthcare services. If integrated in healthcare systems in the global north, HumekaFL can drastically reduce insurance costs and alleviate economic burden to low-income parents.
We are a group of people with a diverse and multicultural background. Our team currently counts five different nationalities. We are from Benin and Nigeria in West-Africa, Burundi and Ethiopia in East Africa and the USA in North America. Our diverse background is a huge asset for the team because we come from some countries that have the highest prevalence in birth asphyxia mortality globally. For example, Nigeria has the greatest burden of birth asphyxia in sub-Saharan Africa while the prevalence of BA in Ethiopia is approximately 22.8%. Therefore we represent the people suffering more from newborn mortality and this gives us a good perspective of the problem we are trying to solve but above all, hope to save the lives of our people. Coupled with our physical location in Kigali, Rwanda, this background positions us well to partner with local hospitals and healthcare organizations in Africa to deploy our solution. Moreover, one of our team members is from the global north and brings a unique and crucial perspective of the developed world and how we can scale and adapt our solution to this geographical context. Finally, diversity gives us a cross-cultural intelligence enabling us to interact more easily with people both clinician and parents around Africa and the USA and therefore getting the right information from people to design and develop a customized solution for our target populations.
- Ensure health-related data is collected ethically and effectively, and that AI and other insights are accurate, targeted, and actionable.
- 3. Good Health and Well-Being
- 9. Industry, Innovation, and Infrastructure
- Concept
So far, we have developed a hybrid mobile application embedding a machine learning model designed using a distributed learning technique, federated learning, which guarantees privacy and security of training data. This model has been trained on an existing dataset of newborn cries and performs well on this dataset, and we gave a demonstration of this prototype at the West Africa Conference on Digital Infrastructure and Cybersecurity. However, our current prototype has not been tested clinically in health facilities and does not use African health data.
We are applying to Solve for two main reasons. First, we need funding to develop our product. Our operating expenses will be for personnel (including two research engineers to extend the existing prototype and one project leader to oversee development and pursue partnerships with healthcare facilities) and equipment (primarily a cloud-based server to host the application backend, and mobile devices to test our application). Our solution is designed to scale to multiple hospitals and other healthcare facilities, so we do not expect these operating costs to increase significantly even if we undertake larger-scale deployments. Since we aim to primarily serve patients at under-resourced hospitals, it will be difficult for them to directly support the development of our solution. Second, we want to have access to Solve’s global network in the healthcare space and beyond to create solid collaboration for a large adoption of our product for a big impact in both the developing world and the developed one. More than a solution, we want to be a global platform to solve some of the most critical healthcare problems by leveraging human-centered technology to save the lives of people around the globe in a responsible and ethical way. We believe Solve is one of the best platforms to support us in our mission.
- Business Model (e.g. product-market fit, strategy & development)
- Financial (e.g. accounting practices, pitching to investors)
- Legal or Regulatory Matters
- Monitoring & Evaluation (e.g. collecting/using data, measuring impact)
- Public Relations (e.g. branding/marketing strategy, social and global media)
HumekaFL is an innovation because it leverages the power of collaboration at all levels to design and develop a robust, privacy-preserving, safe, responsible and scalable solution. HumekaFL’s backbone is its federated machine learning (ML) based software architecture, which enables many health organizations to train a shared ML pipeline without sharing their sensitive and private data or requiring direct communication among themselves. In a context where data privacy is paramount, such as cry-based detection of BA, this is truly an innovation giving full control to the data owner over the technology builder. Healthcare institutions therefore do not need expensive, large, powerful and costly computational resources to participate in this collaboration, which is especially important in resource-constrained environments like many locations in Africa. Moreover, an individual (e.g., parent or verified caregiver) using a simple smartphone can contribute to a shared effort (by recording a baby’s, taking a picture and more) to help in decision-making without compromising privacy. Knowing that Africa has the highest mobile technology penetration globally but lags behind in technological innovation to solve societal problems, this is a breakthrough enabling every single African to contribute responsibly and safely to saving lives without waiting for the governments, international organizations or the global north support. Our solution is therefore a new way to solve the developing world's problems by taking into account its strengths and weaknesses.
In the developed world, we envision to reduce the cost of healthcare services which is a barrier to middle and low income families. We believe our technology can optimize cost for these populations while not compromising their privacy.
HumekaFL will reduce birth asphyxia through a user-friendly and low-cost mobile application and wearable device to help healthcare providers diagnose the condition, while preserving health data security and people’s privacy.
Our impact goal is achieving the UN Sustainable Development Goal 3.2: Reduce avoidable death in newborns and children under the age of five. The key metrics of our impact will be the reduction of daily deaths and thus reduction in motor and cognitive disabilities caused by birth asphyxia in the developing world and beyond. We plan to concretely measure our progress towards these goals through monitoring the prevalence of BA and number of newborns who successfully receive treatment for it in hospitals where HumekaFL is deployed. We will also conduct interviews with doctors and clinicians who work with our solution to qualitatively understand how and whether it has had an impact on their ability to diagnose BA.
HumekaFL is a technological innovation.
First, our software is a framework using federated learning (FL) to train its model. FL is a distributed learning approach which uses the power of collaboration to create robust and accurate ML pipelines. Specifically, instead of using one single centralized client (which can be seen as a hospital or any health institution’s computational infrastructure) to leverage an ML pipeline, we train an ML model collaboratively over multiple hospitals with their data securely stored in their premises without communication between hospitals (clients). This approach presents two major benefits. (1) Health data is very sensitive and hosting it on a centralized infrastructure is not safe and might be very resource intensive. In many cases, it requires very large amounts of storage (e.g., images or videos of neonates) and heavy computational resources, which is not optimal in resource-constrained environments like those in African hospitals. Federated learning instead leverages existing computation and storage infrastructure at hospitals. Furthermore, our training approach mitigates by design security issues that health data might be subject to in centralized ML by first enabling health facilities to keep their data stored locally and then using a shared effort to train a powerful model. Because this is a shared effort, small data limitations in one institution might not be a reason not to have access to the best technology (ML model) to save the lives of people. This can also be viewed through the lens of this popular African proverb “If you want to go fast, go alone. If you want to go far, go together” and its Ubuntu philosophy that supports collectivism over individualism. In Ubuntu, “a person is a person because of or through others” and we believe that the developing world can improve its healthcare system using this core principle incarnated by Ubuntu through unity, trust and collective effort. (2) FL allows easy incorporation of privacy concerns for robustness and we intend to use them to prevent malicious attacks ensuring the privacy and security of health institutions’ data. Privacy is also maintained during inference after training because data collection and model inference are done locally.
HumekaFL, unlike available infant diagnosis tools like Ubenwa, is a tool that uses the best from evidence-based practices and combines them with other clinical criteria that can serve as indicators of birth asphyxia. HumekaFL uses cries, images of the baby, the APGAR score, clinical history and other modalities to develop its solution. This presents more chances for better detection but also to assess the severity (mild, moderate or severe) of BA for better treatment in the future.
Finally, HumekaFL ML model’s is deployed on a mobile application and extendable to wearable devices. Imagine a device recording automatically the baby's voice, taking pictures through an integrated camera coupled with other data stored in memory giving automatically the baby's health condition to a nurse (wearing) while giving birth. This is truly an innovation and that’s our vision for the future.
- A new application of an existing technology
- Artificial Intelligence / Machine Learning
- Imaging and Sensor Technology
- Internet of Things
- Software and Mobile Applications
- Rwanda
- Kenya
- United States
Five people are currently working in our team. Two (2) funding members, one (1) full-time worker and two (2) consultants.
We have been working for a year.
We have a diverse team with different cultural backgrounds. We are from west-Africa, east-Africa and the USA. Because we come from different cultures, working together is an amazing learning experience every single day. We are a group of students, alumni from the same school working together with our course instructor (faculty) or ex-Instructor for some of the team members on a project we believe will change the lives of millions of people around us. This shows that a teacher-student or student-supervisor relationship can lead to bigger collaborations. While mutual respect is paramount, we have an unconventional work culture where everyone has the opportunity to speak freely his/her mind without judgment. Titles matter less and active listening, jokes and camaraderies are more valued. Our team counts two out of five women, and we intend to increase the number of women working on the project in the future.
We have a Software as a Service (SaaS) model to generate a sustainable income stream for maintaining our solution.
Freemium option
Application download
Choose 2 modalities of detection, e.g. cry and baby image
Tutorials for newborn care and best practices during pregnancy and after gestation.
Paid option
Choose more than two modalities for detection, e.g cry, baby image, pulse and heartbeat rate
Online consultation with our network of professionals
Physical consultation with our network of professionals thanks to our optimized algorithm to geolocate the nearest hospital and expert.
API for integration in health facilities monitoring devices and wearable devices like smart watches
Wearable device as a diagnostic tool for medical doctors
Customized infant health monitoring from birth with partnered hospitals and experts until the age of 3.
- Organizations (B2B)
Until we are able to deploy our solution in hospitals and collect revenue from them (see our business model above), we intend to raise money to support HumekaFL’s development through applying for research grants but also through programs such as Solve. Our project is currently supported by a one-year grant from the Upanzi Network at Carnegie Mellon University’s CyLab Institute in Africa. We have also applied for additional funding from the Afretec Network, of which CMU is a member.
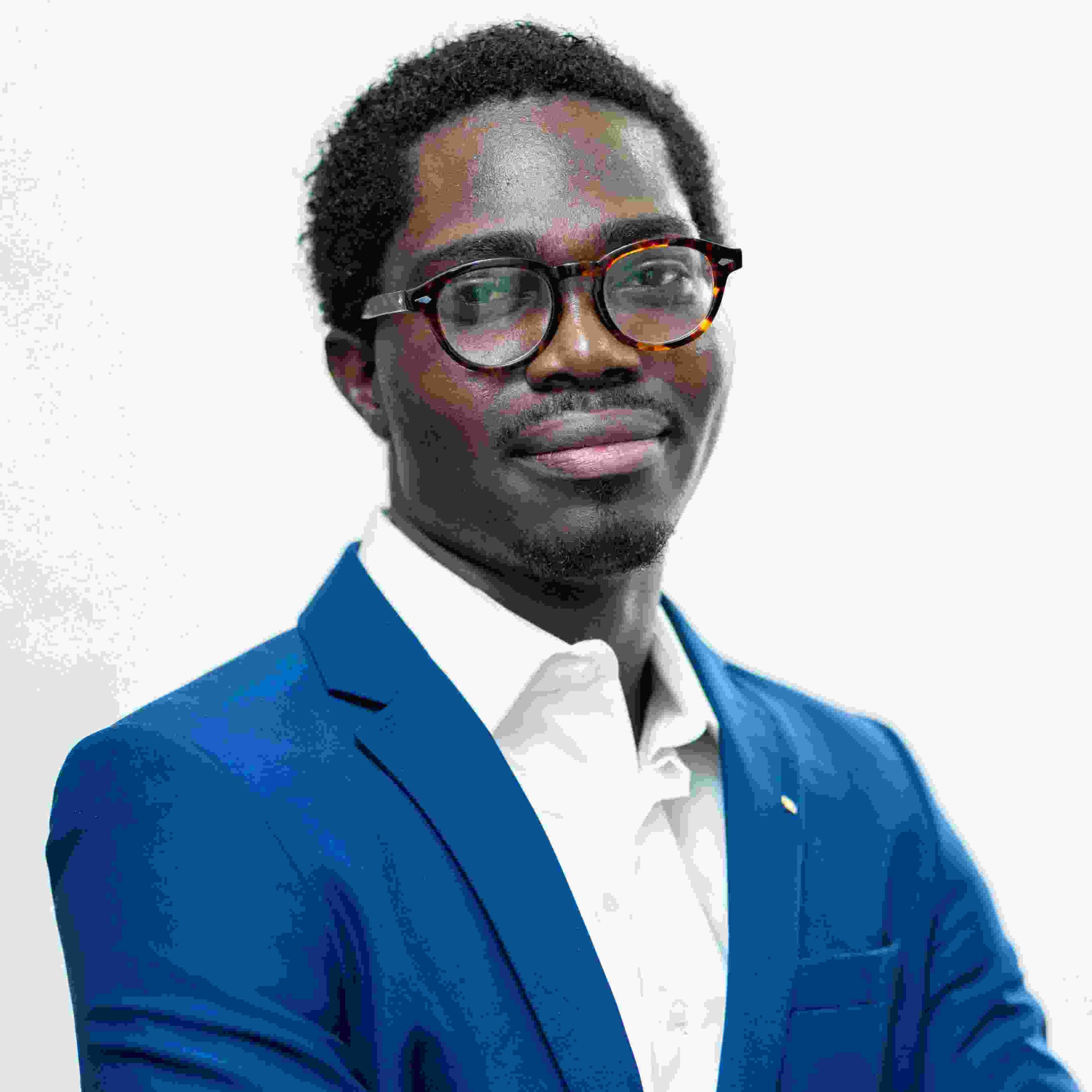