Medical claims AI assistant for businesses and providers
- Brazil
- For-profit, including B-Corp or similar models
Every year in Brazil, $5.4 billion is expected to be spent on fraud and misuse of health insurance, and another extra $5 billion on preventable private hospitalizations for chronic diseases. This makes insurance costs the second largest expenditure for employers, just after employee payroll, and they continue to rise above inflation rates each year. As a result, 80% of all Brazilians don’t have access to health insurance, primarily due to high prices, and at the whole Latin America and other developing countries, individuals without health insurance are often forced to contend with overcrowded public hospitals and limited resources.
Talking to potential users, I understood that health insurance claims data are seen as a "black box" by HR and Healthcare Teams, being hard to manage and decode. With constant claims from hundreds or thousands of employees, it is even tougher to prevent fraud, handle misuse, and be precise in selecting personalized preventable care for each member at risk.
Before Blue AI, employers were blind about the claims and only invested in primary care for those who were already high-cost members in previous years (that did not necessarily present risks for the future). But, with software that is equivalent to a data science team providing actionable information from no-code and pre-trained ML models, in its first 4 months, Blue AI got two Billion-Dollar employers paying for the platform, and 16,163 members. Today, we are tracking the health plan of 43 thousand members, in other words, in only 3 months later we grew 2.2x.
The first unicorn using Blue AI shared the feedback that they were able to negotiate a 30% discount on its health insurance price based on the audits provided by our Beta, and from that provide insurance to a large number of employees (which is the main goal at Blue AI, provide accurate and actionable information that can make healthcare accessible for those who didn't have it in the past). The second one, a NASDAQ-listed company, found more than $200K in duplicated reimbursement as payment for medical appointments that did not happen (most of them came from dermatologists, raising suspicions about aesthetic procedures paid with health insurance).
Blue AI is an AI assistant that helps employers and healthcare providers in Latam catch fraud, misuse, and preventable hospitalizations from health plans, pointing out problems that businesses hardly see coming, can still be treated with $20 per month but might cost $10,000 per year, per member if not taken care of.
Today, it's a no-code AI platform, where HR and Healthcare Managers drag and drop claims database files, and select pre-trained machine learning models, based on XGBoost and NLP mainly, without coding or statistical expertise. Finally, Blue AI provides a list of which employees might need care to prevent a hospitalization that might come in the next 12 months, 6x more precise than investing in the previous high-cost members (how it is done today), and points out unnecessary duplicated procedures, incorrect reimbursements, and other potential misuse.
Users are going to be able to chat with Blue AI. By training a GPT with the results from the ML models, Blue AI is going to help users navigate through the outcomes provided, answer questions, and assist them by generating insights on efficient primary care investments that best fit members at risk, how to avoid the misused healthcare procedures found, and bring strategies to support users negotiate better terms with health plan providers.
1) Initial users: HR and Healthcare managers.
Description: In Latam is expected to have 4.2M employers struggling to provide health insurance as a benefit for its employees. Nowadays, all they have to manage the company’s second most expensive expenditure are dashboards with many fuzzy graphs of past analysis. We also understand that health insurance claims data are seen as a "black box", hard to manage and decode. With constant claims from hundreds of employees, it is tough to prevent fraud and challenging to handle misuse. But, with machine learning preventing accurately is more efficient than just being reactive and investing only in those that already had high costs in the past.
2) Who we envision to serve: Latino and developing countries' populations struggling to afford care.
Description: Regrettably, 80% of all Brazilians (150 million people) don’t have access to health insurance, primarily due to high prices. At the whole Latin America, individuals without health insurance (expected to be something close to 300 million people) are often forced to contend with overcrowded public hospitals and limited resources. Blue AI is being built to address those to more affordable higher-value care in the long term, by suggesting the right treatment at the right time, preventing complications that could overwhelm public hospitals.
To do so, we build ML to navigate healthcare data and generate accurate predictions. Blue AI initially provides a no-code AI platform that works with insurance claims data, as a strategy to get its first users demanding recommended care. From that, we envision creating a marketplace of personalized providers that best-fit members at risk, even those who can’t afford health insurance.
Pedro Freire - https://www.linkedin.com/in/pedrofreirep/
Bachelor in Business Administration at Insper (Brazil), specializing in finance, economics, and venture capital. Pedro has started his journey as a product designer working with mobile apps at a solar energy startup. But, having a strong background in statistics and economics, he has been working with healthcare and health insurance building AI applications since 2017, including a prediction app for diabetes that was awarded at the Brazil Conference of 2019 at Harvard and MIT.
Because of Blue AI, Pedro was recently named to MIT Technology Review's Innovators Under 35 list, in the field of Artificial Intelligence.
- Ensure health-related data is collected ethically and effectively, and that AI and other insights are accurate, targeted, and actionable.
- 3. Good Health and Well-Being
- 10. Reduced Inequalities
- Pilot
• On 02/2022, launched the very first MVP.
• On 12/2022, reached 600K employees' insurance claims data from free trials, used to train and validate the first algorithms.
• On 04/2023, launched Beta with paid month subscriptions. ARR was initially $828,00, considering the first subscriber only.
• On 12/2023, got $11,628 in annual recurring revenue from 6 active B2B users, which subscribed for a 12-month-long subscription.
• On 01/2024, got $16,560 in annual recurring revenue, leading to an average MoM growth rate of 39.3%.
• So far on 02/2024, got $21,324 in annual recurring revenue, from 10 active employers subscribed for at least 12 months, including one insurance broker that subscribed for the next 24 months paying for their clients.
* I'm raising prices by 50% for the next subscriptions, following users' promising feedback.
At Blue AI I'm building a pre-seed funding round and a founding team. Getting to boost those main goals with the Solve team, at the same time getting new doors opened to investors in the US to invest in Latam, would be astonishing.
Last, but definitely not least, I've been part of the MIT Global Startup Lab, the winner of HackBrazil from the Brazil Conference of Harvard and MIT in 2019, so I'm really motivated by being part of and contributing to the MIT community. It would be life-changing to be part of it once again and join people working to make a relevant change in the world building products people want through high-level technology.
- Financial (e.g. accounting practices, pitching to investors)
- Human Capital (e.g. sourcing talent, board development)
How easy it is to use AI with no code on our platform.
We understand that health insurance claims data are seen as a "black box", hard to manage and decode. But, with machine learning preventing accurately is more efficient than just being reactive and investing only in those that already had high costs in the past.
With constant claims from hundreds of employees, it is tough to prevent fraud and challenging to handle misuse. Making AI easy to use for predicting avoidable healthcare outcomes and for auditing misuse is guiding our users to:
- Invest accurately in healthcare providers and primary care that best fits members at risk; - Audit misused healthcare resources, such as duplicated procedures and erroneous reimbursements; - Negotiate better terms with insurance providers based on predicted outcomes that can be prevented.
Activities:
- Data Integration: HR and Healthcare Managers upload and manage claims data using a user-friendly, no-code interface on the Blue AI platform.
- Analysis Using Machine Learning: The platform utilizes pre-trained XGBoost and NLP models to analyze the data, identifying potential fraud, misuse, and high-risk health scenarios.
Outputs:
- Identification of Potential Risks: The AI identifies members at risk of hospitalization within the next 12 months with a precision that is six times higher than traditional methods.
- Highlighting Inefficiencies: It also flags duplicated procedures and incorrect reimbursements, helping to streamline healthcare provision and billing.
Short-term Outcomes:
- Reduced Immediate Costs: Early intervention suggested by the AI helps in managing conditions before they escalate into more severe and costly problems.
- Efficiency in Healthcare Operations: Reduced administrative errors, frauds, and duplication lead to cost savings and more focused healthcare delivery.
Long-term Outcomes:
- Sustainable Cost Management: Ongoing prevention of severe health issues and reduction in administrative waste lower health plan costs significantly over time. In real cases, in one year Blue AI has already proven over 200 thousand dollars in waste and potential frauds, in one employer only.
- Improved Health of Members: Early detection and intervention contribute to better health outcomes, reducing the likelihood and necessity of hospitalizations.
- Enhanced Organizational Health Literacy: As employers and healthcare providers become more adept at using the AI system, they improve their ability to manage health plans effectively.
- Increased Access to Healthcare Resources: By improving efficiency and reducing costs, employers are often able to negotiate lower health plan prices, as evidenced by cases where employers received a 30% discount on monthly health plan costs. This outcome not only demonstrates financial savings but also ensures that employees have better access to necessary healthcare resources without compromise.
Evidence to Support the Links:
- Research on Machine Learning Efficacy: Leveraging published studies on the accuracy of XGBoost and NLP in predicting healthcare outcomes provides scientific backing for the AI’s predictive power.
- Process Evaluations: Regular assessments of how well healthcare managers use the platform and the accuracy of its predictions can help refine the AI models and ensure they meet user needs.
- Impact Evaluations: Comparing healthcare costs and outcomes before and after implementing Blue AI will highlight the platform's effectiveness. Specific attention can be given to cases where health plan costs were reduced due to the platform's use. In most cases so far, Blue AI got to predict right 6 out of 10 avoidable hospital admissions, up to 12 months earlier.
How we are monitoring and evaluating:
- Talking to users for feedbacks: Incorporating feedback from users about the platform’s functionality and the relevance of its predictions ensures that the AI continues to meet the evolving needs of healthcare managers.
- Data Analysis: Ongoing analysis of the outcomes achieved through the AI’s predictions versus traditional methods will help quantify the benefits and refine the approach.
Impact Goals for Blue AI:
Reduce Healthcare Costs: Significantly lower annual healthcare expenditures for employers and healthcare providers by minimizing unnecessary hospitalizations and healthcare inefficiencies.
Improve Healthcare Accessibility and Quality: Increase the accessibility and quality of healthcare services for employees by using predictive analytics to intervene before health issues become critical.
Enhance Healthcare Efficiency: Streamline healthcare processes by reducing duplicated procedures and incorrect reimbursements, thus improving the overall efficiency of healthcare delivery.
Reduce Healthcare Inequality: Make quality healthcare more accessible and affordable for the broader population in Brazil, aiming to decrease the disparity in healthcare access.
Measuring Progress Towards Impact Goals:
To monitor the effectiveness of Blue AI in achieving these impact goals, including the new goal related to reducing inequality, the following specific indicators can be used:
Reduction in Annual Healthcare Costs:
- Indicator: Percentage reduction in annual healthcare costs per employee, comparing year-over-year data.
- Measurement Tool: Analysis of healthcare spending data before and after the implementation of Blue AI to quantify cost savings.
Improvement in Healthcare Accessibility and Quality:
- Indicator: Reduction in the number of hospitalizations per 1,000 employees due to preventable conditions.
- Measurement Tool: Health claims data analysis to track hospitalization rates and compare them against baseline data prior to Blue AI implementation.
Increase in Healthcare Efficiency:
- Indicator: Percentage decrease in duplicated procedures, incorrect reimbursements, and other types of fraud and misuse procedures.
- Measurement Tool: Reporting tools within Blue AI that identify and quantify inefficiencies in healthcare claims processing.
Reduction in Healthcare Inequality:
- Indicator: Increase in the percentage of the underserved population gaining access to preventive healthcare services.
- Measurement Tool: Surveys and healthcare utilization data to assess changes in healthcare access among traditionally underserved groups.
Alignment with UN Sustainable Development Goals (SDGs):
These goals are closely linked to several SDGs:
Goal 3: Good Health and Well-being
- Target 3.8: Achieve universal health coverage, including financial risk protection and access to quality essential healthcare services.
- Indicators for Target 3.8: Could include the proportion of the population with large household expenditures on health as a share of total household expenditure or income.
Goal 9: Industry, Innovation, and Infrastructure
- Target 9.5: Enhance scientific research, upgrade the technological capabilities of industrial sectors in all countries, particularly developing countries, including, by 2030, encouraging innovation and substantially increasing the number of research and development workers per 1 million people and public and private research and development spending.
- Indicators for Target 9.5: Could include research and development expenditure as a percentage of GDP or the number of researchers per 1 million people in the healthcare sector.
Goal 10: Reduce Inequality
- Target 10.2: By 2030, empower and promote the social, economic, and political inclusion of all, irrespective of age, sex, disability, race, ethnicity, origin, religion, economic, or other status.
- Indicators for Target 10.2: This could include the proportion of people living below 50 percent of median income who report having good or very good access to healthcare.
I've been collecting insurance claims data for more than a year, by not charging anything for our predictions. Today we have over 600 thousand employees' insurance claims data, and over 300 thousand of them we have 3 years of historical data.
From past insurance claims data, Blue AI uses machine learning to deliver actionable insights including:
1) Total Healthcare Expense Prediction: We use a time series approach with Facebook's Prophet algorithm, analyzing 24 months of past data to predict the next 12 months' healthcare expenses with an accuracy of 87%. 2) Avoidable Hospitalization Prediction: We employ XGBoost, using procedure and cost data, to predict hospitalizations 12 months in advance. The model provides the probability of each employee, older than 20 years old and with at least 15 procedures performed in the past, being hospitalized in the next 12 months (class 1). On average, our model gets to predict correctly 6 out of 10 employees that will be hospitalized, this is our recall. The precision for the class 1 of this beta version is 63%.
3) Fraud Detection: Our NLP model, based on Latent Semantic Analysis (LSA), identifies non-standard procedure descriptions, helping detect fraud and misuse in claims data. At least 10% of the claims each company shares do not follow any standard procedures terminology. We have been able to track almost 60% of non-identified procedures on our database until now.
Scientific Validation: Our approach is validated against clinical data in the paper 'Algorithmic Prediction of Health-Care Costs' by MIT CSAIL, demonstrating the effectiveness of the use of claims data for our prediction model: https://people-csail-mit-edu.ezproxyberklee.flo.org/gjw/papers/healthcare.pdf
- A new application of an existing technology
- Artificial Intelligence / Machine Learning
- Big Data
- Software and Mobile Applications
- Brazil
- Argentina
- Chile
- Colombia
- Ecuador
- Mexico
- Panama
- Peru
- United States
- Uruguay
1 full-time, which is me as a solo founder, and 1 part-time, a hired software engineer.
I've been building Blue AI full-time for the last 24 months.
Since I'm building a founding team, I have already created a vesting contract with a lawyer which has a clause that makes clear we are both committed to tackling Racial, Gender, Social, and Healthcare Access Inequality. We are just starting, but I seek this same value from others who might join the founding team next.
What is your business model?
At first, Blue AI is a software as a service that helps employers and healthcare providers use pre-built AI models with no-code to catch waste, fraud and predict avoidable hospitalizations.
Key Partners:
- Healthcare providers
- Insurance companies
- Corporate HR departments
- Technology partners (for maintaining, enhancing, and improving AI capabilities)
Key Activities:
- Data collection and management
- Continuous development and training of AI models
- Customer support and training
- Marketing and outreach activities
Key Resources:
- Advanced AI technology, particularly in XGBoost and NLP
- Data security infrastructure
- Skilled data scientists and engineers
- Customer service teams
Value Proposition: Blue AI provides a no-code AI platform that makes easy for employers and healthcare providers to manage misuse and predict health plan avoidable costs. It helps reduce unnecessary hospitalizations and medical claims errors from claims analysis. Blue AI not only cuts costs significantly, for catching frauds and waste in healthcare but also improves the quality of healthcare access, contributing to better overall health outcomes, by predicting hospital admissions that can be avoided with primary care.
Customer Relationships:
- Direct interaction through platform support teams
- Training sessions for HR and healthcare managers on using the platform
- Ongoing customer engagement and feedback loops to improve the service
Channels:
- Direct sales teams
- Online platform where customers can subscribe and manage their services
- Partnership and referral programs with healthcare providers and insurers
Customer Segments:
- Employers in Latam with at least 50 employees who offer health insurance as a corporate benefit
- Healthcare providers looking to reduce fraudulent claims and improve operational efficiency
Cost Structure:
- Development and maintenance of AI technology
- Salaries for data scientists, engineers, and support staff
- Marketing and customer acquisition costs
- Administrative expenses
Revenue Streams:
- Subscription fees charged to employers, priced at $170 per employer per month
- Possible additional revenue from premium services such as customized AI model development or advanced analytics reports
Social Impact:
- Direct Impact: Improved healthcare management leads to better health outcomes for employees, reduced healthcare costs for employers, and more efficient healthcare systems.
- Indirect Impact: As healthcare becomes more affordable and accessible, disparities in healthcare access within Latam countries are reduced, contributing to social equity in healthcare.
Market Potential: Based on the pricing model and the number of potential customers (employers with at least 50 employees in Latam), the total market potential is estimated at approximately $7.1 billion annually. This sizable market offers substantial growth opportunities for Blue AI.
- Organizations (B2B)
What is the plan for becoming financially sustainable?
Today, we charge on average $170 per employer, per month. Latam is expected to have 4.2 M employers with at least 50 employees, the ones that usually pay health insurance as a corporate benefit for its employees.
$170 monthly subscription x 4.2M employers x 12 months = $7.1 billion dollars market.
What evidence is that this plan has been successful so far?
In 6 months of beta, Blue AI got 10 active paid users and the average subscription so far is indeed $170 per month per employer. Here are the ones paying the most:
1. Gallagher Insurance (a top 4 largest insurance broker in the US) pays $1,380 for 23K employees - https://www.ajg.com/
2. XP Investimentos (Nasdaq-listed company) began to pay $540 in February 2024 and accounts for 11k employees - https://www.xpi.com.br/
3. Grupo Comollati (a top 5 Brazilian automotive distributor) began to pay $260 in February 2024 and accounts for 4k employees - https://www.comolatti.com.br/en/
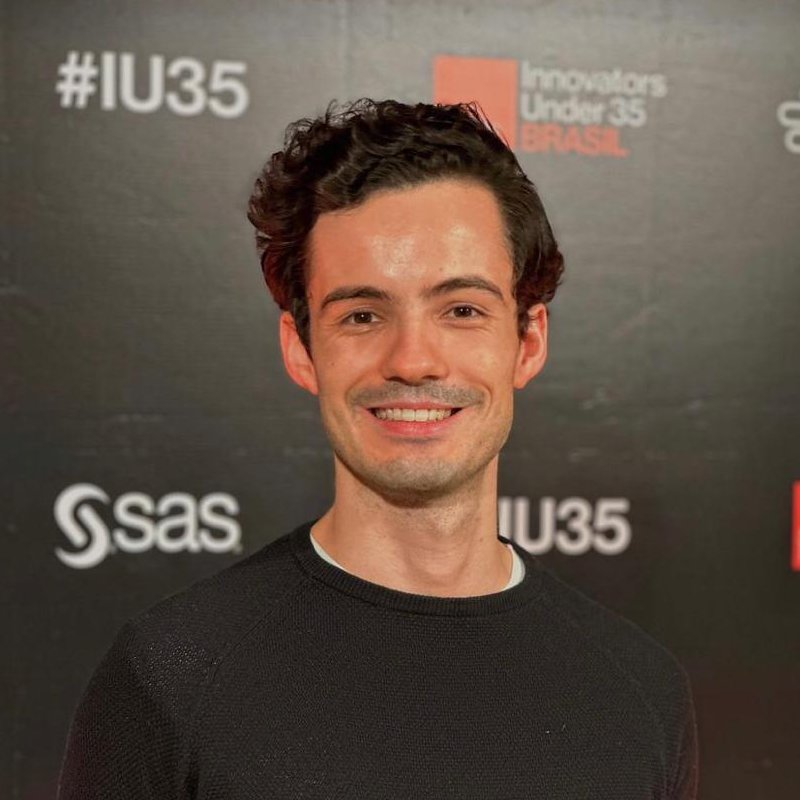
Founder