SAILFLOW (Smart Automated and AI-powered Last-minute FLOod Forecasting and Warning Station)
- Zimbabwe
- Hybrid of for-profit and nonprofit
Due to climate change, global warming is constantly increasing the energy in the earth’s meteorological system, thus increasing the frequency of torrential rain leading to more Flash floods, and urban flooding.
Developed countries are adapting to these issues by using advanced weather radars such as phased array radar and supercomputers to give accurate predictions, Hence lowering the mortality rate caused by floods there. This cutting-edge technology requires millions of dollars to run a year, a phased-started Radar costs about 50 thousand to install, and a phased array radar costs 5 million dollars. Factoring in the supporting infrastructure and trained personnel thus propelling the budget to roughly millions spent for a single operational station.
Many developing nations such as Zimbabwe can not afford to establish such technology, leaving them in danger. In the case of Zimbabwe, it currently has only three small, low-coverage radars, and uses a few international satellites to do its weather forecasting. This makes advanced weather forecasting difficult, and since weather forecasting plays a critical role in flood forecasting or monitoring, most Zimbabwe flood events are not forecasted. Leaving the 60% majority in rural areas in danger as they are the most affected by the flooding events.
In a nutshell, the rising frequency of torrential rains due to global warming puts many communities at significant risk of flash flooding, impacting both ravines and previously flood-free zones.
In essence, the core problems are:
It is harder for developing nations to have adequate flood forecasts since the current technology is expensive to establish
The inadequate distribution of existing weather systems, concentrated in major urban areas. While majority of the population stays in rural areas, this hinders even basic weather forecasting, leaving a gap between urban and rural communities.
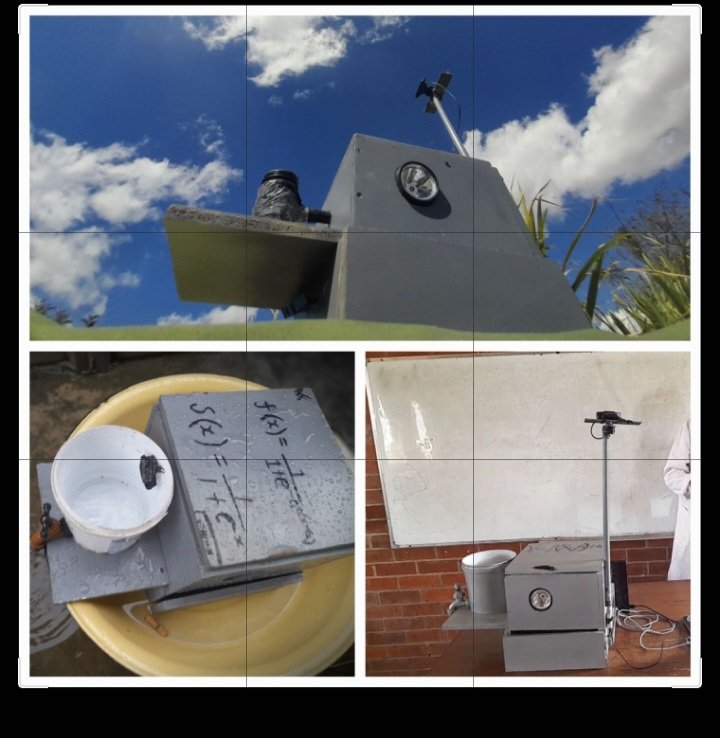
To solve that problem we asked this question: How do you create a low-cost solution that can be used to make accurate flash flood forecasting/prediction and carry out alert and warning protocols to the targeted community?
We developed SAILFLOW: A novel, low-cost, localized smart Automated, and AI-powered Last minute flood forecasting and Warning station. It’s a Stephenson screen-like device that is equipped with various weather sensors and microcontrollers, to create a portable automated weather station powered by solar energy.
What makes it Unique is the fact that it is based on two systems, the Flood Forecasting/Prediction Systems (FLOPS) and the Flood Alert SysTem (FAST), that work hand in hand in order to accomplish our engineering goals .
Flood Prediction System (FLOPS): we came up with an innovative way of forecasting floods, which is based on using AI models to forecast precipitation hourly( precipitation Nowcasting) using real-time weather datas, then use analytical techniques to forecast the water levels based on model the drainage or infiltration of an area. These models then work to predict different types of flooding(flash or ravine) depending on the Area. All the computations for the current prototype are done in Raspberry Pi, Jetson Nano, and Arduino microcontrollers. Although the majority of the experiments were tested separately.
Flood Alert System (FAST):
It's a combination of both low-tech and high-tech. It consists of a civil alert system made up of lights and sirens that are triggered by the FLOPS.It also uses IOT, and communication technology that will send SMS to most of the community members hours before a flood event occurs. Depending on the level of the threat( low, medius, high risk, existential) SMS are sent to community leaders, households, and nearest rescue centers depending on the threat, as well as crucial precautions. These Alerts are given in all three languages commonly used in Zimbabwe. For the current prototype, the lights and sirens are on the device, but they can be placed throughout the village and activated via radio, such as already existing systems.
Other applications:
the data collected and generated is not only vital for immediate flood warnings but also beneficial for agricultural forecasting. It can be stored locally on the device or uploaded to the cloud, where it can be accessed through smart mobile apps by farmers for enhanced agricultural planning.
This system also holds potential for further research and data collection in flood-prone areas, which are often under-documented. We hope to integrate it into the infrastructure of developing smart cities, connecting with existing weather stations and drainage systems to enhance urban flood management.
SAILFLOW will save lives against natural disasters, support agricultural activities, and contribute to vital environmental research.
Our solution, Sailflow, is specifically designed to serve rural communities in Zimbabwe and similarly situated regions in developing nations like Tanzania, Mozambique, and Vietnam, where access to reliable weather information and flood forecasting is severely limited. Approximately 60% of Zimbabwe's population resides in rural areas, distant from cities and towns, with limited weather monitoring infrastructure predominantly placed in urban or peri-urban areas. This distribution inadequacy results in a significant information gap between rural and urban dwellers.
Sailflow addresses these critical needs by focusing on two primary impacts:
1. Enhanced Flood Forecasting: With Sailflow’s a technology, we aim to provide lifesaving flood predictions. By predicting floods hours before they occur, our solution prioritizes rapid dissemination of warnings to enable timely evacuations and other preparatory actions. This capability is not just about information delivery; it's about giving rural communities the crucial time they need potentially saving lives during flooding disasters. We believe that during every dister every minute counts!
2. Reliable mini automated weather stations : Sailflow also offers a reliable source of weather and climate-related information that is accessible through smartphone apps. This service is vital for rural inhabitants, particularly those engaged in agriculture or other weather-dependent activities. By providing accurate and timely weather data, we empower these communities to make informed decisions that can mitigate risks associated with unpredictable weather patterns, enhance agricultural planning, and improve overall livelihood resilience.
By focusing on these areas, Sailflow not only bridges the information gap between rural and urban areas but also contributes to reducing the vulnerability of millions living in poverty within high-risk and free flood zones across, Sub-Saharan Africa. Our goal is to expand this service to have a significant global impact, starting with local communities in Zimbabwe and scaling up to reach other vulnerable populations worldwide
Type 1 Ai is a hybrid research focused startup founded by Tinevimbo Musingadi after realizing that AI has the potential to significantly advance scientific research with a direct impact on our community. Currently, we are focused on addressing issues prevalent in third-world countries, with one of our key projects being SAILFLOW. Our team at Type 1 Ai is uniquely positioned to deliver this solution, and at this moment, we are the only organization developing such a solution in Zimbabwe.
Despite being a team of recent high school graduates, the leadership and innovative approach of our founder, Tinevimbo Musingadi, are promising. His invention, SAILFLOW, has the potential to revolutionize flood forecasting. This journey began in 2019, sparked by the near-tragic experience of his grandparents during Cyclone Idai. Initially, the solution was merely a concept, hampered by both financial and technical limitations.
In September 2021, a generous science fair judge from California provided Tinevimbo with a laptop, enabling him to learn coding and further develop the solution. Over two years, he made significant progress, creating various versions of the prototype and iterating to develop the best one. Motivated by his low-income background, he understood the necessity for the solution to be cost-effective.
We are confident that our solution will be a great success as we have managed to overcome many hurdles during the prototype's design. Coming from a disadvantaged community, our founder had to learn coding and programming amidst frequent power cuts, often working through the night and then attending school during the day. We had to think outside the box to overcome these financial and technical challenges and to deliver the current version of the solution.
Currently, our team is very small, consisting only of the founder, Tinevimbo, and a friend who assists with some of the technical work. Due to our limited resources, we have not yet tested our solution in real-world situations, but lab tests and experimental results have shown promising outcomes. We are relying on our innovative approach to the problem, which we believe has the potential to make a significant difference.
We believe that with sustained support, we can continue to develop this solution. Most of the work on transitioning from concept to prototype has been done and is quite promising. Moreover, our founder remains energetic and committed to learning and growing the startup and advancing climate technology through Ai.
- Adapt cities to more extreme weather, including through climate-smart buildings, incorporating climate risk in infrastructure planning, and restoring regional ecosystems.
- 10. Reduced Inequalities
- 13. Climate Action
- Prototype
Our device is a low-cost automated flood forecasting station designed for both flood-prone and non-ravine areas. Our main goal is to provide a device that can forecast future flash flooding events and issue critical warnings to communities. We observed that remote and rural communities, particularly in developing countries, are often excluded from traditional weather and disaster forecasting. Our solution encompasses both hardware and software capabilities.
Our station is powered by two main systems: the Flood Prediction System (FLOPS) and the Flood Alert System (FAST), backed by an embedded system for data collection and computation.
Flood Prediction System (FLOPS): We developed a novel approach to flood forecasting, utilizing AI models to forecast precipitation hourly (precipitation nowcasting) using real-time weather data and cloud imagery. These models then use analytical techniques to predict water levels based on the modeled drainage or infiltration of an area. The models are designed to predict various types of flooding depending on the area.
Precipitation nowcasting:Our grounded precipitation nowcasting model can run on edge devices such as a Nvidia Jetson Nano or Raspberry Pi for the current prototype. The model uses data from sensors and cloud images, taken by a webcam attached to the device, to make hourly forecasts for up to 10 hours.
Analytical water level monitor:
The device records data on current precipitation and simulates drainage or infiltration using this data, alongside precipitation data, to predict future water levels within a ten-hour timeframe.
Current developments with FLOPS:
The ground-based precipitation nowcasting model is still in development due to data challenges. The current model was trained on open-source hourly data from a UK airport available on Kaggle and spatial data was trained using semantic segmentation of clouds from a university in Singapore through transfer learning. However, this model struggles with application in Zimbabwe due to domain shift, and the local meteorological department does not have the necessary hourly data. Despite these challenges, all other aspects of the prototype system function properly.
Flood Alert System (FAST):
FAST is a blend of low-tech and high-tech components. It includes a civil alert system of lights and sirens triggered by the FLOPS predictions. It also incorporates IoT and communication technology to send SMS alerts to community members hours before a flood, depending on the assessed threat level. Alerts are sent to community leaders, households, and nearby rescue centers along with crucial precautions, and are issued in the three languages commonly spoken in Zimbabwe. For the current prototype, the lights and sirens are mounted on the device but can be distributed throughout a village and activated via radio, integrating with existing systems.
Current standing with the FAST system:
This system performs well during prototype testing and can be further improved depending on the community it serves. Each station is custom-made for the specific community or area, with potential additions like radio or weather services accessible via the cloud.
Overall conclusion:
We are still at the prototype stage and aim to further develop the prototype and conduct pilot tests along the way. Our progress is mainly hindered by economic resources and lack of support, but we remain committed to refining the model. We are exploring the use of reinforcement learning to enable the model to adjust to local environments, an approach we refer to as "reinforcement learning through natural environment feedback."
I am applying to MIT Solve because, as a recent high school graduate (January 2024), I am seeking guidance on how to advance my startup, Type1Ai, and our solution, SAILFLOW, onto the global stage.
SAILFLOW was initially conceived to help people like my grandparents in rural areas access crucial climate disaster information. I first shared the idea with my geography teacher, who I thought could assist me. As I delved deeper into developing my concept, I encountered significant technical and financial challenges. Transitioning from an idea to a functioning prototype required me to learn and adapt continuously, as the problem was complex. I reached out to the Meteorological Department of Zimbabwe for assistance, but they lacked the technical expertise needed to refine my idea further. Over the years, I utilized MIT courseware to understand AI and learn the basics of economics to conduct a cost-benefit analysis of my tool until I stumbled upon MIT Solve.
I believe that MIT Solve, and MIT at large, can provide the technological support and expert advice necessary to refine and advance my project. MIT Solve is the perfect program to help bring my solution into the real world as quickly as possible. As this is my first venture into startups, the guidance from seasoned leaders would be invaluable for scaling my solution and making a lasting impact. At the moment my team is understaffed, we are still developing our business model, we are just new to this world of social entrepreneurship.
Because our solution is both on the technology dependedant and has to be cost effective, the 9-month MIT Solve program is exactly what I need to grow my team and learn the relevant skills to deploy my solution. I am confident that through MIT Solve, I will receive the expert guidance to development botht the solution and the startup to help me deloy the ideas. Through its vast network, I want to take that advantage to meet and collaborate with other climate tech leaders globally, as in Zimbabwe the space is just too small.
I plan to use the funding to initiate pilot testing in communities like Muzarabani, a town prone to flash floods, where SAILFLOW could have a significant impact. I also intend to experiment with other ways to enhance my solution and make it as accessible as possible to many people. Collaborating with other Solver teams, especially those in the climate technology space, would enhance our development and extend our reach.
I am confident that participation in Solve will empower me to not only build the startup I envision but also to effectively deploy a solution that could transform disaster readiness for vulnerable communities worldwide.
- Business Model (e.g. product-market fit, strategy & development)
- Financial (e.g. accounting practices, pitching to investors)
- Human Capital (e.g. sourcing talent, board development)
- Technology (e.g. software or hardware, web development/design)
Our project, SAILFLOW, redefines flood forecasting with a focus on accessibility and inclusivity. We ensure that no community is overlooked by prioritizing those who are typically disadvantaged in rural areas. SAILFLOW is versatile, serving both rural and urban communities effectively. In urban areas, its effectiveness is enhanced by aggregating data crowd-sourced from multiple weather stations, thereby maximizing its utility.
Traditionally, flood forecasting relies on data from radars, stream gauges, high-resolution satellites, and sophisticated models, including AI or analytical tools. While these methods are standard, they are prohibitively expensive for resource-limited areas. To address this, we have re-engineered our forecasting methodology. We have developed a novel technique that predicts precipitation on an hourly basis—precipitation nowcasting—using AI models. Crucially, instead of relying on expensive radar systems, we utilize images of clouds along with data from weather sensors to train a deep learning model for predicting precipitation. This method substantially reduces costs, making the system more affordable.
Additionally, we have innovated a novel rain gauge, the "Calculus Gauge," which functions similarly to traditional gauges but includes a tape that serves as an approximation for the drainage system or infiltration rate. By integrating this with our forecasting system, we can precisely predict when specific water levels will be reached, enhancing our ability to warn of potential flooding.
Our approach not only makes the solution more cost-effective but also revolutionizes flood forecasting. This could catalyze broader positive impacts by setting a new standard for affordability and accuracy in flood risk management, encouraging adoption in regions that previously could not afford such technology. As more communities are able to implement advanced forecasting technologies, the overall market for flood prediction tools could shift towards more innovative, inclusive solutions. This would not only expand the market but also drive down costs and foster competition, leading to continual improvements and wider accessibility.
In summary, SAILFLOW introduces a transformative, cost-effective method of flood forecasting that is scalable across different geographical and socio-economic contexts. This approach could redefine industry standards and inspire further innovation in the field, making advanced forecasting tools a viable option for communities worldwide, regardless of their resources.
Not sure how to respond
Activities:
Develop and deploy AI-driven flood forecasting technology: Utilize AI models that process cloud imagery and local sensor data to predict precipitation more accurately and affordably. The use of Ai model has been proven to work, based on current literature.
Implement the Calculus Gauge: Introduce a new type of rain gauge that integrates local environmental data to enhance prediction accuracy. This has been experimentally proven through model and the methodology is actually used other Calculus problems
Conduct community engagement and training: we will Educate and empower local communities, particularly in rural areas, on how to use and benefit from the new technology during pilot test
Immediate Outputs:
Availability of localized flood forecasts:Communities will have access to real-time, accurate flood predictions specific to their localities.
Increased community capability: Residents and local officials will have the knowledge and tools to respond proactively to flood warnings.
Enhanced predictive technology: The integration of AI and innovative measuring tools (like the Calculus Gauge) will refine the accuracy and reliability of flood forecasts
Short- to Medium-Term Outcomes:
- l Improved preparedness for floods: With better forecasts, communities can prepare more effectively, potentially reducing the impact of floods.
Reduced economic and human losses: Effective preparation and timely warnings can decrease damage to property and loss of life.
-increased trust and reliance on local forecasting: As communities witness the effectiveness of SAILFLOW, trust in local forecasting technology and methods is likely to grow.
Long-Term Outcomes:
-Resilient communities:Over time, as the forecasting technology becomes integral to local disaster management practices, communities are expected to become more resilient to floods.
-Policy adoption and scaling:
Successful implementation and visible benefits may lead policymakers to support broader adoption and integration into national disaster management strategies.
Evidence to Support the Links:
Third-party research: Studies have shown that accurate and timely flood forecasting significantly reduces economic and human losses.
- Pilot project data: Initial tests or deployments of the AI models and Calculus Gauge can provide empirical evidence of their effectiveness in improving prediction accuracy.
- Feedback from communities: Surveys and interviews with local populations who have accessed the training and used the technology can offer qualitative insights into its benefits and areas for improvement
Core Technology Components
1. AI-Driven Predictive Models:
- Functionality:Your solution utilizes artificial intelligence, particularly machine learning algorithms, to analyze vast amounts of data from cloud imagery and local weather sensors. These AI models are trained to identify patterns that precede weather changes leading to potential flooding.
- Advantage: AI enhances the accuracy and timeliness of predictions, allowing for more precise forecasting of flood events down to the local level. This means that communities can receive earlier warnings and more specific information, tailored to their geographical location and environmental conditions.
2. Embedded Systems in the Calculus Gauge:
- Functionality:Embedded systems play a crucial role in the operation of the Calculus Gauge, which is a novel type of rain gauge equipped with sensors and a unique mechanism (perhaps a tape or similar device) to measure and record rainfall and drainage data directly from the environment.
- Advantage: These systems allow for real-time data collection and processing at the site of installation. The integration of this data with AI models improves the forecasting model's input, thereby enhancing the overall prediction accuracy.
3. Fast Alert Systems (FAST):
- Functionality: Combining low-tech and high-tech approaches, FAST is designed to disseminate flood warnings quickly and efficiently across various platforms. This may include traditional sirens or loudspeakers as well as modern communication tools like SMS, mobile apps, and possibly social media alerts.
- Advantage :Ensures that all segments of the population, regardless of their access to technology, receive timely and life-saving warnings. This inclusive approach is critical in ensuring no one is left vulnerable due to technological limitations.
4. Flood Operation and Logistics Platform (FLOP):
- Functionality:This platform likely uses a combination of AI for data analysis and computational technologies for logistics and resource management during flood events.
- Advantage:Helps in planning and executing evacuation routes, resource allocation, and emergency responses efficiently. By leveraging computational logistics, FLOP can optimize response efforts in real-time, potentially saving more lives and reducing overall impact.
- A new application of an existing technology
- Artificial Intelligence / Machine Learning
- GIS and Geospatial Technology
- Imaging and Sensor Technology
- Internet of Things
- Software and Mobile Applications
- Zimbabwe
Currently two people work on the solutions.
full-tiime =1 founder Tinevimbo Musingadi
Part time =1
We have been working on the solution for two years. As part time project after school.
Once we grow, we are committed to ensuring equity by hiring people across all ages and genders. We aim to maintain a gender ratio of 1:1, actively fostering the participation of all genders within our workforce.
Additionally, we plan to engage six high school interns to assist with technical work, such as programming logic controllers and monitoring data. This initiative not only supports our technical needs but also provides valuable hands-on experience to young individuals from diverse backgrounds.
By integrating these practices, we strive to create an environment that reflects MIT Solve’s commitment to diversity, equity, and inclusion. We understand that diversity encompasses the social, cultural, and identity-based attributes within our team; equity means providing access to opportunities for all, addressing disparities and removing systemic barriers that hinder participation; and inclusion involves creating a welcoming and supportive environment where every team member feels valued and respected.