RARE-ML (Resolving Antimicrobial ResistancE by Machine Learning)
Pragmatic and dynamic solutions are needed to combat AMR. Data from patients and doctors will be gathered in an app, and machine learning will be used to understand the spread of diseases and AMR through time and space. Reinforcement learning will be used to dynamically suggest optimal therapies.
Team lead of an international consortium will be Markus Zeitlinger. He is an experienced physician and was involved in over 100 drug and MPG trials and 8 EU projects.
- Innovation
- Integration
Antimicrobial resistance (AMR) is a global health threat; rapid and timely identification of AMR may improve patient prognosis and reduce inappropriate antibiotic use. There is strong evidence that if the initial treatment is inappropriate, treatment failure, reinfection and death occur more frequently.
However, controlling AMR is a complex and multifaceted field. The clinical impact of AMR by itself is comparatively low if not assessed in the context of other factors such as patient risk factors, type of infection, and choice of antibiotic and its treatment regimen. Multivariate analyses are necessary to reveal the impact of resistance on duration of symptoms, hospitalisation, duration of ICU stay and death.
This highlights the need to consider complex patterns; however, linking individual patients to the most effective treatment regimen is difficult with current patient care models. Individual components that can at the same time easily be implemented in a broad population with moderate efforts and in a timely manner are needed. Action is required to ensure that professionals are not only educated and equipped with the core skills necessary for good prescribing practice, but are also supported by prescribing aids that apply local information on treatment success in real time.
Patients. Tailored treatments will be suggested to the treating doctors based on local AMR profiles and the most probable causative pathogens of the symptoms. Thereby, patient outcomes will improve.
Doctors. Treatment suggestions will be provided to doctors in the VRA using the learnings from the machine-learning algorithms.
Policy makers. The information provided in the VRA can be used by policy makers to adapt treatment recommendations for doctors.
- Pilot: A project, initiative, venture, or organisation deploying its research, product, service, or business/policy model in at least one context or community
- Artificial Intelligence / Machine Learning
- Big Data
- Software and Mobile Applications
Our solution will result in peer-reviewed publications about our methodological approach and the apps. The apps will be made available to any interested parties under licensing terms to be negotiated in order to result in the most impact in less developed countries and to ensure sustainable future development of our solution.
A key advantage of the proposed solution is that it will provide benefit to the patients dynamically during development with growing benefit as the dataset evolves. We hope – but obviously have to demonstrate first – that this will positively impact patients by providing them with optimized empirical therapy and thereby improve the outcome of their infections.
Our solution will have concrete impact by providing up-to-date, actionable information to patients, doctors, and stakeholders. Our machine-learning approach will extract as much information as possible from data collected by patients and doctors, and any learnings obtained from the data using modern algorithms will be made available to the general public, serving underserved and vulnerable populations.
The populations who help to develop and optimize the apps and algorithms will automatically benefit the most from the solution. We will therefore aim to provide the tool as cost efficient as possible to patients and caregivers.
Originally the solution will be piloted and then used in Ghana and Gabon. We will thereafter scale the impact of our solution by scaling it beyond the two initial countries, Ghana and Gabon. Scaling to more countries will increase the size of the data sets, ensuring that our methods will work even better, and thus provide more impact to the general public.
We will measure progress by comparing patients’ progress before/without our solution to patients’ progress and treatments wherever our solution is deployed. The speed of recovery can be directly extracted from the datasets we will collect, thereby providing specific, measurable indicators. Since a randomised controlled trial is not feasible given the setting and the currently provided financial support, non-interventional datasets will be used for comparison.
In our previous work (please see references above: [Bologheanu2023development], [Böck2022superhuman]), we have compared treatment strategies calculated by reinforcement learning to the performance of doctors as recorded in historic data, resulting in strategies that are at the level of doctors or better. The mortality was considered the specific, measurable indicator.
- Austria
- Gabon
- Germany
- Ghana
- Gabon
- Ghana
A specific barrier are privacy considerations in medical data collection. We will address privacy by ensuring that only pseudonymized data are stored and used, ensuring that all applicable laws are observed.
A possible barrier is the size of the datasets. We will address this by educational campaigns targeted at the general public to ensure that people understand the benefits and support data collection for the public good.
Availability of mobile devices is considered to be a minor barrier, but will be closely monitored to avoid a patient selection bias.
- Collaboration of multiple organizations
All parties are already well established and do not participate to boost their career. We are applying because we have the means to make a difference by a pragmatic solution that will quickly and dynamically overcome the AMR threat and improve the lives of patients in less developed countries.
Machine learning and patient based data collection has been neglected in the past. We are applying because we want to make state-of-the-art know-how in machine learning available to the general public, ensuring that everybody receives the best possible medical care.
Markus Zeitlinger is fellow of ESCMID (European Society of Antimicrobial Agents), expert to EMA (European Medical Agency), expert to GARDP (Global Antibiotic Research and Development Partnership) and is work package leader of several AMR accelerator projects (designed to overcome antimicrobial resistance). We will employ his network as well as his connections to WHO to make optimal use of the solution.
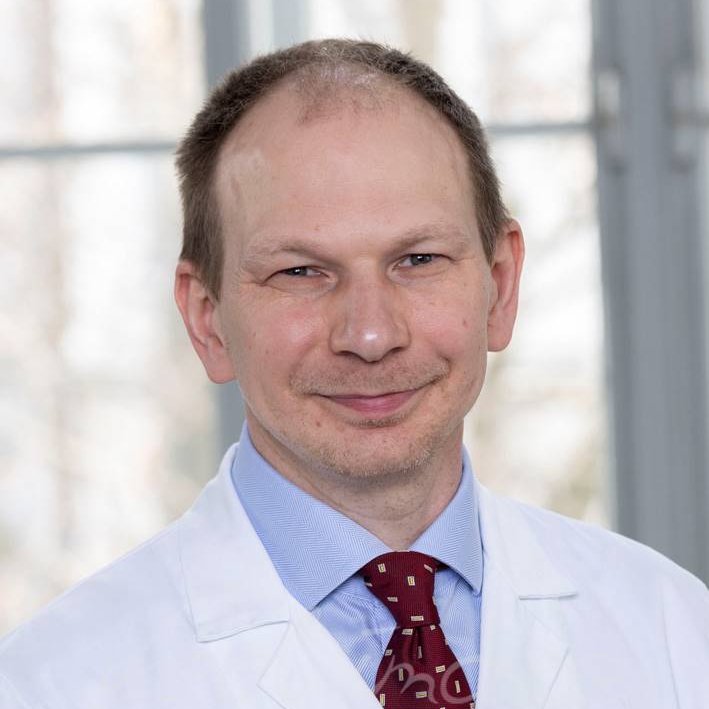