1Mpacto AZap: AI-Driven Antimicrobial Resistance Mapping in Brazil
1Mpacto AZap leverages advanced AI analysis of mass spectrometry data from Brazilian labs to detect subtle patterns in local bacterial strains, identifying hidden correlations with diseases and antibiotic resistance. This initiative aims to process and understand over one million data points, enhancing community-level insights for combating antimicrobial resistance effectively.
João Marcelo Occhiucci, Co-founder of AZap.ai. With a background in AI and its applications, João will be responsible for guiding the development and implementation of the solution.
- Innovation
Our focus is on leveraging the underutilized wealth of data generated daily by mass spectrometry (MS) devices in Brazilian laboratories. While these devices are prolific in generating data on bacterial strains, much of this potential remains untapped due to conventional analysis methods. We believe that by applying advanced AI techniques, we can unearth deeper insights from this existing data, specifically tailored to the Brazilian context.
The lack of precise, localized data (for the brazilian case) on AMR patterns makes it challenging to implement effective interventions. By calibrating and refining our data analysis to incorporate the Brazilian case specifically, we aim to bridge this gap. Our solution will enable more accurate estimates of the burden of bacterial diseases and antimicrobial resistance in local communities, impacting millions of lives.
Through our innovative approach, we will address several root causes of the AMR crisis in Brazil: inadequate surveillance of resistance patterns, poor understanding of local AMR dynamics, and ineffective policy responses due to a lack of specific data. Our solution will not only improve the understanding of AMR in Brazil but also set a precedent for other countries facing similar challenges.
By addressing the critical issue of antimicrobial resistance (AMR), we aim to enhance the effectiveness of treatments and improve overall health outcomes for these individuals.
The target audience includes:
Patients in Need of Accurate Diagnosis and Treatment: By providing healthcare professionals with more precise information about local AMR patterns, our solution ensures that patients receive the most effective antibiotics, reducing the risk of treatment failure due to resistant bacterial strains.
Healthcare Providers and Laboratories: Professionals in these settings benefit from our advanced AI analysis of MALDI-TOF mass spectrometry data, which helps them identify resistance patterns more accurately and quickly. This leads to better-informed treatment decisions and improved patient care.
To understand and address the needs of these groups, we are taking the following steps:
Collaboration with Laboratories Equipped with MS Technology (ex: Hospital das Clínicas São Paulo): We are partnering with laboratories, especially those equipped with MS or those that are our partners, to integrate our AI-driven analysis into their existing workflows. This allows for a seamless adoption of our solution in their routine diagnostic processes.
Utilizing Data from Instituto Adolfo Lutz, Instituto de Medicina Tropical and algorithms, ensuring that our solution is well-calibrated to the local bacterial profiles and AMR patterns in Brazil.
- Proof of Concept: A venture or organisation building and testing its prototype, research, product, service, or business/policy model, and has built preliminary evidence or data
- Artificial Intelligence / Machine Learning
- Big Data
- Biotechnology / Bioengineering
- Imaging and Sensor Technology
- Software and Mobile Applications
1Mpacto AZap provides a significant public good in the form of enhanced knowledge and data on antimicrobial resistance (AMR), which is crucial for the global fight against this growing health threat. Our solution contributes to the public good in several key ways:
Open-Sourced AI Model: The AI algorithms developed for analyzing MALDI-TOF mass spectrometry data will be made open-source. This allows researchers and healthcare professionals worldwide to use and adapt our models for their specific needs, fostering a collaborative approach to understanding and combating AMR.
Data Accessibility and Knowledge Sharing: We plan to publish our findings and insights in peer-reviewed journals and make them available through free-to-use dashboards. This ensures that the knowledge we generate is accessible to the global community, contributing to the broader understanding of AMR patterns, especially in Brazilian and similar contexts.
At-Cost Services for Developing Regions: Recognizing the importance of combating AMR in low- and middle-income countries, we aim to offer our analytical services at cost. This approach ensures that resource-limited settings can benefit from advanced AMR detection and analysis, contributing to global health equity.
The immediate output is the generation of detailed, accurate insights into the AMR profiles of local bacterial strains. This includes identifying resistance patterns that are specific to the Brazilian context, which have been underrepresented in global AMR research.
Outcomes: The practical application of these insights is multifold:
- Improved Treatment Strategies: Healthcare providers will have access to more precise information for prescribing antibiotics, reducing the risk of treatment failure and curbing the spread of resistant strains.
- Informed Policymaking: Our data will assist policymakers in crafting targeted AMR strategies, considering the specific AMR landscape in Brazil.
- Enhanced Public Health: In the long term, more effective management of AMR will lead to improved health outcomes, particularly for vulnerable populations who are most affected by drug-resistant infections.
Evidence of Impact: The effectiveness of MALDI-TOF in pathogen identification is well-documented, and the application of AI in data analysis has shown promising results in preliminary tests with datasets from Instituto Adolfo Lutz, Instituto de Medicina Tropical and Hospital das Clínicas São Paulo. By enhancing this existing technology with our AI approach, we anticipate a significant improvement in the detection and understanding of AMR, directly impacting public health management and patient care in Brazil.
Year 1: Expansion and Refinement
- Expand collaborations with additional Brazilian laboratories to access a broader range of data.
- Refine our AI algorithms based on feedback and initial testing results, enhancing accuracy and reliability.
- Initiate partnerships with healthcare providers for practical application and integration of our solution into their diagnostic processes.
Years 2-3: Wider Implementation and Global Outreach
- Extend our solution to a national scale across Brazil, encompassing a wide array of healthcare settings.
- Start publishing our findings and insights in international medical and scientific journals to share our learnings and methodologies globally.
- Engage with healthcare organizations and policymakers in other countries, especially those in low- and middle-income settings, to adapt and implement our solution to their specific needs.
Long-term Impact and Sustainability
- Continuously update our AI models to keep pace with evolving AMR patterns and bacterial strains.
- Develop training programs and workshops for healthcare professionals worldwide on utilizing our AI-driven insights for AMR management.
- Establish a sustainable model for ongoing data collection and analysis, ensuring that "1Mpacto AZap" remains a vital tool in the global fight against AMR.
Accuracy of AI Predictions: The primary indicator is the accuracy rate of our AI in identifying AMR patterns in bacterial strains. This is measured by comparing AI predictions against known AMR profiles in our database. Our pilot phase has shown promising accuracy rates, which we aim to continuously improve.
Adoption Rate in Laboratories: We track the number of laboratories integrating our solution into their diagnostic processes. Successful adoption in our initial partnerships with Instituto Adolfo Lutz, Instituto de Medicina Tropical and Hospital das Clínicas São Paulo will serve as a benchmark for expansion.
Healthcare Provider Feedback: Qualitative feedback from healthcare professionals using our insights for treatment decisions is crucial. We measure this through surveys and interviews, assessing satisfaction and practical utility.
Impact on Antibiotic Prescription Practices: In collaboration with healthcare providers, we monitor changes in antibiotic prescription patterns, aiming for a reduction in the use of broad-spectrum antibiotics and an increase in targeted treatments based on our AI insights.
Publications and Citations: The number of research papers and reports published using our data and insights, both from us and external researchers, serves as an indicator of our contribution to the scientific community.
- Brazil
- Bolivia
- Brazil
- Paraguay
- Peru
Financial Barriers: Developing and deploying advanced AI algorithms requires significant investment. We plan to overcome this barrier by seeking governmental grants, partnerships with NGOs, and potential investors interested in the social impact of our work.
Technical Barriers: The need for the collection, processing, and analysis of vast amounts of data presents a technical challenge. We are working on expanding our team with skilled experts and continuously enhancing our algorithms based on initial testing results.
Legal and Policy Barriers: Implementing AI technologies in healthcare requires compliance with stringent regulations. We are engaging with regulatory bodies early on to ensure our solution meets all necessary guidelines.
Cultural and Market Barriers: Adoption of new technologies in healthcare settings can be slow due to resistance to change and lack of awareness. We are planning training programs and workshops for healthcare professionals to facilitate the integration of our solution into their diagnostic processes.
To meet the minimum viable requirements in the target environment, we are bolstering our technical infrastructure, cultivating a multidisciplinary team, and establishing strong collaborative partnerships with laboratories and healthcare providers. This ensures our continuous suitability and advancement towards a sustainable model of data collection and analysis.
- Collaboration of multiple organizations
Our application to The Trinity Challenge is driven by our vision to deliver impactful development across populations through free, democratized access to artificial intelligence, with the intent to cause the greatest positive effect on health and other sectors. We see this challenge as a critical catalyst that can help us materialize our goal and address the multifaceted barriers we face.
By participating in this challenge, we can bridge financial gaps that are currently an obstacle to the extensive R&D required for our ambitious project. Furthermore, the collaborative environment fostered by The Trinity Challenge can help us overcome technical and regulatory barriers as we engage with a community of experts whose insights will inform and streamline our development process.
Aligned with the barriers identified earlier, The Trinity Challenge's resources and global network are especially important for engaging stakeholders and promoting cultural acceptance of AI solutions in healthcare, particularly within low- and middle-income communities where such advancements are most needed but often inaccessible. This aligns perfectly with our drive to realize large-scale, high-impact deployment in health and beyond, ensuring inclusive development and the welfare of all communities.
To advance and scale our AI-driven solution for antimicrobial resistance (AMR), we envision receiving support from Mérieux NutriSciences, an organization with a rich history in scientific innovation aimed at improving food safety, health, and sustainability. Their expertise would be invaluable in enhancing our understanding of the food industry's influence on AMR patterns, particularly within low- and middle-income communities.
In addition, collaboration with Big Tech companies like Microsoft, Google, and IBM would provide access to advanced AI technologies, cloud computing resources, and global insights into best practices in AI implementation and data security. These partnerships could significantly accelerate our algorithm development, data analytics capabilities, and computational power.
Finally, engagement with the World Health Organization (WHO) would offer guidance on aligning our solution with global health standards and priorities, facilitating integration into healthcare systems worldwide. It would also enhance our credibility and enable us to tap into a network of global health experts dedicated to controlling and mitigating the AMR crisis.
These collaborations would not only help address the technical and financial barriers we face but also ensure that our solution is scientifically sound, globally integrated, and designed to make a tangible impact on public health.
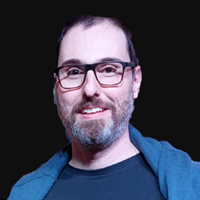
Co-founder
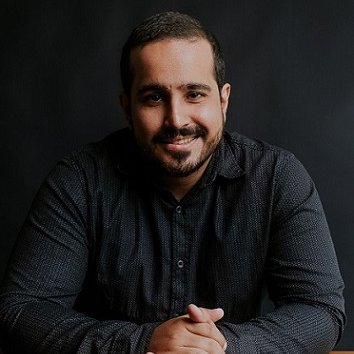
AI Startup Specialist
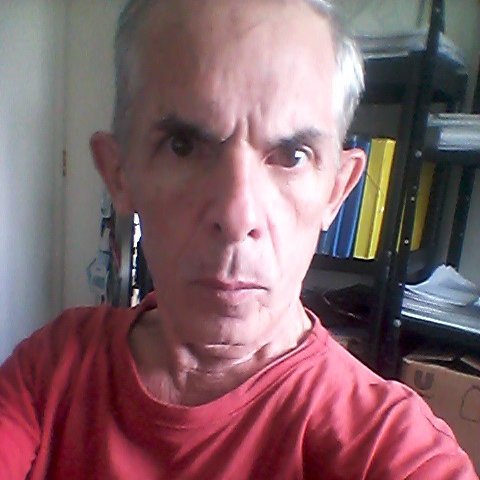
Assistant Professor
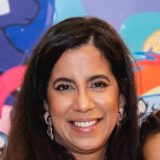
Doctoral degree
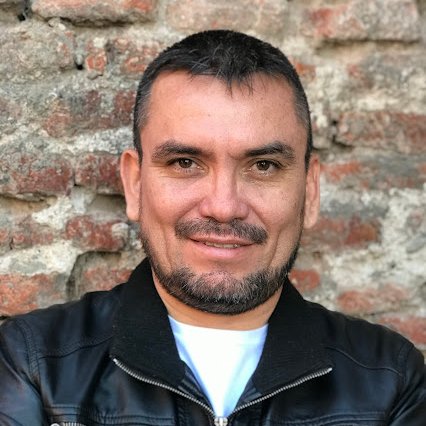