Predictive Analysis System for Antimicrobial Resistance Hotspots
Predictive Analysis System for Antimicrobial Resistance Hotspots (PASHAR) is our innovative machine learning solution designed to enhance AMR surveillance by integrating environmental sampling and genomics to identify patterns, predict hotspots, and inform effective public health strategies in Ghana and beyond
Ms. Nana Eghele Adade will lead as Principal Investigator. She is currently Technical Advisor and Lead Microbiologist on our AMR portfolio.
- Innovation
- Integration
- Implementation
AMR is a significant public health challenge in Africa, which has the highest AMR-related mortality rate globally. In Ghana, the AMR challenge is driven by misuse of antibiotics in both human and veterinary medicine, as well as the frequent use of antibiotics for non-therapeutic purposes in the agricultural sector. The complex interplay between these natural compartments and humans facilitates horizontal gene transfer among commensal and pathogenic bacteria. This highlights the need for comprehensive AMR surveillance beyond the present focus on clinical and veterinary contexts, which overlooks significant environmental reservoirs of AMR that can provide crucial insights into the prevalence and spread of antimicrobial resistance genes (ARGs) at the community level. As such, current AMR surveillance data in Ghana has significant gaps in community-level environmental sampling that limits the comprehensiveness of surveillance and the understanding of metagenomic drivers and predictors of AMR in communities. Notably, while the current surveillance strategy employs culture-based approaches, antibiotic susceptibility testing (AST) and whole genome sequencing (WGS) to predict genomic characteristics linked to AMR in bacterial isolates, this approach fails to capture the full spectrum of microbial communities and resistomes present in communities, thus overlooking critical ARG data.
The solution seeks to provide policy makers at the four AMR sector ministries in Ghana (Health, Food & Agriculture, Fisheries and Environment with a comprehensive tool for proactive surveillance and monitoring of AMR. By expanding data collection beyond clinical settings to include environmental samples, it offers a more holistic view of the AMR landscape, enabling authorities to better understand the spread of bacterial resistance and identify potential hotspots. The comprehensive surveillance data and predictive modelling capabilities enable policy makers to implement targeted interventions aimed at mitigating the spread of AMR and preserving the effectiveness of antimicrobial agents. Healthcare providers will benefit from this solution by gaining access to more detailed insights into the dynamics of AMR within their communities. By understanding the spread of resistant bacteria across different reservoirs, providers can implement more targeted treatment strategies and antimicrobial stewardship programs, ultimately improving patient outcomes and reducing the spread of resistant bacterial infections. Our solution will provide researchers in the field of AMR with a rich dataset comprising both clinical and environmental samples, allowing for in-depth analysis of AMR patterns and dynamics. This can also facilitate discovery of new resistance mechanisms and the development of novel interventions to combat AMR.
- Proof of Concept: A venture or organisation building and testing its prototype, research, product, service, or business/policy model, and has built preliminary evidence or data
- Artificial Intelligence / Machine Learning
- Big Data
- Biotechnology / Bioengineering
The open-source database of integrated WGS, metagenomics, and AST data to be developed in this project will be a crucial resource for understanding interactions between microbial communities and AMR phenotypes in a high-burden LMIC like Ghana. This database will aggregate detailed information on sample collection and sequencing alongside the abundances of genes, microbiota, and molecular functions from both clinical and environmental samples. A unified data-processing pipeline will ensure consistency and reliability in the data, enhancing the utility of the database. Key features will include tools for browsing and searching, equipped with descriptive attributes, gene sequences, microbiota, and functions, making the database user-friendly and efficient for researchers and public health officials alike. The significance of this resource in a nascent AMR surveillance system cannot be overstated; it would be a first-of-its-kind contribution to AMR surveillance through which researchers in Ghana and beyond will have streamlined access to vital data, facilitating other studies on AMR patterns, resistance gene abundances, and the impact of environmental factors on AMR. All publications stemming from this project will be published in open-access journals, democratizing access to enable a broad spectrum of stakeholders to engage in informed decision-making and policy development to combat AMR effectively.
We aim to create a tangible impact by enhancing AMR surveillance through a comprehensive and integrated approach, the benefits of which extend to both the general population and underserved or vulnerable groups. The logical link between our activities (expanding AMR surveillance to include environmental sampling and applying deep learning for data analysis), outputs (a more detailed and accurate understanding of AMR spread and resistance patterns), and outcomes (informed public health strategies and interventions based on a predictive model) is clear and direct.
By incorporating environmental samples alongside clinical data, our solution broadens the scope of AMR surveillance in Ghana, capturing a more complete picture of AMR dynamics. This comprehensive data collection enables the identification of AMR hotspots and transmission routes, including those affecting underserved populations who might be more exposed to environmental reservoirs of AMR due to factors such as inadequate access to clean water or proximity to livestock farming.
The use of deep learning to analyze this data ensures that insights derived are both precise and actionable. For vulnerable populations, this means targeted community-level interventions can be developed to address specific AMR threats they face, potentially reducing the incidence of resistant infections in these communities.
Over the next year, our focus will be on establishing and refining environmental sample collection strategies, protocols, and processes. These methodologies will be piloted in four regions to discern AMR patterns, with the expectation to collect and sequence at least 5,000 ESKAPEE isolates and associated samples in the first 12 months. Concurrently, the DL models will be fine-tuned to analyze this data.
In the subsequent two years, the team plans to expand its efforts to additional regions to achieve national coverage for our sampling activities. Early findings from the initial phase will be integrated into our existing clinical decision support tool for antibiotic selection, enhancing their utility and effectiveness. The team will continue to refine its DL models, both at the national and, if relevant, local levels, to assess AMR trends and understand patterns of spread more accurately.
This phased approach allows for iterative learning and adaptation, ensuring that the solution remains responsive to emerging AMR challenges. By systematically scaling impact, the solution aims to transform AMR surveillance and response in Ghana, providing a model that can be adapted and adopted by other countries in the region facing similar AMR burdens.
Short-term impact will be measured by the successful collection and analysis of samples, the development of an integrated database for AMR genomic surveillance, and the establishment of a framework for ongoing stakeholder engagement. In the long-term, the aim is to expand surveillance to national coverage, integrate findings into clinical decision support tools, and refine the deep learning models to provide actionable insights on AMR trends and spread. Impact measurement will include quantitative metrics such as the number of samples collected and analyzed, as well as qualitative feedback from stakeholders on the utility and effectiveness of our surveillance data in informing public health strategies and interventions.
- Ghana
- Ghana
- Nigeria
- Senegal
Over the next year and three years, the team anticipates various obstacles including financial constraints, technical complexities, legal and policy hurdles, cultural resistance, and market challenges. Chief among these is financial limitation, necessitating significant investment for extensive sampling and sequencing efforts. To mitigate this, the team plans to pursue grants and partnerships with public health organizations and research institutions locally and globally.
Technical challenges stem from the need for advanced deep learning models and quality microbiology labs. Leveraging institutional experience and collaborating with academic and research institutions for access to cutting-edge technology and expertise will be vital.
Legal and policy barriers, especially concerning data privacy and sharing, require navigating complex regulatory landscapes. Early engagement with legal experts and policymakers is crucial.
Cultural resistance might impede community participation in sampling efforts. To address this, the team intends to conduct awareness campaigns and community engagement activities to foster trust and understanding.
Additionally, challenges related to technology adoption will be tackled by demonstrating the value and efficacy of the approach through feasibility studies and open-access publication of findings to encourage wider acceptance.
- Nonprofit
Our application to The Trinity Challenge is driven by the critical need to advance AMR surveillance methodologies in Ghana, where the traditional surveillance mechanisms are inadequate against the multifaceted and severe AMR threat. We recognize that to develop the best tools for informing policy and guiding response, we must embrace more comprehensive and rapid innovation. The Trinity Challenge represents an opportunity to augment our burgeoning AI portfolio within AMR, invested by Gates Foundation and Fleming Funds to start, now ready to scale extending beyond stewardship innovations to encompass surveillance advancements. It provides a platform that could aid in addressing key barriers we face, including the need for advanced data analytics, integration of diverse data streams for a One Health approach, and the development of predictive modeling capabilities. Support from The Trinity Challenge would enable us to access critical resources, expertise, and networks to enhance our AI-driven solutions, ultimately leading to a more robust and proactive AMR response infrastructure for Ghana that may be reproducible in other African nation and LMIC contexts.
Partnerships with entities like the West Africa Center for Cell Biology of Infectious Pathogens and Kumasi Center for Collaborative Research will be instrumental for genomics analysis, while Google Research's advanced technology will support our DL model development. Collaborating with the Center for Scientific and Industrial Research and leveraging relationships with National AMR sentinel sites will facilitate comprehensive sample collection. We will also engage with the Bill & Melinda Gates Foundation to draw on a vast pool of AMR expertise and align our efforts with global standards, ensuring a robust AMR surveillance system that is both effective and scalable.
Aurum’s Business Development Unit based at HQ office connected through Vanderbilt University weekly grant opportunities list serve.
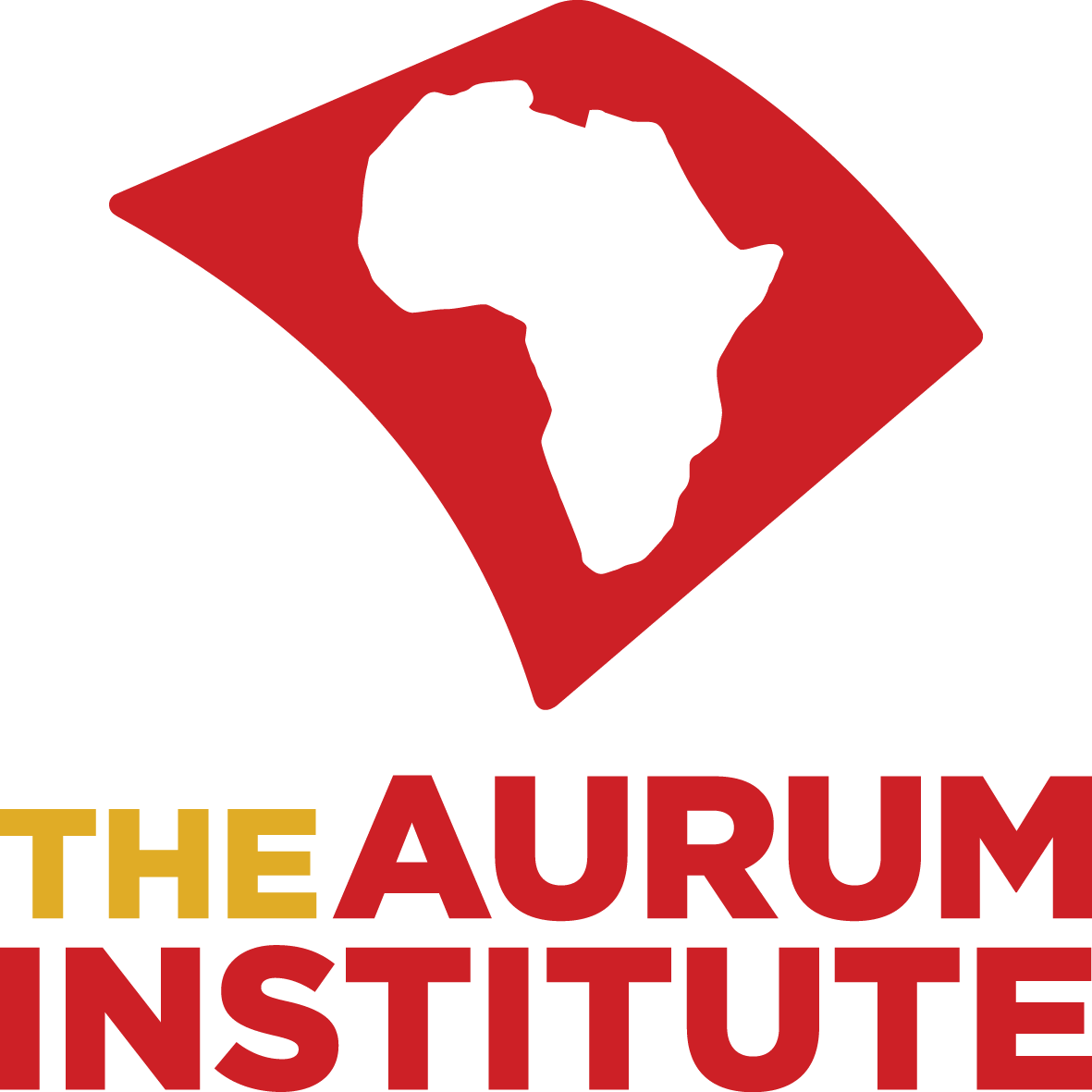