Smarter digital prescribing
Our solution uses local antibiotic resistance data from the community together with information on locally available antibiotics and follows the WHO AWaRe guidelines to inform prescribers to treat patients for the infectious syndromes they present with.
Our digital solution is available on a simple smartphone application available for prescribers.
Dr Catrin Moore (London), Dr Mathieu Raad (France), Dr Francois Kiemde (Burkina Faso), Dr Freddy Kitutu (Uganda).
- Innovation
- Implementation
Between 1.27 and 4.95 million people globally died due to antibiotic resistance in 2019 (GRAM project, GBD). This data is based almost exclusively on hospital data, it is difficult to determine the full burden of mortality and mortality due to AMR and to understand if our current estimates are over or under-estimating the burden of disease in humans. A small number of studies have begun to understand the burden of disease caused by antibiotic-resistant bacteria in some infectious syndromes, such as urine tract infections in the community, this remains a huge gap in our knowledge and evidence in which to build guidelines, tools, and policies. The global treatment guidelines (WHO AWaRe system) recommends using antibiotics that do not increase resistance in bacteria (those from the Access group of antibiotics), but the effectiveness of these drugs and guidelines against the infections locally are unknown.
Using local data available from community settings in two countries: Burkina Faso (West Africa) and Uganda (East Africa) on infectious syndromes, antibiotic resistance, and a list of available antibiotics in local pharmacies our digital tool will inform local prescribing practices.
Our digital tool is available to download on mobile devices and follows the 2021 AWaRe system.
The solution is aimed at several groups of people:
1. prescribers to provide personalised treatment for their patients based on local resistance, incidence of infectious diseases and available antibiotics
2. pharmacists to understand their prescribing patterns for different age groups, and provide an understanding of their prescribing practices, to understand the most prevalent infectious syndromes they have and to inform their ordering in the future (and over many seasons)
3. Ministries of Health to understand the denominators of infectious diseases, the bacterial causes, true burden of resistance and antibitiotic use locally
4. Ministries of Health to detect any new bacterial causes of infection and to pick up on any outbreaks that occur in the community through analysing the available digital data
5. local community users to understand if they think the data is useful for them and if they follow the treatment guidelines suggested or seek antibiotics elsewhere if they have not been prescribed
Within the social science arm of the study we will engage each on of these groups in focus groups and one to one interviews in each country to fully understand their needs, to modify our tool and update based on local feedback.
- Pilot: A project, initiative, venture, or organisation deploying its research, product, service, or business/policy model in at least one context or community
- Artificial Intelligence / Machine Learning
- Behavioral Technology
- Big Data
- Software and Mobile Applications
This solution provides a public good through both arms of the solution, the first one providing locally applicable prescribing for patients, and with the second solution we will better understand the local health-seeking behaviours to reduce inappropriate antibiotic prescribing in community settings. The solution focuses on treating patients appropriately based on local data reducing inappropriate antibiotic use in humans, thereby decreasing the drive for resistance and in the long term minimising AMR.
Our target population are ordinary people living in communities in Uganda and Burkina Faso. We provide their medical providers with a tool in their pocket to better treat the community based on the symptoms they present with. This has worked well in hospitals in Madagascar and Canada and is being trialed in Laos. Based on locally available evidence, prescribers can be confident in their treatment of infectious diseases locally. Through this work we will gain insights into the current treatment-seeking behaviour and begin to change the behaviour of the practitioners and community members by building confidence in the SmartBiotic tool.
In the long term, the reduction of inappropriate use of antibiotics using appropriate guidelines will have an impact reducing antibiotic resistance globally.
The first year we will collate local data from the collaborating organisations, from the community and hospitals to inform the algorithm and build locally applicable tools in the local language (French and English). At the same time, our social science teams will work in the community through mixed focus groups and one-to-one interviews to understand treatment-seeking behaviour and prescribing behaviour.
The understanding of the behaviour will help to inform the SmartBiotic tool to build in any local behaviours which might affect the way patients interact with prescribers and follow their advice, for instance, there may be certain language that is useful to persuade sick patients or their caregivers not to buy an antibiotic when it is not needed.
We will pilot the tool in a small number of pharmacies in the second year and seek feedback from the prescribers and customers to understand the usefulness of the tool and whether the customers follow the advice given or if they go elsewhere for antibiotics. Pharmacies will have continual feedback.
The third year we will discuss the outcomes of the work with the Ministries of Health and policy makers to roll the initiative out to more pharmacies in Uganda and Burkina Faso.
The measures we are using to monitor our project are to:
1. Capture local community data which includes the presenting infectious syndrome, sex, age, microbiology resistance data, antibiotic availability and antibiotic guidelines (WHO AWaRe system)
2. Clean and collate all data
3. Prepare the data for input into the SmartBio system for analysis
4. Training for the use of SmartBiotic in the community settings
5. Test the use of the programme in the community settings: first in a small number of outlets (pharmacies), and roll out if successful, if not to determine the bets method for roll out
6. Setup focus groups (FGs) and one-to-one interviews at the same time as data collection is ongoing to learn the local understanding and treatment-seeking behaviour
7. FGs to understand how acceptable the SmartBiotic tool is in the field
8. Determine the use of the SmartBiotic tool in the field: how many outlets use the system, how often is it used (daily click rate), which clinical syndromes are searched for most frequently
9. Determine the long term use of the tool - does use diminish over time, are users looking for more information (to collect this information through FGs and interviews)
- Canada
- France
- Lao PDR
- Madagascar
- Belgium
- Burkina Faso
- Canada
- France
- Luxembourg
- Switzerland
- Uganda
- United States
The main barrier to any work in the community globally is the lack of data to provide evidence. This is based on financial difficulties and the inequity of health across low- and middle-income countries to afford diagnostic tests and treatment (or to have the choice between the two). The informal treatment sector remains unregulated in many countries, partly based on a lack of evidence and guidelines.
We have identified two countries that have collected data on AMR and AMU who are willing to work collaboratively to use their available data to begin to address inappropriate prescribing in their countries. Through engagement with local pharmacists, we will support them to use local data and guidelines to inform their practice and reduce both inappropriate prescribing and following treatment guidelines based on local data.
- Academic or Research Institution
Currently, data informing the SmartBiotic digital tool is from hospitals containing functioning microbiology laboratories. Little or no data is available on antibiotic resistance outside large hospitals.
This exciting challenge encourages the production and use of data on antibiotic resistance and antibiotic use in the community which begins to shine a light on this gap on the available data and evidence. Overall, the production and sharing of this data will ensure better evidence followed by more appropriate guidelines and policies for treating patients who are sick in the community.
The Trinity challenge provides the funding to bring together researchers who have data in the community in Uganda and Burkina Faso to begin with, enabling them to use the digital tool and better treat patients with infectious diseases based on the prevalence of resistance and available antibiotics.
The SmartBiotic digital tool relies on local data to inform the algorithms and treat local patients more effectively based on local evidence.
For the data analytics using community data, mentorship from companies such as bluedot around our machine-learning solution, Zenysis around data harmonisation and the problems they have encountered and solutions they have devised around data linking, cuebiq for support on ethical and responsible data collection and use to ensure our solution follows the correct guidelines. We would also like to have mentorship from the Joep Lange Institute around working with Ministries of health to shape policy initiatives based on our digital solution and from Palantir in the UK to better understand UK community data (on AMR and AMU) and how they are storing and using the data effectively.
For the second area of our work, mentorship from the Behavioural Science consultancy to help us build a clear vision and structure for our work in this area together with the ability to seamlessly link both the electronic data and the health behavioural work.
I went to a talk given by Marc Mendelson in St George's, University of London where he spoke about the challenge which peaked my interest and we began discussing as a collaborative team.
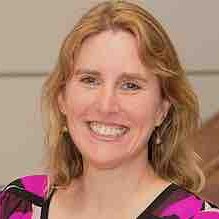
Dr
Dr
Dr