EmbMed
Description of the Problem: Deep learning models on healthcare medical images is one of the most revolutionary techniques for medical support and decision making. The challenge centers around the creation of deep-learning models for medical images. This endeavor demands personnel with highly specialized knowledge, substantial data resources, and significant computational power for training large-scale deep learning models. As a result, this problem limits AI use to be confined to privileged groups across the globe, thus exacerbating disparities in healthcare access and outcomes.
Scope of the Problem: The issue is not limited to one specific geographic region; rather, it extends globally, with a disproportionate impact on low- and middle-resource countries. However, even some institutions in high-income countries also face this problem, either due to insufficient resources or because they lack the required volume of data and computational resources. Given that our organization, MIT Critical Data, comprises an international team, we have the unique advantage of access to diverse perspectives and data from institutions spanning various continents, including Africa, and South America. Our scope for the next year is to explore the use of retina embedding images focusing on Brazil with the Brazilian dataset BRSET and the Uganda dataset (Under data collection process right now).
Relevance: The problem we're addressing is of supreme importance because medical research grounded in the analysis of medical images is one of the most essential techniques for supporting various medical tasks. However, it is important to recognize that healthcare solutions are not generalizable to all the populations. Populations differ significantly due to multiple factors such as genetics, social dynamics, and economic conditions, making it imperative for each country and institution to create their own models. The truth is that many of these entities are unable to do so due to the limitations previously outlined, thereby perpetuating health inequality. Our proposed solution offers an opportunity to empower institutions to conduct research and develop their own models through the utilization of vector embeddings extracted from (initially) their own medical retina images to be tested in multiple downstream specific tasks with high quality and reduced resource requirements.
Factors Contributing to the Problem: The multifaceted problem is underpinned by several key contributing factors:
Scarcity of Local Medical Image Data: Many regions lack a sufficiently substantial repository of local medical image data essential for training robust deep learning models.
Shortage of Specialized Personnel: The deficit in highly specialized personnel capable of creating deep learning models poses a substantial barrier in resource-constrained settings.
Inadequate Computational Resources: The insufficiency of computational resources required for training large-scale deep learning models using medical images presents a significant hindrance to progress in these regions.
These factors collectively hinder the advancement of medical image analysis, impeding equitable healthcare access and perpetuating health inequalities in these underserved communities and institutions.
Our solution, known as "EmbMed," addresses the critical issue of limited access to deep learning models for medical image analysis, Initially using retina images, from regions usually under-represented such as Brazil and Uganda. EmbMed leverages cutting-edge data science techniques to create medical image vector embeddings, revolutionizing the landscape of healthcare research by promoting equity in underrepresented populations.
Image embeddings are compact numerical representations that capture the essential information and features of a medical image in a format that can be easily processed and analyzed by machine learning algorithms. These embeddings condense the image data into a structured and meaningful format, making it possible to compare, analyze, and extract insights from medical images efficiently.
EmbMed uses self-supervised learning techniques to train foundational models of medical retina images. These foundational models serve as a universal building block for institutions in different countries and regions, enabling them to generate their own vector embeddings and develop models to address their unique healthcare challenges. Here's a brief overview of how EmbMed works:
Data Collection: We collect diverse medical image data from various institutions worldwide, initially from Uganda, and Brazil, ensuring the inclusion of usually underrepresented populations.
Self-Supervised Learning: EmbMed utilizes state-of-the-art self-supervised learning algorithms to train foundational models on this diverse dataset. Self-supervised learning allows the models to learn meaningful representations from the data without the need for extensive labeled data, making it a cost-effective and resource-efficient approach.
Vector Embeddings: The trained models generate vector embeddings for retina images. These embeddings capture essential features and patterns within the images, allowing institutions to utilize them as the foundation for their own custom models.
Customization: Institutions, regardless of their geographic location or resource constraints, can use these vector embeddings to create specialized deep learning models using their own images to their unique patient populations, healthcare challenges, and research objectives.
By offering this scalable and adaptable solution, EmbMed effectively democratizes medical image analysis and research, breaking down barriers related to limited data, specialized expertise, and computational resources. It empowers healthcare institutions worldwide to take control of their own healthcare research, thereby mitigating disparities in healthcare access and outcomes.
EmbMed's potential to address the global challenge of healthcare inequality by making medical image analysis accessible to a wider range of institutions is what sets it apart as a transformative solution. We are confident that with your support, we can make this vision a reality, and ensure that healthcare research is truly equitable and inclusive across the globe.
Our solution, EmbMed, serves to address the critical issue of limited access to deep learning models for medical image analysis in low-resource settings. The primary population we aim to serve are healthcare institutions and researchers in underserved regions, who currently face significant barriers in leveraging the power of deep learning for medical image analysis. These institutions and researchers are often unable to harness the potential of AI techniques due to factors such as a scarcity of large amounts of local data, a shortage of specialized personnel, and inadequate computational resources. As a result, they are unable to develop models that cater to the specific needs and characteristics of their unique patient populations, perpetuating health inequalities and disparities in healthcare access.
EmbMed directly impacts the lives of this target population in several meaningful ways:
Access to Deep Learning Models: EmbMed provides healthcare institutions and researchers in low-resource settings with access to deep learning models for retina image analysis. EmbMed becomes a critical resource for institutions that lack the means to develop such models independently. This access empowers them to perform retina image analysis, diagnose conditions, and conduct research with the aid of AI, ultimately leading to improved healthcare outcomes for their patients.
Customization for Diverse Patient Populations: EmbMed's approach is adaptable and flexible, allowing institutions to create specialized deep learning models tailored to the unique needs of their patient populations. This customization factor is essential as patient populations can differ significantly due to genetics, social dynamics, and economic conditions.
Resource-Efficiency: EmbMed's utilization of vector embeddings derived from self-supervised learning reduces the reliance on extensive labeled data and extensive computational resources. This approach is cost-effective and resource-efficient, making it accessible to a broader range of institutions, regardless of their resource constraints.
Global Collaboration: EmbMed's data collection spans low-represented regions, such as Brazil and Uganda, ensuring a wide range of perspectives. This fosters global collaboration, enabling institutions from different parts of the world to benefit from a diverse dataset and share their findings and insights. This collaborative approach promotes a more comprehensive understanding of healthcare challenges and solutions.
Healthcare Equality: By addressing the barriers to medical image analysis, EmbMed directly contributes to reducing healthcare disparities. Institutions in low-resource settings can now be on par with their counterparts in high-income countries when it comes to conducting research and providing healthcare services. This, in turn, leads to more equitable access to quality healthcare and improved patient outcomes.
Our team at MIT Critical Data is positioned to deliver the EmbMed solution, addressing the critical limitations to access to retina image research in low-resource settings, focusing this solution on Uganda and Brazilian data.
Our global and local experts have a wealth of experience in creating vector embeddings and datasets for various types of images, including ophthalmological images (https://shorturl.at/bguHI, https://shorturl.at/mpwAW), and satellite images (https://huggingface.co/MITCriticalData). We firmly believe in the importance of understanding local contexts and challenges, which is why our team is composed of individuals from diverse backgrounds and countries including physicians and data scientists from Uganda and Brazil. Through our extensive network and regular datathons held in over 50 countries, we've established strong connections with institutions and researchers worldwide, fostering collaboration and knowledge exchange.
Our Team is composed of a team of medical and data science experts from different specialties and regions of the world, including local experts. This ensures that we have the necessary domain knowledge to navigate the complexities of healthcare delivery in diverse settings.
We recognize that the problem of limited access to deep learning models is not limited to one specific geographic region. It impacts low- and middle-resource countries globally, as well as some institutions in high-income countries that face resource constraints. With our international team, we have the advantage of accessing diverse perspectives and data from usually unrepresented populations such as Brazil and Uganda.
EmbMed's approach to self-supervised learning ensures that models can learn meaningful representations from the data without the need for extensive labeled data, making it a cost-effective and resource-efficient solution. By providing vector embeddings, we empower institutions to conduct research and develop models tailored to their specific needs.
- Creating a versatile data framework that connects broadly disparate, multimodal data sets to identify patterns or insights to serve as hypotheses for improvements in health systems or global surveillance systems
- Other
- Concept: An idea for building a product, service, or business model that is being explored for implementation
- Financial (e.g. accounting practices, pitching to investors)
- Legal or Regulatory Matters
- Product / Service Distribution (e.g. delivery, logistics, expanding client base)
Our solution, EmbMed, brings innovation to the field of medical image analysis in a unique and transformative way. EmbMed addresses the problem of limited access to deep learning models for medical image analysis in low-resource settings, and it does so by leveraging cutting-edge data science techniques to create medical image vector embeddings.
What makes EmbMed innovative can be summarized in the following key points:
Universal Building Blocks: EmbMed employs self-supervised learning techniques to train foundational models on a diverse dataset collected from institutions worldwide. This approach allows us to create universal building blocks that serve as a foundation for institutions in different countries and regions. These building blocks can be customized to address specific healthcare challenges, making the solution highly adaptable and scalable.
Data Collection and Diversity: Our extensive network allows us to collect diverse medical image data from institutions across the globe. This diversity is crucial, as it ensures that the vector embeddings generated are representative of a wide range of patient populations and healthcare challenges, making them applicable in various contexts.
Self-Supervised Learning: EmbMed employs state-of-the-art self-supervised learning algorithms to train foundational models. Self-supervised learning is a cost-effective and resource-efficient approach, as it doesn't require extensive labeled data. This innovation allows us to overcome one of the major barriers to deploying deep learning models in resource-constrained settings.
Customization: Our solution empowers institutions to create specialized deep learning models using the vector embeddings generated by EmbMed. This customization is essential because healthcare solutions are not generalizable due to the vast differences in patient populations and healthcare challenges. EmbMed enables institutions to tailor the technology to their unique needs.
Equity and Inclusivity: By democratizing medical image analysis and research, EmbMed breaks down the barriers related to limited data, specialized expertise, and computational resources. This fosters equity and inclusivity in healthcare research by enabling institutions worldwide to conduct their research and address the unique healthcare challenges they face.
Global Perspective: Our international team, with representation from diverse regions, allows us to capture a global perspective on healthcare challenges. This unique advantage not only contributes to the diversity of our dataset but also ensures that the vector embeddings created are relevant and applicable to institutions worldwide.
EmbMed has the potential to catalyze broader positive impacts by setting a new standard in medical image analysis and research accessibility. It can change the market by promoting the adoption of self-supervised learning techniques and vector embeddings in the healthcare sector, making it a more equitable and inclusive field.
EmbMed can revolutionize healthcare research, mitigate disparities in healthcare access and outcomes, and make healthcare research truly equitable and inclusive on a global scale.
EmbMed is aligned with UN Sustainable Development Goal 3 for Good Health and Well-Being by addressing critical issues in healthcare access and outcomes. This project leverages cutting-edge data science techniques to create medical image vector embeddings, promoting equitable healthcare in low-resource settings. Here's how EmbMed contributes to achieving SDG 3:
Equitable Access to Healthcare Technologies: Deep learning models for medical image analysis are revolutionizing healthcare, but access to these technologies has been limited to privileged groups. EmbMed breaks down this barrier by creating universal medical image vector embeddings that can be used by institutions in low-resource settings, enabling them to develop their own specialized models. This fosters equitable access to advanced healthcare technologies.
Diverse Data Inclusion: Our approach involves combining highly heterogeneous and varied sources of data, allowing EmbBed to learn from a broader representation of real data that has been collected from diverse institutions across the globe, including low- and middle-income countries in Africa, North America, and South America. This ensures that the vector embeddings are representative of a wide range of patient populations, addressing the need for diverse and inclusive healthcare solutions.
Resource-Efficient Learning: EmbMed utilizes self-supervised learning techniques, which do not require extensive labeled data. This makes the creation of vector embeddings more cost-effective and resource-efficient, enabling institutions with limited resources to participate in medical research.
Empowering Local Expertise: By allowing institutions to create their own specialized models using the vector embeddings, EmbMed empowers local expertise to address healthcare challenges specific to their regions. This approach recognizes that healthcare solutions are not one-size-fits-all and must be tailored to local conditions.
Mitigating Disparities: The scarcity of local medical image data, specialized personnel, and computational resources has perpetuated health inequalities. EmbMed addresses these contributing factors by democratizing medical image analysis, thereby mitigating disparities in healthcare access and outcomes.
Global Collaboration: Our organization has a global network of experts and collaborates with institutions and researchers from various countries. This fosters global collaboration, knowledge sharing, and mutual support, reinforcing our commitment to addressing healthcare inequalities on a global scale.
EmbMed is a transformative solution that aligns with UN SDG 3 by addressing the challenges of limited access to healthcare technologies and the resulting disparities in healthcare outcomes. It empowers institutions in low-resource settings, promotes inclusivity in healthcare research, and contributes to the goal of ensuring good health and well-being for all, regardless of their geographic location or resource constraints.
EmbMed tackles the challenge of limited access to deep learning models for medical image analysis in low-resource settings. EmbMed leverages cutting-edge data science techniques to create medical image vector embeddings, transforming healthcare research and promoting equity in underserved regions.
AI Components: EmbMed relies on state-of-the-art self-supervised learning techniques to train foundational models for medical image analysis. These models are the core AI components that generate vector embeddings from medical images. Vector embeddings are compact numerical representations that capture the crucial information and features of medical images, making them suitable for machine learning algorithms. These embeddings condense image data into a structured and meaningful format, allowing for efficient image analysis and insight extraction.
Novel Data Sets: We focus on the acquisition of novel, proprietary data sets that include medical images from various institutions, especially those in low- and middle-resource countries. These data sets are an essential component of our solution as they underpin the development of robust foundational models, ensuring that the resulting vector embeddings are representative of the diverse populations and healthcare challenges encountered globally.
Data Curation Plan:Our data curation plan is multifaceted. We utilize our extensive network, which includes professionals and institutions from around the world, to collect medical image data. This data collection process is ongoing and continuously updated to ensure the most relevant and current data. Moreover, the use of self-supervised learning in our models reduces the reliance on extensive labeled data, making the data curation process more cost-effective and resource-efficient. We are committed to maintaining data integrity, protecting patient privacy, and adhering to ethical data usage standards.
EmbMed's approach of training foundational models using this diverse dataset allows institutions, regardless of their geographical location or resource constraints, to create specialized deep learning models. They can use their own images, specific to their patient populations, healthcare challenges, and research objectives, making the solution highly adaptable and customizable.
By providing scalable and adaptable AI technology alongside diverse, curated data sets, EmbMed is poised to democratize medical image analysis and research, breaking down barriers related to limited data, specialized expertise, and computational resources. This transformative solution empowers healthcare institutions worldwide to take control of their healthcare research, mitigating disparities in healthcare access and outcomes.
Our organization, MIT Critical Data, is deeply committed to ensuring the ethical and responsible use of AI in our project, EmbMed. We recognize the transformative potential of AI in healthcare but are equally cognizant of the ethical challenges that come with its application. We have put in place a comprehensive approach to address these issues.
Privacy and Security Concerns: We are acutely aware of the sensitive nature of medical data. To safeguard patient privacy, we strictly adhere to data protection regulations, such as HIPAA in the United States, and equivalent laws in other countries. The use of embeddings also secures the data sharing since it provides a de-identifiable, rich in information, lightweight version of the data.
Bias and Fairness: Bias in AI models can exacerbate existing healthcare disparities. We employ rigorous data collection and curation practices to minimize bias in our training data. Furthermore, we actively monitor and evaluate our models for fairness and bias using established techniques, making necessary adjustments when disparities are detected.
Ethical Considerations: The deployment of AI in healthcare demands a deep commitment to ethical principles. Our team, which includes clinical experts from diverse specialties and countries, actively engages in discussions on the ethical implications of AI in healthcare. We continually refine our models and processes to ensure alignment with ethical guidelines.
To further mitigate risks and ensure responsible use of AI, we actively involve the community in our solution:
Community Input in Solution Design: We have incorporated feedback from our network of international experts and institutions. This iterative process ensures that our solution remains grounded in the unique needs and contexts of different regions and healthcare settings.
Community Engagement: We actively engage local medical experts, researchers, and institutions in the development and deployment of EmbMed. Their insights, drawn from their deep understanding of local healthcare challenges, guide our model development and deployment strategies.
Transparency: We are committed to transparency in our work. We provide clear documentation of our methodologies, model architectures, and data sources. We also plan to openly share our findings and research outputs, allowing the broader scientific community to assess and validate our work.
Our approach to ethical and responsible AI is comprehensive, encompassing privacy, fairness, ethics, and community involvement. By closely adhering to these principles, we aim to ensure that EmbMed's deployment will have a positive impact on healthcare while minimizing potential risks. We are dedicated to not only addressing the challenges but also to actively engaging with the community to foster responsible AI innovation in healthcare.
Our impact goals for the next year and the next five years are centered around transforming healthcare research and ensuring equitable access to medical image analysis in low-resource settings. We aim to achieve the following:
Year 1 Impact Goals:
Develop and release the initial version of EmbMed's medical image vector embeddings for retina images using the Brazil and Uganda dataset.
Host a minimum of two collaborative datathons in Brazil and Uganda using EmbMed to promote the use for research and contact with local institutions and experts.
Foster community engagement and promote the use of vector embeddings in research by publishing the framework for retina image embedding extraction and by presenting the EmbMed in internations events such as datathons and conferences.
Year 5 Impact Goals:
Expand EmbMed's reach to include partnerships with over 10 healthcare institutions worldwide, spanning also to other countries in other unrepresented regions in South America, Africa, and Asia.
Contribute to the development of a global network of medical image vector embeddings, covering a broad spectrum of healthcare needs and populations.
Host annual international conferences on equitable healthcare research, bringing together experts and institutions from around the world.
Collaborate with international organizations to set up data-sharing agreements, ensuring accessibility to a wide array of medical image data.
How We Will Achieve These Impact Goals:
Initial Development of Vector Embeddings: Allocate resources and expertise to create and validate the retina foundational models for vector embeddings using Brazilian and Uganda data during year 1.
Partnerships with Healthcare Institutions: Leverage the extensive network of MIT Critical Data and our team's global presence to establish partnerships with institutions in low-resource settings in Continents like Africa, South America and Uganda. Provide technical and logistical support to help these institutions integrate EmbMed into their research processes.
Community Engagement and Datathons: Continue to organize datathons, engaging with more institutions and researchers, promoting knowledge sharing and collaborative research efforts.
Customization for Local Needs: Empower healthcare institutions to customize their deep learning models using vector embeddings, allowing them to address unique patient populations and healthcare challenges. Provide training and support to ensure institutions can utilize the technology effectively.
Global Expansion and Data Sharing: Expand the reach of EmbMed by establishing partnerships with healthcare institutions in a variety of regions. Collaborate with international organizations and governmental bodies to facilitate the sharing of diverse medical image datasets, ensuring the inclusivity of healthcare research worldwide.
Annual Conferences: Host international conferences in the datathons organized to facilitate collaboration, knowledge exchange, and advancements in equitable healthcare research. These conferences will serve as a platform to showcase the impact of EmbMed and foster a global community dedicated to addressing healthcare disparities.
- Nonprofit
We are a group of researchers from different institutions. We have 8 data scientists and engineers and 3 Medical Doctors from different institutions and different countries. All the data scientists in our team are working part-time on our solution, and all the Doctors are consulting the team once a week.
We have been actively working on our solution, EmbMed, for approximately 5 months, during which time we have made significant progress in data collection and some tests of the self-supervised techniques for our EmbMed approach by reviewing, and testing current approaches. Our journey began as a small group of dedicated researchers, and we are now poised to expand our efforts to address critical healthcare disparities in low-resource settings.
Our approach to incorporating diversity, equity, and inclusivity into our work is central to the mission and values of EmbMed and MIT Critical Data. We recognize that healthcare solutions must be tailored to diverse populations, and equity is a fundamental principle in our work. Here are the key components of our approach:
Diverse Leadership Team: Our leadership team reflects a global and multidisciplinary composition. We have technical and clinical experts from different countries and specialties. This diversity in leadership brings a variety of perspectives, experiences, and cultural insights, which are invaluable in addressing healthcare disparities.
Team Goals for Diversity and Inclusion: Our team is committed to enhancing diversity and inclusion. We understand that diversity fosters innovation and ensures that our solutions are more equitable and relevant. We are actively working to expand our team's diversity by recruiting members from underrepresented backgrounds.
Proximity to the Target Population: Our global team embodies the principle of proximity to the target population. We are committed to understanding the local challenges and healthcare disparities in various countries. By engaging with diverse communities through events like datathons, we foster strong connections and collaborations that lead to more inclusive and region-specific solutions.
Community Engagement: Inclusivity is not just a theoretical concept for us. We actively engage with medical experts and institutions from diverse backgrounds and countries. Their input is invaluable in shaping our solutions to address the unique challenges they face.
Community Input in Solution Design: We have a network of community members who provide us with invaluable insights into the difficulties they encounter in developing healthcare solutions. This community input directly informs the design of our solutions to ensure they are more inclusive and applicable to a wider range of institutions and regions.
Our operational model and plan for the EmbMed addresses the critical issue of limited access to deep learning models for medical image analysis in low-resource settings. The following sections outline our strategy and execution plan:
Team Expertise:
Our team consists of a group of global and local experts from different countries, comprising technical and clinical experts with a strong track record of developing projects related to the creation of vector embeddings for various types of images. This expertise ensures that we have the knowledge and experience necessary to execute this project effectively.
Proximity to the Target Population:
To better understand the unique challenges faced by different communities and healthcare institutions, our team is a global and diverse group with members from around the world. We regularly organize datathons in over 50 countries, fostering collaboration and strengthening relationships with various institutions and researchers. This approach ensures that our solutions are not developed in isolation but are deeply connected to the needs and contexts of the target populations.
Team's Representation:
Our Team Leader, Luis Nakayama, is an experienced researcher and ophthalmologist from Brazil and the creator of the Retina Brazilian Dataset BRSET. In our team we also have medical experts and data scientists from different countries and specialties with experience and commitment to reducing health inequities. This diversity in representation ensures that our solution is well-informed and adaptable to various environments.
Community Engagement:
Our team actively engages with the medical community in different countries, allowing us to leverage their expertise and collaborate on the development and deployment of our solution in diverse healthcare environments.
Community Input in Solution Design:
Through our global network, we have received valuable insights into the difficulties faced by institutions and healthcare providers in developing solutions for medical image analysis. This feedback has directly influenced our solution design and implementation.
The solution includes the following steps:
Data Collection: We gather retina image data from institutions in Brasil and Uganda, ensuring a wide range of datasets and perspectives.
Self-Supervised Learning: EmbMed employs state-of-the-art self-supervised learning algorithms to train foundational models on this diverse dataset.
Vector Embeddings: The trained models generate vector embeddings for medical images, capturing essential features and patterns within the images.
Customization: Institutions can use these vector embeddings to create specialized deep learning models tailored to their unique patient populations and healthcare challenges.
EmbMed is a scalable and adaptable solution that democratizes medical image analysis and research. It empowers healthcare institutions worldwide to take control of their healthcare research, mitigating disparities in healthcare access and outcomes.
To ensure the financial sustainability of our research project, EmbMed, we have devised a multifaceted approach that leverages our unique positioning, global network, and innovative solutions. Our primary goal is to cover our expected expenses and ensure the continuous growth and impact of our project. Here's how we plan to secure funding:
Grants and Donations: We will actively seek grants from both governmental and non-governmental organizations that support healthcare research and technology innovation. Given our affiliation with the Massachusetts Institute of Technology and our commitment to addressing a critical healthcare issue, we believe we are well-positioned to secure grants from various sources.
Corporate and Private Donors: We will reach out to corporations and individuals who are passionate about healthcare equity and the potential of our project to make a meaningful impact. Building strategic partnerships with organizations aligned with our mission will be a priority.
Research Collaborations: As a part of the MIT Critical Data research group, we have strong connections with global institutions and experts. Collaborative research projects can bring in funding and resources. We will actively seek opportunities for such partnerships to fund specific research initiatives.
Service Contracts: We will explore opportunities to provide our expertise and services to governments, healthcare institutions, and organizations in need of medical image analysis solutions. By offering customization and consultation services, we can generate revenue while also fulfilling our mission.
Fundraising Events and Datathons: We will continue to organize datathons and fundraising events, leveraging our global network and expertise to attract participants and sponsors. These events can generate revenue and help us maintain strong connections with our collaborators.
By diversifying our revenue streams and leveraging our global network, we aim to ensure that EmbMed becomes financially sustainable in the long term. Our commitment to addressing the critical issue of healthcare inequality through innovative medical image vector embeddings positions us for success in securing the necessary funding to drive our mission forward.
As of the present moment, MIT Critical Data's "EmbMed" project is operating on a minimal budget. The majority of the team members, including data scientists and engineers, are currently dedicating their time and expertise to this project on a voluntary basis. We have received an initial grant of $100,000 from the Oracle for Research program, which has been primarily utilized for computational resources and data storage. Therefore, our current annual operating costs are relatively low, primarily covering essential expenses such as cloud computing services, data acquisition, and minimal administrative overhead.
Projected Operating Costs for the Next Year:
Our projected operating costs for the next year will increase as we seek to expand and sustain the EmbMed project. We anticipate the need for funding in the following areas:
Human Capital: As we continue to grow the project, we intend to offer stipends or part-time salaries to our team members, especially the data scientists and engineers who are currently contributing their time voluntarily. The team's expertise is crucial to the success of the project, and we aim to ensure their commitment by providing fair compensation.
Computational Resources: The current grant from Oracle for Research is essential, but it is finite. We will require ongoing funding to maintain access to the computational power required for training and developing vector embeddings from the increasing volume of medical images.
Administrative and Operational Expenses: There will be administrative overhead related to project management, outreach, and collaboration with partner institutions. These costs may include software licenses, legal services, and general operating expenses.
Community Engagement and Events: To maintain our global reach and engagement with different communities, we will continue to organize datathons and collaborative events. These activities help strengthen our network and advance the project. Funding will be necessary to support event logistics and travel expenses for team members and collaborators.
In summary, our projected annual operating costs for the next year are estimated to be 200.000 USD. This budget will enable us to ensure fair compensation for our team members, expand our dataset, maintain computational resources, cover administrative costs, and sustain community engagement efforts. With this budget, we are confident that we can advance the EmbMed project and work toward equitable healthcare solutions in low-resource settings.
The Cure Residency opportunity will play a pivotal role in accelerating the development and impact of EmbMed.
Seed Funding: The seed funding provided by the Cure Residency will be invaluable in advancing our project. It will enable us to invest in computational resources, and support our team of dedicated researchers who are currently working on this project voluntarily. This financial support will also help us scale our efforts and reach more healthcare institutions in need.
Mentorship: Mentorship is a crucial element for the success of the project. We believe that the guidance and expertise of mentors associated with the Cure Residency will provide valuable insights mainly in areas such as communication with the Industria, where we as researchers have not that much experience.
Lab Space: Access to lab space is particularly exciting for our team. It will enable us to carry out experiments, test our models, and collaborate with other researchers in a dedicated and well-equipped environment.
In 2024, we are seeking $100,000 in funding to further our research project, "EmbMed." This selection is based on a careful consideration of the critical needs and expenses required to achieve our objectives. The funding will be allocated as follows:
GPU Usage for Model Training and evaluation: 40% will be allocated to the ongoing costs associated with GPU usage for training our models. Deep learning models require significant computational resources for continuous training and optimization. Also 20% of that 40% will be dedicated to data preprocessing and storage infrastructure. This includes data curation, cleaning, and organization, ensuring that the medical image data we collect is ready for model training and analysis.
Dissemination and Publication: 15% will support the dissemination of our research findings through conferences, academic papers, and participation in datathons. This funding will help us share our results and insights with the global research community.
Staff stipends: 30% of the funding will be allocated to compensating our diverse team of global and local experts, including technical and clinical professionals from different countries. These experts will be crucial in driving the success of our project, "EmbMed." By providing stipends, we aim to retain and further engage their specialized knowledge and expertise in developing medical image vector embeddings for equitable healthcare solutions in low-resource settings.
Contingencies: The remaining 5% of the funding will be earmarked for unforeseen contingencies that may arise during the course of our research project. This contingency fund will help us address unexpected challenges or seize unforeseen opportunities, ensuring the smooth progress and adaptability of our project. It will serve as a safety net to mitigate any potential risks that could affect the successful execution of EmbMed.
The Cure Residency opportunity will play a pivotal role in accelerating the development and impact of EmbMed.
Seed Funding: The seed funding provided by the Cure Residency will be invaluable in advancing our project. It will enable us to invest in computational resources and support our team of dedicated researchers who are currently working on this project voluntarily. This financial support will also help us scale our efforts and reach more healthcare institutions in need.
Mentorship: Mentorship is a crucial element for the success of the project. We believe that the guidance and expertise of mentors associated with the Cure Residency will provide valuable insights mainly in areas such as communication with the Industria, where we as researchers have not that much experience.
Lab Space: Access to lab space is particularly exciting for our team. It will enable us to carry out experiments, test our models, and collaborate with other researchers in a dedicated and well-equipped environment.
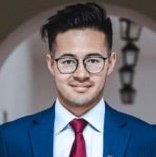
AI Engineer
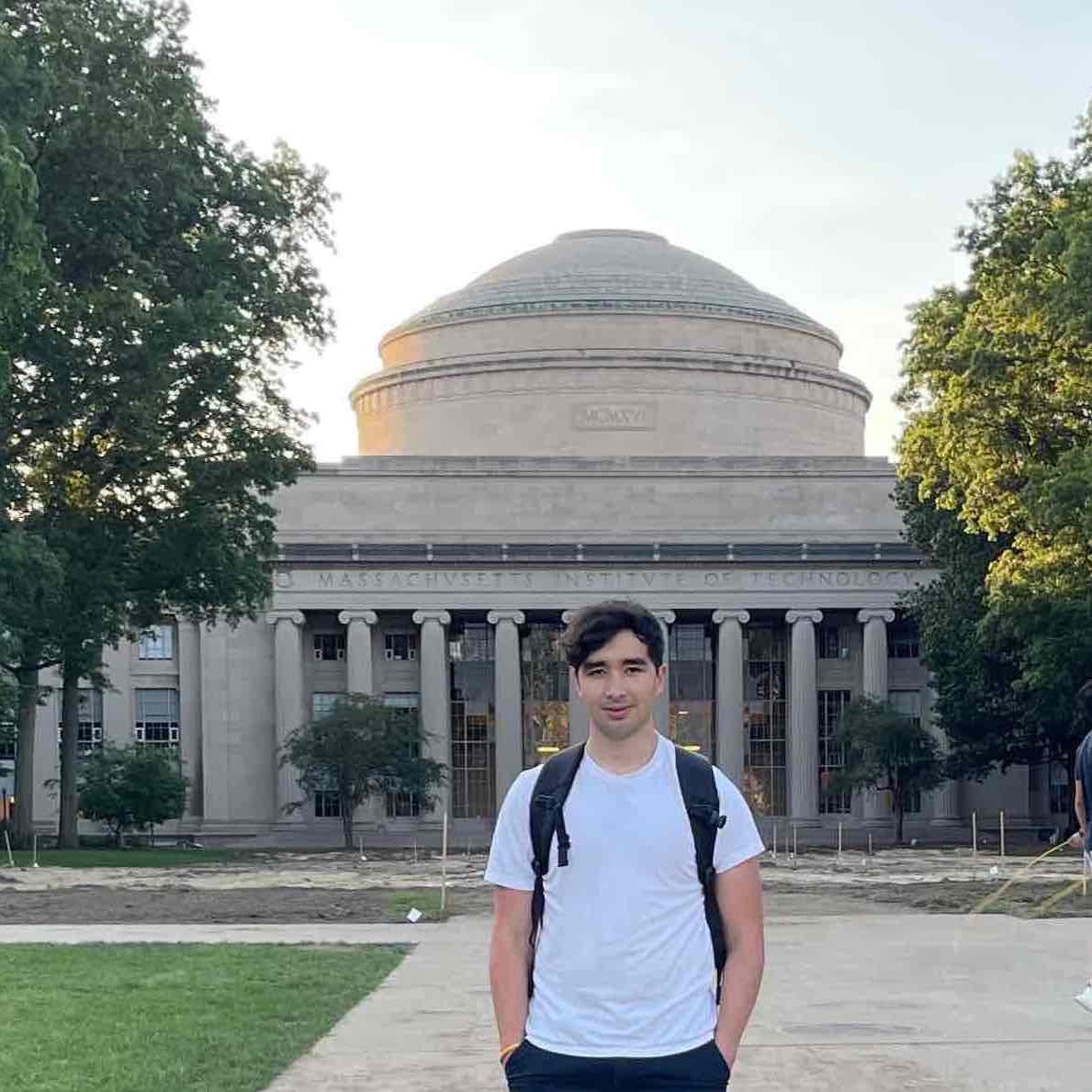
Data Scientist, Researcher