Blue AI
Every year in Brazil, $5.4 million is expected to be spent on fraud and misuse of health insurance, and another extra $5 million on preventable private hospitalizations for chronic diseases. This makes insurance costs the second largest expenditure for employers, just after employee payroll, and they continue to rise above inflation rates each year. With constant claims is even more challenging to handle misuse and be precise on preventable care.
So, what if employers' HR teams and healthcare providers could have software that is equivalent to a senior data science team providing actionable information? That’s what Blue AI solves.
Blue AI offers a platform that uses pre-trained ML (XGBoost and NLP mainly) to catch avoidable healthcare outcomes from insurance claims data, helping HR teams and healthcare providers save employers in LATAM up to 30% on healthcare costs. Our Beta initially detects fraud and misuse, reducing erroneous reimbursements, and predicts members' preventable hospitalizations for chronic diseases, pointing out those that will face complications and might go to the hospital up to 12 months earlier.
HR and Healthcare Managers are using Blue AI by dragging and dropping claims files and selecting our ready-to-use ML models, without coding or statistical expertise. We envision creating a marketplace of recommended healthcare providers for at-risk employees.
1) Who we envision to serve: Latino population struggling to afford care.
Description: Regrettably, 80% of all Brazilians (150 million people) don’t have access to health insurance, primarily due to high prices. At the whole Latin America, individuals without health insurance (expected to be something close to 300 million people) are often forced to contend with overcrowded public hospitals and limited resources. Blue AI is being built to address those to more affordable higher-value care in the long term, by suggesting the right treatment at the right time, preventing complications that could overwhelm public hospitals.
To do so, we build ML to navigate healthcare data and generate accurate predictions. Blue AI initially provides a no-code AI platform that works with insurance claims data, as a strategy to get its first users demanding recommended care. From that, we envision creating a marketplace of personalized providers that best fit members at risk, even those who can’t afford health insurance.
2) Initial users: HR and Healthcare managers.
Description: In Latam is expected to have 4.2M employers struggling to provide health insurance as a benefit for its employees. Nowadays, all they have to manage the company’s second most expensive expenditure are dashboards with many fuzzy graphs of past analysis. We also understand that health insurance claims data are seen as a "black box", hard to manage and decode. With constant claims from hundreds of employees, it is tough to prevent fraud and challenging to handle misuse. But, with machine learning preventing accurately is more efficient than just being reactive and investing only in those that already had high costs in the past.
Pedro has been building AI applications to predict chronic diseases since his grandparents struggled with cancer treatment for not having access to health insurance, 5 years ago. In order to engage, communicate, and solve real healthcare and economics friction problems Pedro first committed himself to provide free AI applications, including a prediction app for diabetes that was awarded at the Brazil Conference of 2019 at Harvard and MIT, from behavioral data. After various free AI apps, in 2021 employers, insurance brokers, and health plans emerged with the demand of accessing predictions of chronic hospital admissions from insurance claims data.
From September 2021 to April 2023, we've been collecting insurance claims data and validating preventable hospitalization prediction models by not charging anything for our predictions to any user. Today we have over 600 thousand employees' insurance claims data, and over 300 thousand of them we have 3 years of historical data. 6 months later, we got over 16 thousand paid members.
On the other hand, Ronaldo has been a data scientist since 2017, working with recommendation systems and classification models, similar approaches that Pedro was using on its first predictions for hospital admissions. Ronaldo got his Physics Ph.D. for conducting an algorithm to improve efficiency during phase transactions at the Federal University of Minas Gerais, the highest-ranked university in the state.
- Collecting, analyzing, curating, and making sense of big data to ensure high-quality inputs, outputs, and insights.
- Creating models and systems that process massive data sets to identify specific targets for precision drugs and treatments.
- Pilot: An organization testing a product, service, or business model with a small number of users
- Business Model (e.g. product-market fit, strategy & development)
- Financial (e.g. accounting practices, pitching to investors)
- Public Relations (e.g. branding/marketing strategy, social and global media)
How easy it is to use AI with no code on our platform.
We understand that health insurance claims data are seen as a "black box", hard to manage and decode. But, with machine learning preventing accurately is more efficient than just being reactive and investing only in those that already had high costs in the past.
With constant claims from hundreds of employees, it is tough to prevent fraud and challenging to handle misuse. Making AI easy to use for predicting avoidable healthcare outcomes and for auditing misuse is guiding our users to:
- Invest accurately in healthcare providers and primary care that best fits members at risk;
- Audit misused healthcare resources, such as duplicated procedures and erroneous reimbursements;
- Negotiate better terms with insurance providers based on predicted outcomes that can be prevented.
Based on the data collected so far, our beta version has generated predictions for preventable hospitalizations with up to 6 times more precision compared to the traditional reactive approach of seeking primary care only after members had high costs in the past, which is the common current practice.
Having said that, we've been ensuring healthy lives and promoting well-being for all by providing a tool that is accessible to get healthcare predictability and take preventable actions before expensive healthcare bills due to avoidable and chronic complications come.
About our training dataset: We've been collecting insurance claims data for more than a year, by not charging anything for our predictions. Today we have over 600 thousand employees' insurance claims data, and over 300 thousand of them we have 3 years of historical data.
From past insurance claims data, Blue AI uses machine learning to deliver actionable insights including:
1) Total Healthcare Expense Prediction: We use a time series approach with Facebook's Prophet algorithm, analyzing 24 months of past data to predict the next 12 months' healthcare expenses with an accuracy of 87%.
2) Avoidable Hospitalization Prediction: We employ XGBoost, using procedure and cost data, to predict hospitalizations 12 months in advance. The model provides the probability of each employee, older than 20 years old and with at least 15 procedures performed in the past, being hospitalized in the next 12 months (class 1). On average, our model gets to predict correctly 6 out of 10 employees that will be hospitalized, this is our recall. The precision for the class 1 of this beta version is 63%.
3) Fraud Detection: Our NLP model, based on Latent Semantic Analysis (LSA), identifies non-standard procedure descriptions, helping detect fraud and misuse in claims data. At least 10% of the claims each company shares do not follow any standard procedures terminology. We have been able to track almost 60% of non-identified procedures on our database until now.
Scientific Validation: Our approach is validated against clinical data in the paper 'Algorithmic Prediction of Health-Care Costs' by MIT CSAIL, demonstrating the effectiveness of the use of claims data for our prediction model: https://people-csail-mit-edu.ezproxyberklee.flo.org/gjw/papers/healthcare.pdf
We only get anonymized data or after the member has signed a term of use expressing the consensus for specific tasks.
Our main risk is the case where part of that data turns public. But, by far, over 90% of the data we have is anonymous, and 100% of the data that is being uploaded to our platform is already anonymized by the user previously. For now, we plan to keep it that way in order to secure privacy and once that doesn't affect our user experience.
For next year we plan to:
1) Automate the data upload process. Today we're doing things that don't scale first so we still ask for a few days to update the results on our platform after the user drops a new data file.
2) Deploy a diabetes ML classifier model. This is one of the main chronic conditions leading causes of preventable hospitalizations.
3) Deploy a spine complications ML classifier model. Another one of the main chronic conditions leading causes of preventable hospitalizations.
4) Reach 50 healthcare providers: to use as an acquisition pattern to distribute our platform faster.
5) Reach 1000 employers as recurrent paying customers.
For 5 years from now, we plan to:
1) Provide predictions for 10 million Brazilians.
2) Build a marketplace of recommended healthcare providers based on our AI.
3) Scale our solution to provide our valuable information to others who can’t afford health insurance today.
- For-profit, including B-Corp or similar models
2 people, Ronaldo and Pedro. Both of them full-time.
Pedro since September of 2021. Ronaldo since January of 2023.
On our vesting contract, we have a clause in which we are both committed to tackling Racial, Gender, Social, and Healthcare Access Inequality. We are just starting, but we seak this same value from others that might join our founding team next.
We use insurance brokers and healthcare providers as channel partners to reach employers faster. Because of it, we got all of our paying customers today.
Our first users and channel partners we got by asking for referrals and talking to them in person. We did not run any ads so far, but still:
1) We were featured at a 2M+ LinkedIn followers media portal by not paying anything as well, you can find the link below.
2) Pedro has recently been named one of the entrepreneurs at MIT Innovators Under 35 in Brazil. We know this is only PR, but it has also increased our visibility at the moment.
We've been also looking forward to building a tech community of HR and Healthcare managers on Slack, so we can share updates on our platform and make potential users even more engaged on our journey.
Today, we charge on average $129 per employer, per month. Latam is expected to have 4.2 M employers with at least 50 employees, the ones that usually pay health insurance as a corporate benefit for its employees.
$129 monthly subscription x 4.2M employers x 12 months = $6.5 billion dollars
Today we spend 400 dollars in COGS for every 2K dollars in revenue. But that can be scalable and the difference is expected to drop in order to provide a gross margin of 10%.
By now, the team is composed of me and Ronaldo. Besides we do work full-time, we do not take any salary or other compensation out of Blue AI yet. We plan on bringing 6-8 senior software engineers and data scientists to build our founding team, offering either payroll + stock option.
The salary of a senior full-time engineer/data scientist is approximately 7K dollars per month, in Brazil, based on what we got before Blue AI.
We are looking to raise a 700K dollar pre-seed by the end of the year.
(6 new founding team members + 2 co-founders) x 7K monthly payroll = 672K dollars yearly.
In addition to that we plan on raising more funds to invest in acquisition strategies. But that looks like a seed round ask.
Sharing experiences and learning about business models applied to AI platforms in healthcare is one helpful thing to do.
Secondly, improving skills in programming and AI algorithms is another topic that gives us butterflies about being part of the residency. We hope we can learn and practice new technologies to improve our product and decrease our learning curve in building new AI models.
Last but not least, connecting with new entrepreneurs or potential engineers looking to build a company together is one thing that would help us build a strong and diverse founding team.
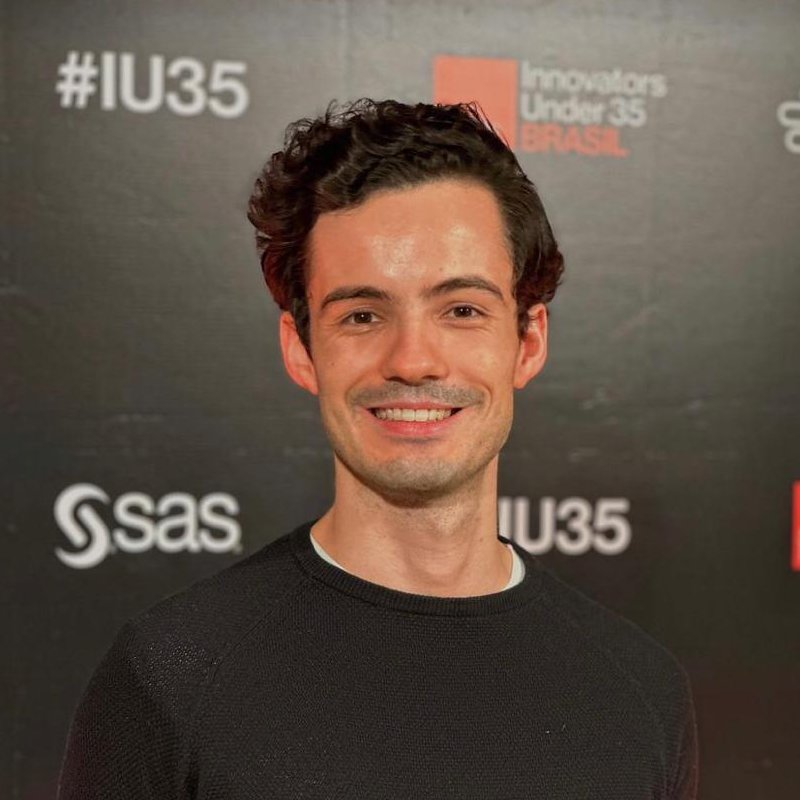
Founder