Patient AI Care Navigator
A few years ago, my grandmother was admitted to the hospital for the second time in as many weeks. She missed a couple of important follow-up appointments and had an adverse reaction to medication she was not supposed to take at home. Neither she nor her primary caretakers, my mom and I, were equipped with the health knowledge to fully comprehend her discharge instructions. About 27% and 30% of Medicare and Medicaid patients respectively have below basic health literacy. Low health literacy results in poorer health outcomes from factors such as low medication adherence, fragmented care transitions, and miscommunication of care plans. According to The Literacy Project, the average American reads at a seventh grade level. This level of literacy is even lower for underserved communities and vulnerable populations. The results of poor health literacy are exacerbated for these patient populations because many of these patients come from marginalized demographics, lower levels of education, different cultural dynamics, and may hold certain stigmas about healthcare. Low health literacy also leads to a lower likelihood to seek out preventative care and can be attributed to higher utilization of emergency rooms and higher rates of hospitalization. All of this results in compounding stress at every point of care for these patient populations, leading to poorer health outcomes and increased economic burdens. Higher rates of readmissions and emergency department visits has had extremely negative financial effects on health systems and patient populations across the country. Higher health literacy could save Medicare up to $25.4 billion annually, according to UnitedHealth Group research. Patients now have ever-increasing access to their medical data, commercial digital biometrics, and virtual resources but the influx in availability of medical information has not addressed the root of the problems with health literacy. The key to tackling low health literacy is providing patients personalized access to their medical information and health data in the mode that they are most comfortable with.
We are working on an idea to build the first virtual healthcare assistant for patients that is actually personalized for their level of health literacy. We plan to use structured data that can be pulled from integrations with patients’ medical records along with AI document processing to convert unstructured data from clinical documents, such as discharge instructions, into structured data that can be used to paint a portrait of a patient’s care journey. Patients interact with their data using natural language processing in a generative AI system that is built on a language chain leveraging a large language foundational model that is pre-trained on clinical data. We can utilize many different characteristics and demographic information about the patients to automatically generate prompts that will yield outputs that are safe and comprehensible for patients. These same attributes will be used for ongoing prompt engineering to get patients faster access to the information they need. Personalized care management powered by generative AI such as formulation of medication plans, creating care plans, interpreting lab results, and directing to patient educational materials will all be at their fingertips in a single central hub. We want the interface to be a dynamic layout that adapts to how patients interact with the application by highlighting the most important information to them based on their medical conditions and level of health literacy. Further integrations with insurance benefits and provider directory data will be used with our virtual healthcare assistant will allow patients to get better explanations on what healthcare services are available to them. We also want to set a new standard for measuring health literacy in the age of through surveys in our application that will help us gather data to continue to inform how we can help patients engage with their medical data in more meaningful ways. Patients will be able to share their conversational data, medical data, and health literacy data with members in their circle of trust as well as their providers directly. This way, there is full transparency for their care teams to know how the patients are managing their own care and interacting with their medical information. This will allow for more productive conversations between providers and patients at each point of care that will minimize miscommunication of health information.
The issue of low health literacy disproportionately impacts vulnerable patient populations such as older patients and patients from lower socioeconomic and minority backgrounds. These patients tend to live in low-income urban and rural communities with healthcare systems that are under financial and human resource constraints. Overall, these communities have fewer health care resources such as health literacy education or health awareness available for their patients. These patients are also typically insured by Medicaid or Medicare and there is lower access to elective treatments and lower reimbursement rates mean that they may not be accepted by certain providers or practices. These populations inherently have lower access to quality care and tying that with higher likelihood of low health literacy can prevent them from finding treatment or lead to mismanagement of their healthcare needs. This can lead to exacerbation of more severe health issues and this is a continuous negative cycle. Growing up, my family qualified for MassHealth coverage and they still are. My sister, who herself is a college graduate but still under 25, recently had some health concerns that led to a few specialist visits and she had to get a series of lab work done at a local teaching hospital. She could not get a clinician on the other side of the phone to answer her questions about her lab results for weeks. Veterans are another vulnerable population where low health literacy has a significant impact. A VA study conducted at VHA facilities found that 55% of veterans receiving care at those facilities have lower than adequate health literacy skills. Even within the veteran populations, minorities are more likely to have lower health literacy than their white counterparts. Veterans also have higher likelihood for mental health concerns and are less likely to seek out behavioral treatments or preventative treatments for other non-behavioral conditions. Our solution is in a unique position to address many of the underlying causes of low health literacy. We can centralize all of their medical data, from their recent admission or their history of visits to their insurance benefits to learning about their medications and their conditions, in a structured manner that patients can interact with in a simple interface. Patients can have conversations about their medical information and share these learnings with their caregivers or their care providers. These conversations can be tailored towards their level of health literacy or in their native language and the virtual healthcare assistant can adapt as it learns more about the patient. A continuous feedback loop on how a patient wants to view or visualize their data will allow for more meaningful outputs as they interact with our solution. By meeting patients where they are with their own vernacular, our solution can eliminate the confusion and miscommunication across each point of a patient’s care journey.
We have a team with very diverse backgrounds that have close connections with these underserved patient populations. I am a first generation Chinese American who grew up in a low-income immigrant family so I have experienced firsthand the inequitable access to healthcare and have been impacted by the stigmas that my parents hold against Western medicine due to their lack of education and low health literacy. I can get in front of a low-income Chinese immigrant population who will provide valuable input to help guide the development of our solution. We have members of our team who are immigrants from Egypt and India who have also had their own challenges with accessing healthcare in the United States within their own respective communities. One of our team members is a clinician in the VA healthcare system so she has worked and gotten to know many veterans. We will be very close to this population since we were recently accepted into VA Innovator Network’s Spark-Seed-Spread accelerator for this upcoming year to get us in front of real veterans to validate our idea and follow human-centered design principles as we begin to build our prototype. We will be conducting user research to understand their experiences with low health literacy and how our idea might improve the health literacy levels for these veterans. Another key stakeholder within this population would be veteran caretakers and we want to explore how this same tool may be adapted to empower them to manage their veterans’ care better. We will be iterating on our prototype using this feedback to build an experience that will address their needs in a safe and patient-centered way. After building our prototype, we plan to put this solution in front of these veterans to measure impact and continue gathering insights on how to improve our solution. That being said, we want this to be scalable to patient populations across many demographics and we want more connections that will help us get diverse perspectives on what our solution may need to meet every patient’s needs. We are looking for resources and different avenues that can get us access to more patient populations and more user groups. This way, we can build a solution that can demonstrate positive, measurable outcomes tackling low health literacy for all patients.
- Augmenting and assisting human caregivers.
- Creating user-friendly interfaces to improve communication between experts and patients, including providing better information, results, and reminders.
- Concept: An idea for building a product, service, or business model that is being explored for implementation
- Business Model (e.g. product-market fit, strategy & development)
- Financial (e.g. accounting practices, pitching to investors)
- Human Capital (e.g. sourcing talent, board development)
- Monitoring & Evaluation (e.g. collecting/using data, measuring impact)
- Technology (e.g. software or hardware, web development/design)
No solution currently out in the market is utilizing or developing generative AI tools that engage patients in a way that tackles the problems stemming from poor health literacy. Most patient engagement tools that are currently in the market are limited to patient scheduling, appointment reminders, and provider text messaging. These products are built to optimize the workflow for providers to connect with their patients and there remain a lot of issues with appointment no shows, provider miscommunication, and poor adherence to plans of care. These applications tend to be difficult to navigate especially for older patients. These applications can contain a lot of patient data, which is already hard to digest, and are often fragmented into unintuitive areas of the application. This data can be difficult to locate and hard to understand the complete picture of what is going on for the patient. What we are doing is reimagining how patients can access their health data in a platform that adapts to how they want to interact with their information. Current solutions in the healthcare generative AI market are primarily focused on optimizing provider workflows such as summarizing patient charts, provider conversation summarization, and providing generative search tools for providers but no one has yet attempted to improve patient access to their own data by leveraging large language models. Our solution will be at the forefront in adopting use of generative AI that is patient-centered rather than provider-centered. It will change the market by forcing other companies to take a closer look at how they can use AI to build a patient-centered experience. I envision this as a new form of personalized medicine that addresses the consumption of health information and driving positive patient outcomes by empowering patients who are now armed with increased knowledge about their health and the steps they need to take to take care of it. We will set a new standard to how generative AI tools can be patient facing and revolutionize the way that all market players address communication of health data to their patients.
Health literacy is a healthcare equity problem for communities and patients everywhere. Improving health literacy and making health information more accessible can help improve outcomes for the key indicators for SDG 3. Our solution could improve the maternal mortality ratio by providing an informational tool for women to learn about the risk factors for pregnancy complications that they might have. Tying together integrations with patient educational materials and ongoing monitoring of risk factors in our application could improve a patient’s ability to detect early signs and seek out treatment for these complications sooner. Our virtual healthcare assistant can also contribute to improving the under-5 mortality rate by providing parents with easy to understand information about vaccines, nutrition and hygiene practices. Our application will be able to tie in their children’s charts to allow direct access to their health data as well as directing them to online resources to find vaccine appointments, such as the CDC’s Vaccine Finder, using plugins for our conversational model so that patients can access those resources without having to leave the application. Armed with our application which will improve understanding of their clinical information and care plans, patients will be able to manage their own care better. This will help reduce the prevalence of non-communicable diseases and mental health disorders because patients are more informed and will seek out preventative or behavioral treatment sooner. In the application, we would be able to connect them to their providers by initiating phone calls or virtual visits and integrate with provider directories as a plugin for our virtual healthcare assistant so that it will be able to search and schedule with specialists. Our application will improve patient access to healthcare services by providing a centralized hub that will allow them to manage all of their healthcare needs. The ability for our application to simplify medical jargon communicated by providers may also decrease risk for medical errors and miscommunication of health information. Clinicians burnout will be reduced with patients that are empowered with their own care management and actually adhere to their care instructions because clinicians can now trust that their patients are continuing to receive the support they need when they are not in the hospital or doctor’s offices. Reducing clinician burnout is key in improving development and retention of the health workforce.
A core component of our product will be our virtual healthcare assistant that will use natural language generation that will allow patients to retrieve various pieces of their data in a conversational model. We plan to build a language chain on top of a foundational large language model such as a GPT that will be fine-tuned on datasets such as MIMIC clinical notes in order to process and generate clinical text. Next we will also fine tune the model with HL7 FHIR datasets in order to allow the model to parse and process FHIR data. We plan to use prompt tuning as a more efficient way to guide our model towards specific tasks. We will be gathering a large dataset of prompt data that we would train another smaller AI model to generate soft prompts. This data would include an extensive number of prompts based on multiple factors such as the patient’s educational level, preferred language, and other demographic information. This level of prompt tuning will allow our application to generate soft prompts to be passed down to our fine-tuned foundational model. My team will compile this prompt tuning data through extensive research on patients from various populations. To start, this will come from the veteran population and we plan to seek out a diverse sub-populations within that group to gather that data. We also plan to gather data from patient populations with various other demographic groups. We will build plugins for our foundational model to query any structured data through FHIR integrations directly within a patient’s chart. There may also be unstructured data such as clinical documentation such as discharge instructions or progress notes. We can grab the text from these PDF documents to pass down to pre-built tools such as AWS Comprehend Medical that would be able to detect medical concepts that we store at the patient level that can later be called upon by our model. Through a combination of pre-built datasets and novel data, we will be able to develop a virtual healthcare assistant never seen before in the consumer market.
Putting the generative AI tools directly in patient hands will involve a lot of scrutiny and risk. A big risk is the patient reception and trust in our solution because if we lose that trust, then we fail in making a positive impact for our users. This means we have to take every measure to validate the clinical accuracy of conversations or other outputs from the AI model underlying our solution. Physician members of our team will play a huge responsibility in conducting this clinical validation. As patients are using our application, we will ensure the necessary safeguards are in place to pull in provider intervention when needed and provide proper messaging to prevent misconstruing our solution as a diagnostic tool. There is potential liability that we will need to protect ourselves against in ensuring we surface adequate disclaimers, terms of service, or end user licensing agreements. As we partner with health systems to provide this tool for their patients, we will need to ensure proper contractual agreements are in place such that our liability is minimized. As we are developing and training our AI models, we will abide by the responsible AI principles set forth by the OECD. One of the potential risks will be potential interpretability issues of potential reasoning when using prompt tuning to generate soft prompts. We will need to ensure transparency at each layer within our AI system. Another risk is the introduction of unjust biases into our AI system during its development and we will work to address this by ensuring proper safeguards and ethical training of our models. Privacy and security will also be at the forefront of our responsibilities since they are entrusting us with sensitive data as well as preventing any penetration from our essential infrastructure. We will complete all due diligence when selecting third party vendors for core services such as server hosting, user authentication and authorization, and any integration with external services. Ultimately, we will seek the highest accreditations in industry standards for regulatory compliance such as HITRUST CSF and SOC II Type 2 certifications. We will abide by all HIPAA regulations when allowing patients to access their health data and address any potential risks to be considered in order to allow these patients to share data from our application securely with their providers or loved ones.
Over the next year, we seek to have an early prototype that we can put in front of veterans for usability and viability validation. The core focus will be getting a working conversational interface for these veterans to start interacting with in a controlled environment. A primary focus of our user research will be to gather some baseline data on levels of health literacy for veteran participants speaking English and Spanish, the languages supported by the Short Test of Functional Health Literacy in Adults (T-TOFHLA) test. We also want baseline data on rate of care plan adherence rates, which we plan to measure using the Patient Adherence Survey developed by the Rand’s Medical Outcome Study (MOS). As we are developing our prototype, we will measure individual comprehension of medical information and gather feedback about user experience as we work through iterations of our model using health literacy and user satisfaction surgery’s. By next year, we want our participants to demonstrate a statistically significant positive improvement in T-TOFHLA and MOS Patient Adherence Survey scores in both test groups, with and without assistance of our virtual healthcare assistant. Overall, we are aiming for higher patient and caregiver satisfaction with our solution than incumbent patient engagement and chart access tools on the market. Over the next five years, we aim to have the MVP of our solution in the hands of patients at some small, innovative healthcare organizations with demonstratively higher health literacy levels and patient adherence. We want to seek out early-stage partnerships with some healthcare organizations who are innovators or early adopters to explore how use of our tool can improve health outcomes in their patient populations. We will use patient satisfaction metrics to understand patient experience gaps that we need to close and assess requirements for improvement of our AI systems. We will conduct regular assessments on each stage of development and ongoing improvement of our AI systems to evaluate our adherence to responsible AI principles to ensure that we are making a positive and ethical impact on patient lives with our application. We plan to connect with health literacy advocacy organizations such as the Institute of Healthcare Advancement (IHA) and the Office of Disease Prevention and Health Promotion (OASH) to get connected to resources and partner with them to measure outcomes of AI-assisted consumption and comprehension of medical information.
- Not registered as any organization
Currently, our team consists of 6 part-time staff who have careers in parallel to all of the work we are dedicating to this solution. We aim to bring on a contractor for our UI/UX design as well as a data scientist contractor to help us build and fine tune our AI models.
We started working on this solution this year coming out of the MIT Grand Hack and as a team, we have participated in a couple more hackathons since. We have been working on our solution for a little over half of a year.
Our team is very diverse, with members coming from various different races and backgrounds. Our team is still quite small but we have great representation: I am Chinese American, a technical expert who is Indian, a physician who is Egyptian, an process innovator who is Jewish American, and another technical expert who is white. We want to remain diverse, equitable, and inclusive and this is something that we will remain mindful of as we expand the team and bring on more people. We want everyone to be heard and we value everyone’s opinions and perspectives. I am a staunch believer that incorporating ideas through collaboration with individuals from diverse backgrounds and experiences will help us build the most successful solution.
Our team members have diverse professional backgrounds and expertise that puts us in a good position to build and operationalize our solution. We have a clinician entrepreneur on our team who will be responsible for driving our clinical strategy and another member of our team is a clinical process innovator who will be focused on the clinical implementation of our workflows. We have another team member who is the Chief of Family Medicine in Tampa and is conducting his own clinical research on impact of generative AI in primary care. We also have great technical expertise on our team with one member who is a UX engineer and strategist along with another member who is a back-end developer with a background in building healthcare chatbots. I have a background as a product leader at healthcare product organizations and as the team lead, I will be leading our product strategy and managing our business operations. Patients and their caregivers are key stakeholders for our product and we will engage them with our design and development process as closely as possible from the get-go. We plan to conduct user research on various populations of veteran patients and veteran caregivers through the VHA accelerator program. Additional stakeholders we want to include in our validation will be the discharging physicians and the primary care physicians following these veteran patients because it is crucial to incorporate the patient’s care team building our solution. We want to engage innovative leaders in the health literacy and health equity space who we can partner with to implement and operationalize our solution at their respective organizations. Down the line, we want to engage insurance payers or accountable care organizations and partner with them to make our solution available for their patients and measure clinical and financial outcomes. From a technical build perspective, we have access to some powerful tools such as Amazon SageMaker along with TensorFlow that will allow us to build, train and deploy our large language and prompt tuning models. We will use the production-ready LangChain toolkit that will allow us to connect those models to our data sources within a powerful language chain. We plan to use Node.js as our back-end infrastructure paired with React Native as our front end technology, both open source libraries that the technical members of our team are experts in. We will utilize AWS cloud hosting and data storage services which will allow us to scale our infrastructure in the future while making configuration of data pipelines for our model feedback data for ongoing training and optimization of our models easier. We plan to integrate with medical record systems and various third party services through REST APIs to pull in patient data, educational materials, provider directories and more to achieve our vision of a single, centralized hub for patients to access information about their health.
We plan to bring in money through a combination of different means. Through our participation in the VHA accelerator, they will be providing us some funding to conduct our user research and build our prototype, although the capital level is still relatively low. We want to raise some more capital that we can invest in the R&D needed to build, train and deploy our generative AI models. We will seek out grants with health equity and literacy advocacy groups to support our the development of our solution and who will hopefully connect us to further grants or capital resources. There are also health insurance companies with health equity initiatives who will provide programming and grants that we will apply for. We may also seek out angel investments to fund our work and I have already been connected to some interested investors who we can initiate those conversations with when we are ready. After we have our prototype built, we want to conduct some early stage pilots with some value-based care organizations and some smaller healthcare systems to demonstrate positive patient outcomes that lead into longer term contracting to license the use of our solution for their patient populations. We will plan to seek out some health insurance companies as prospective buyers who may be interested in licensing the use of our solution for their subscribers.
We currently do not have any operating costs but we are estimating our technology spend to increase over the next year. Based on our projected development costs and lower volume of initial users while we are validating our prototype, we are predicting monthly data storage and compute costs of around $15,000 and around $1,500 in monthly software costs. We do not currently have personnel expenditures to report for the team but as we begin engaging with stakeholders over the next year, we will have travel and operational costs that project to be about $2,000 per quarter for members of our team over the second half of this year. We will bring on a data scientist contractor for a one-time contract fee of $10,000 with an estimated $700 monthly cost for monitoring for six months after initial deployment of our model. In total, our projected operating cost is $117,200 over the next year.
We are seeking $75,000 in funding over the next year. We plan to apply for the AWS Activate credits to offset some of our data and compute costs which make up a majority of our operational spend over the next year. We want to invest $60,000 in the research and development of our AI models, conduct user experience research, and the general development of our MVP solution over this next year. We have an investment from the VHA accelerator that will help us with some of our R&D costs as well but it will be far from covering those expenses. We want to invest $15,000 in stakeholder engagement and business development costs to gain market interest and conduct outreach to prospective healthcare organizations who may be interested in piloting our solution. Any leftover capital will be reinvested into our R&D budget or could help us cover some of the potential implementation costs after we finish building our prototype over the next year.
The Cure Residency will be an amazing opportunity for us to continue developing our idea and building our prototype. I am most excited about the mentorship, the educational programming and networking opportunities that the residency can provide. Mentorship from members of the Cure Advisory Board would be an invaluable resource for me as a budding healthcare entrepreneur and I want to continue learning from some incredible leaders in the industry. Ultimately, I want to be a leader in the healthcare AI space and this residency will help position me to do so in the future. I am very excited in the Residency’s professional development programming, which will teach me valuable lessons on forming and running an early-stage company as one of its co-founders. I want to continue building on my leadership skills and entrepreneurial expertise to be able to lead my team on our journey to bring this solution to the patients who need it most. The networking opportunities will connect me with other innovators who are passionate about revolutionizing healthcare. I want to be a thought leader in this space and meet like-minded people who are driven towards improving healthcare for good. Having this network would allow me to learn from my peers and I am excited to have thought-provoking conversations about how others are innovating with AI to solve the problems with healthcare that they care about. Gaining access to the Cure headquarters will also place me in a surroundings that will fuel the passion that I have to build our solution. Having done healthcare hackathons in the past such as the MIT Grand Hack, having a collaborative environment like that is exhilarating and plants the seeds for innovation. From a business operations perspective, we are also very excited about the Cure Residency’s funding. This capital would be an incredible investment in our idea and will give us a financial runway to validate our idea and develop our solution. A bigger budget for research and development will allow us to acquire tools and resources to build and deploy our AI systems.
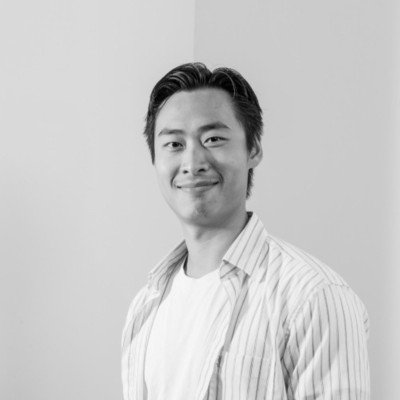
Product Management
Senior Product Software Engineer
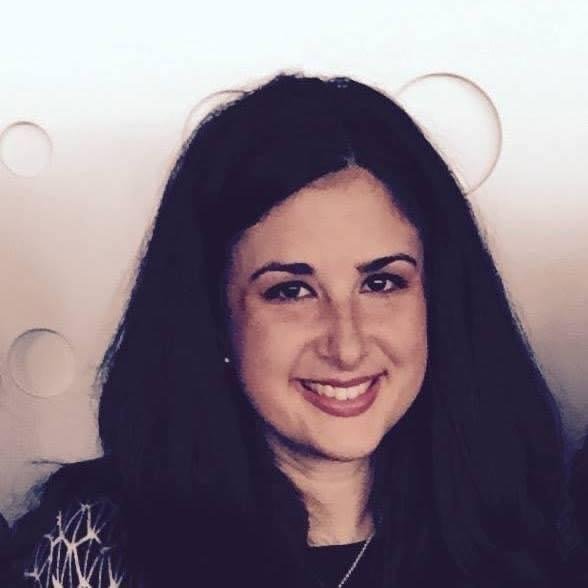
Occupational Therapist, Clinical Specialist