Artificial Wisdom in BioMedicine by MedScen
Labeling data is the most controversial part of Machine Learning (ML) and Artificial Intelligence (AI).
When it comes to patient health data or biomedical laboratory tests, details matter. Crucial details must be recorded and they must be correctly labeled at the time of the patient visit or at the time the laboratory test is performed.
Correctly labeled health records is beneficial:
An AI based on correctly labeled and detailed patient data can efficiently assist the doctor in diagnosis and choosing the best individually tailored treatment for the patient. This AI will assist the doctor with rare diagnoses through the following:
A. Using simple statistics, the AI calculates the probability of seeing a rare diagnosis in the near future based on previous visits.
B. Reminds the doctor to ask all simple questions that are key in diagnosing rare diseases.
Detailed biomedical laboratory test records can prevent waste in clinical research:
MedScen will force the laboratory technician to record and report all the nuanced details that may not sound important for the technician but are crucial in pooling data and comparing laboratory tests.
For example, the process of separating Peripheral Blood Mononuclear Cells (PBMCs) is expensive and includes multiple steps that differ between laboratories due to differences in routine protocols. Some laboratories prefer to perform this procedure on ice and after incubating the blood in fridge overnight, whereas, some laboratories perform this procedure in room temperature on freshly taken blood. These important details are unfortunately either not reported or overlooked in making final conclusion from accumulated data of multiple research.
A good example of this is the failure of using PSA as a screening tool for prostate cancer. Hundreds of clinical research and thousands of dollars were wasted because the researchers failed to recognize an important small contributor to bias in their research: performing rectal exam before taking blood for PSA exam changes the results of the research:
https://link.springer.com/arti...
This simple confounder caused a massive impact on ROC AUC of the screening test and caused thousands of false diagnoses.
MedScen brings wisdom to Artificial Intelligence by adding experience.
MedScen is a collection of scenarios. Each scenario is a tree of labels made by experienced biomedical doctors who have experience in treating patients, perforrning laboratory exams, clinical trials and data science and ML. Each label in these scenario has a well-defined definition that will standardize the recording process (standardized biomedical language).
MedScen reduces bias in ML because it does not require Natural Language Processing (NLP). All data is labeled by the user (practicing doctor/nurse) at the time of visit or by the laboratory technician at the time of procedure.
Everyone will get sick.
If you are a human reading this, you will get sick at some point in your life. You will require consult regarding your health one day. That consult may require biomedical laboratory tests performed on you. At the end of the consult, you will make a DECISION.
MedScen will help your doctor make a better decision for you with your help. MedScen teaches wisdom to AI so it can be a better assistant to your doctor.
Mehrsa J is a medical doctor trained in biomedical research and data science. She has performed multiple biomedical laboratory procedures, and clinical trials. She has personally observed waste in these settings.
Mehrsa J has been analyzing medical and biomedical research data for the past 8 years. Her speciality is time-series analysis and simple statistics.
Furthermore, Mehrsa J has treated more than 80,000 patients in clinic and emergency settings and have scored top 5% in USMLE.
Saman S is an experienced coder who have been managing a large international IT company for the past decade.
- Enhance efficiencies in clinical trials and research, including data collection and sharing.
- Australia
- Brazil
- Concept: An idea for building a product, service, or business model that is being explored for implementation; please note that Concept-stage solutions will not be reviewed or selected.
We need moral support. Financial support is a big plus
Mehrsa J has worked with CLICKs, (a click-based health recording system that uses the same concept as MedScen.com however lacks the scenarios).
Mehrsa J is connected to doctors and professors of UNICAMP, the second largest university in Brazil.
Saman S owns an AI company.
The solution has a core: label data at the time it is being recorded.
This has multiple advantage.
A. Data is labelled at the time it is happening, the person recording the data is forced to pay attention to the details or ask extra questions to find the correct label.
B. Labels are standardized and well-defined for the data collector so there will be global understanding of all terms.
C. There will be no NLP so the analysis of the data is not limited by the bias and errors caused by NLP.
D. MedScen.com has a collection of suggestions to standardize biomedical research. For example, if the data collector is recording the results of PBMC extraction, MedScen will remind them to record the temperature, a step that is often overlooked. Or if a researcher is recording the PSA results of a patient, the researcher is reminded to ask the patient whether there was any anal penetration before the PSA exam. This question is private and embarrassing for the patient, however the answer to it is crucial in interpreting PSA results; the researcher can blame the software as a scapegoat.
In the next one year there will be a collection of all possible MEDICAL scenarios in MedScen. As mentioned before, scenarios are made of interconnected label trees.
In the next five years there will a collection of all possible scenarios in BioMedicine (clinical trials, laboratory procedures, etc.).
MedScen is only click-based, however there will be a space for typing: the user can type and explain about any biomedical situation that cannot be described by the labels already provided. These comments will constantly be reviewed and new labels will constantly be added.
MedScen executives will constantly monitor users to make sure they are using the software correctly. Any misunderstanding in handling the software and labels will be recorded and dealt with.
MedScen is the only way for AI to have a truly beneficial impact on human health. Currently AI does not clearly understand the human body because no one has correctly defined the medical terms and the biomedical laboratory tests that define the human body.
The results of any machine learning is dependent on the quality of the data gathered. MedScen improves the quality of data by reducing missing values, bias in interpretation of results, and ensuring that all confounders are present. MedScen allows pooling of data gathered from different sources that vary in their routine procedures because it ensures that all those nuanced details are recorded.
Cloud based data gathering of large health-related information.
A software with easy user-interference
- A new application of an existing technology
- Big Data
- Software and Mobile Applications
- Not registered as any organization
2
7 years
We will use MIT approach
If MedScen is bought by government, we accept a yearly salary.
If MedScen is to operate as a private company, we will sell health related data under HIPAA supervision
- Government (B2G)
The expensive part of MedScen is maintaining the data warehouse. That part will be delegated to more experienced teams.
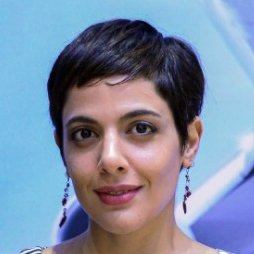
Medical Doctor / PhD Student / Scientist with Autism
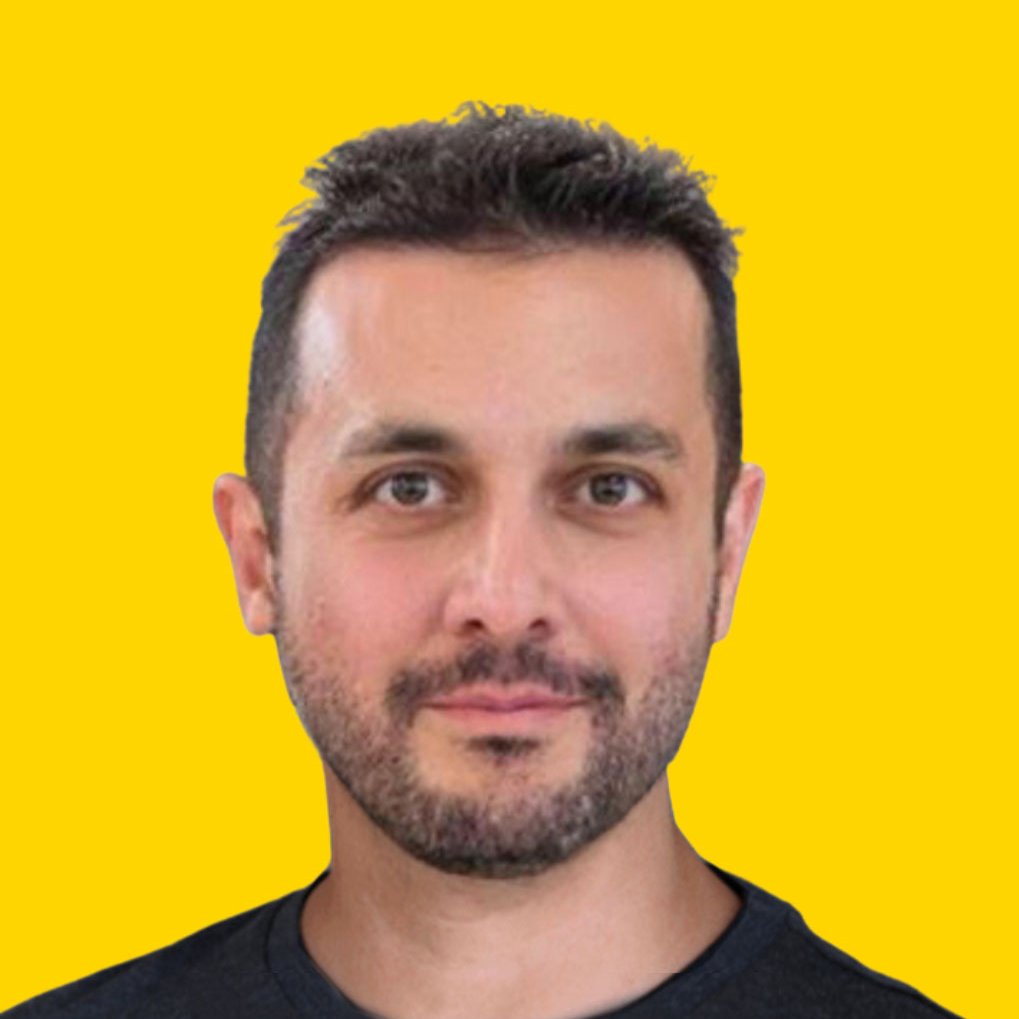
Group Engineering Lead @ Canva / PhD candidate, Computer Science and Engineering, University of New South Wales (UNSW), Australia