PhenotypIQ AI discovery platform
We are automating the intelligence portion of phenotypic cellular analysis. Why does this matter? In drug discovery research teams are often trying to first test the effectiveness of a potential drug or therapeutic by measuring its effect on a certain attribute, e.g. whether administration of a compound increases expression of a particular protein. If effective, and moreso than others, then an asset progresses in a workflow to other tests, such as animal testing for toxicity. Years of tests along this workflow must be conducted to position a top therapeutic for success in clinical trials, and even then a small fraction of therapeutics actually are granted market approval.
The high costs, risks, and extensive timelines of both preclinical and clinical drug development, combined with the ticking clock of patent protection, mean that once drug companies do bring a drug to market they typically price it exorbitantly to recoup their investment before an enveloping patent expires. Ultimately, consumers suffer both from high therapeutic costs and reduced investments in disease indications with low populations.[1]
When dealing with destabilizing global events, such as pandemics, it is critical to: (1) reduce the timelines of identifying and developing an effective therapeutic, e.g. an antiviral; and, (2) to democratize the ability to accomplish this globally. Machine learning computational workflows are vastly underutilized in drug discovery.[2] High-throughput quantitative imaging is one application of machine learning with the potential to revolutionize assay design, candidate selection and optimization, while predicting mechanisms of action across compound families. The problem in utilizing machine learning workflows generally is that few drug discovery teams, whether parts of universities, small biotechs or even large pharma, feature machine learning scientists. Even fewer feature scientists with imaging expertise.
Preclinical workflows in drug discovery are ripe for radical disruption through image-based, phenotypic screening.[3] If a truly plug-and-play, deep learning platform were available, then researchers could simply upload their in vitro experiment images and obtain rich phenotypic modeling information to guide their decision making. Ultimately, widespread adoption of this technology allows for streamlined discovery by combining efficacy and toxicity paths into a single model, reducing animal experimentation, and further allowing reverse prediction capabilities of experiments and structures that would result in desirable phenotypic states.
[1] Robin Feldman, May your drug price be evergreen, Journal of Law and the Biosciences, Volume 5, Issue 3, December 2018, Pages 590–647, https://doi-org.ezproxyberklee.flo.org/10.1093/jlb/lsy022
[2] McNair D. Artificial Intelligence and Machine Learning for Lead-to-Candidate Decision-Making and Beyond. Annu Rev Pharmacol Toxicol. 2022 Jun 9. doi: 10.1146/annurev-pharmtox-051921-023255.
[3] Malandraki-Miller S, Riley PR. Use of artificial intelligence to enhance phenotypic drug discovery. Drug Discov Today. 2021 Apr;26(4):887-901. doi: 10.1016/j.drudis.2021.01.013. Epub 2021 Jan 20. PMID: 33484947.
In simple terms, you can draw a corollary of our work to facial recognition technology. In these applications, automated analysis of as little as 60 nodes of a human face has the power to identify a person and unlock a vast range of security, transactional, and even health applications. We automate the analysis of approximately 1,500 image-based nodes of a cell, and in that analysis can model how a cell changes in response to potential drugs, whether small molecules, biologics, or genetic therapies. This analysis shows at a high level whether a diseased cell is moving back to a healthy phenotype, and along the way provides insights into mechanisms of action, efficacy and toxicity.
With phenotypic analysis, research teams may now access technology to extract and model thousands of specific morphological data points that a cell expresses, in its optimal state, when it is diseased, and when it is treated. This type of approach provides orders of magnitude greater intelligence regarding how a potential therapeutic is working within a cell, and scientists can use this information to make better decisions in early stage drug selection. Ultimately, image based screening can move the industry away from animal testing (because degrees of toxicity can be modeled in the images), and even provide the ability in reverse form to predict what types of biological/chemical constructs will produce a desired phenotype.
To get here, research teams need to understand how to create and use tools for analysis and prediction of cellular images. Since very few teams employ AI/ML scientists, there is tremendous opportunity for an upload-and-see / plug-and-play image analysis enterprise platform that automates the AI/ML scientist's work. Our phenotypIQ platform will be an enterprise disruptor, and further allow us to enter into 1:1 direct partnerships with pharma under customer asset development deals.
Our initial work is based on technology developed at the Broad Institute, and we are in discussion with the Broad for in-licensing technology developed by QUANTiSCOPE co-founder Juan Caicedo, which we will place into pilot studies this year with a large pharma, a small biotech, and a foundation.
The wireframes of our platform can be found here.
We launched in August 2022 through the federal Biomedical Advanced Research and Development Authority (BARDA) startup program. Our approach is of significant interest to federal agencies tasked with medical countermeasures and pandemic responsiveness. Our populations are threefold: (1) global populations are risk of epidemics and pandemics; (2) the rare disease community; and, (3) populations in less advanced economies with limited drug development innovation ecosystems.
We satisfy goals 3 and 17 of the UN SDGs. The Gates Foundation has written on the opportunities of AI/ML to improve drug access, and we have already targeted Access Accelerated about how we can fit in as a pipeline partner to accomplish non-communicable disease medicine goals. In a 2021 Access review, the 20 participating pharma companies scored high in pricing strategies but low in innovation models. Our platform can help with drug repurposing and combination strategies, and reduced costs for dedicated drugs in lesser developed countries, e.g. small molecules vs biologics.
Cell painting is one type of phenotypic analysis that can expand rapidly through our platform. In 2021, a team of researchers at the Karolinska Institute in Stockholm were able to utilize cell painting for the identification and screening of novel broad-spectrum antivirals against Ebola, SARS-Co V-2, and Zika. Other customized applications have pushed clinical candidates into trials at an 80% reduction in time. However, in each success case, extensive resources and dedication, often through years of a doctoral student's time, were required. Even then, the learning and application of open source analysis tools, less robust than ours, was a very manual approach. Our users can take advantage of this improved platform for the ultimate benefit of the populations identified above.
Juan and I came together to solve an important issue, one that I have seen professionally as a life science founder and patent attorney, and personally as survivor to a family member lost to a rare disease. Drugs take too long and are too expensive to develop, meaning pharma passes on risk and limited patent life to consumers in the form of high prices and ignored, less popular indications. Juan is an immigrant, and my mother is an immigrant, and we both are also cognizant of how poorer countries around the world have less access to population treatments because of this industry problem in the U.S. and Europe. Juan and I want to create better access to safer drugs, both in the U.S. and abroad.
My own professional background includes work for many years to develop a broad spectrum antibiotic. The molecular class was originally developed through a public-private partnership, and in-licensed to a startup company that I co-founded. We spent years trying to advance a lead asset into clinical trials, through large public grants, venture funding, and ultimately through a joint venture. Despite significant champions, resources, and science, we were not able to beyond the pre-clinical phase. I am championing the QUANTiSCOPE platform because I know how relevant it is for efficient, meaningful decision making in pre-clinical evaluation and candidate selection. I'm well networked within the industry to understand pain points and the business model of how our platform scales, and Juan is the innovation engine behind our work.
- Enable informed interventions, investment, and decision-making by governments, local health systems, and aid groups
- United States
- Prototype: A venture or organization building and testing its product, service, or business model, but which is not yet serving anyone
Our models have been trained and tested in an academic setting, we merely need to move them out of the lab and into a dashboard workflow. We are actively scoping pilots to do just this.
Currently none, we are in pilot phase.
Technical expertise: we need to build a solid innovation leadership team to expand this in multiple application areas.
Impact: we are impact-oriented, and want to ensure that this type of technology can be developed in a way for broad public impact.
Industry partners: beyond our initial pilot partners, we want to connect with foundations, industry, and government organizations who can help inform the direction our technology and business needs to go.
- Business Model (e.g. product-market fit, strategy & development)
- Human Capital (e.g. sourcing talent, board development)
- Product / Service Distribution (e.g. delivery, logistics, expanding client base)
- Technology (e.g. software or hardware, web development/design)
A key component of our platform involves the incorporation of advancements Juan's group has made at the Broad in autoencoder design. Image autoencoders are computer programs that can be used to generate or modify digital images. They work by breaking down an image into its individual features and then reassembling it based on those features. Autoencoders are widely used in computer vision applications, including things like style transfer, image editing, and representation learning. One way to train an autoencoder is to use a loss function that maximizes either the quality of the generated images or the quality of the feature representations. We take a different approach, using a modular strategy that allows us to separately train the encoder and decoder networks for their own tasks, ensuring high-quality semantic representations and high-quality image reconstructions. This is a technology we intend to in-license from the Broad, and it is this unique aspect of a SaaS offering that allows us to move across biological experiments, opening an offering suite that is not limited to certain types of experiments, cells, or therapites. Beyond that, we have some ideas we want to employ to utilize CRISPR for broadening cell painting to get beyond existing limitations in staining types and regions.
Our 2023 milestones including placing our MVP in sponsored pilot studies to demonstrate customer ROI. We will use those metrics to position for a seed raise and technical hiring. In sum, our near-term milestones include:
• Raising a small, pre-seed round to facilitate MVP optimization to take advantage of sponsored pilot studies.
• In parallel with ongoing pilot studies, we will iterate our algorithms based on pilot feedback, and develop early academic/industry adopters.
• Based on MVP data, we will target a seed round, with strong verbal interest to date.
We will launch our SaaS platform in 2024 and goals will be on uptake and innovation. Use of our platform will further inform our models, and we can position for 1:1 custom development partnerships within the industry, helping pharma hand-in-hand to understand how to move toward a phenotype centric discovery model.
In terms of impact, we will monitor for how our customers increase development timelines and reduce use of animal testing. We will also specifically partner with foundations to employ our platform for rare disease research.
- 3. Good Health and Well-being
- 9. Industry, Innovation, and Infrastructure
- 10. Reduced Inequalities
- 12. Responsible Consumption and Production
- 17. Partnerships for the Goals
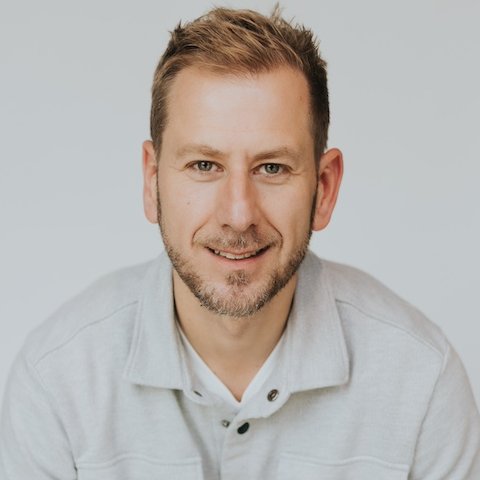