Image Classification for Natural Disasters Aid
We can use existing satellites to classify how much has an area been damaged after a natural disaster, providing a way to allocate necessary resources more efficiently.
We have recently seen what the impacts of climate change are. The increase in global surface temperatures escalates the possibility of more intense storms and more droughts, that lead to bushfires.
2019 was the hottest record year in Australia, causing one of the worst bushfire seasons in the country's history. These events caused great damage throughout the country, affecting all states and territories, and thousands of lives.
While this solution does not aim to solve bushfires, it aims to provide a way to allow local governments and other responsible bodies to use technology as a way to locate areas that have been heavily affected after a natural disaster. This solution could mean a more efficient resource allocation that could potentially save a life.
Essentially, image classification algorithms are used to take satellite images and classify them into two groups: "damage" and "no damage". Currently, the model was trained using one dataset from the areas affected by Hurricane Harvey in 2017. The model has an accuracy of 98%.
The future goal for this project is to train the model with more datasets. For that, we need access to satellite imagery taken in areas that have been affected by natural disasters.
This solution is aimed to help the Australian population, especially those who are located in areas that are prone to floods, cyclones, and/or bushfires.
Currently, the necessary organizations and bodies that are responsible for controlling and aiding during these disasters are doing their best efforts. However, when bigger disasters occur, as seen during the 2019-2020 bushfires, there were not enough people to help, and the Australian government brought people from overseas.
This solution aims to provide a way for a more efficient resource allocation when these events occur by alerting what areas are in most need of help. It could also mean that an event can be handled to not get quite to the size of what was seen in 2018-2020, by alerting early what areas are affected.
As a resident of Australia, Tatiana has knowledge about the frequent bushfires and flooding that happen near the area where she resides. She has also talked to people that were affected by the Australian bushfires in the last two decades.
Juan Diego was a Red Cross volunteer. He trained to be able to provide emergency aid to people during events such as earthquakes, fires, etc.
Both Tatiana and Juan Diego are currently working as Software Engineers. Both have experience in training and building machine-learning algorithms, as well as creating apps for the visualization of complex data.
We have reached out to organizations that could help us acquire access to satellite images, however, it has been unsuccessful. We believe that more data is needed before we reach out to potential users, the solution we plan to build is one that we want to ensure is of quality and thoroughly tested.
This is why we wanted to apply to this challenge. We are confident that we can develop further our algorithm if we have access to more data. Participating in this challenge means that we will be able to reach out to more organizations that could potentially assist us in our mission to gain more data to train our algorithm.
- Taking action to combat climate change and its impacts (Sustainability)
- Concept: An idea being explored for its feasibility to build a product, service, or business model based on that idea.
While there are already existing technologies provided to firefighters to combat situations such as natural disasters. We believe our solution to be innovative as it could breach the gap between existing technologies, such as satellite imagery, and personnel who are trained but might not be able to access the view of a satellite.
We expect this solution to positively impact those who work for emergency services during natural disasters. The goal is to provide a clear map showcasing areas that are in need of help during or after a natural disaster. By doing so, the relevant emergency services will be able to reach those affected areas faster, possibly saving someone in need.
We mainly want to gain access to more data in the relevant areas where we want our solution to work. By doing so, we will be able to train our model to classify areas that have been affected by natural disasters, allowing emergency responders to go to the most affected areas and provide the necessary aid.
Machine learning and pattern recognition, in the space of image classification.
- Artificial Intelligence / Machine Learning
- Software and Mobile Applications
- Australia
As this is only a pilot, this solution currently serves no one. We would like to initiate by training our algorithm in areas within Australia that have more probability of experiencing floods or bushfires.
In January 2003, the capital of Australia, Canberra, experience a big season of bushfires. We would like to attempt to find satellite imagery of that time and train the algorithm to classify affected areas by bushfires in Canberra. By doing so, we would expect to serve the Canberra population of approximately 390,000 people. Including the firefighters working in the nine firefighter stations located in the city.
Our main issue in further developing our algorithm is the lack of access to satellite images. To be able to train our model, we need more data, specifically data for Australian locations, such as Canberra.
We are not partnered with any organization.
We would provide our solution as a service to local governments. By displaying the classified data through a web app. The idea would be that local governments provide the necessary emergency services with the software, for them to quickly respond to natural disasters.
Initially, we would seek grants from the Australian government, during that time we would make sure to further develop the algorithm, and build the interface to display the data efficiently.
Later, we would aim to sell the finished product as a service. During this time, we would seek to continue improving the algorithm with collected data, and feedback from those who interact with it.
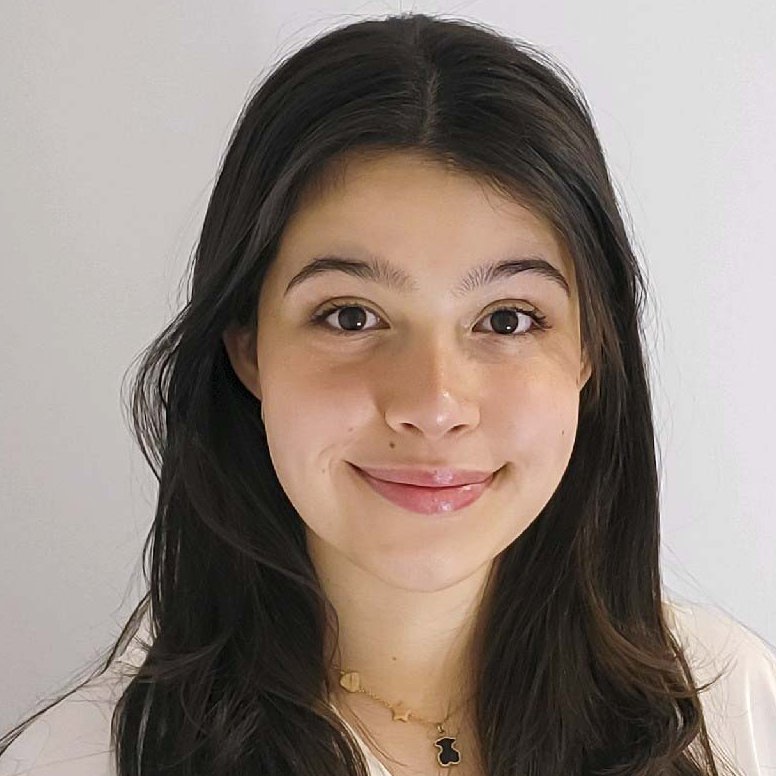
Software Engineer