BRAC-mPower AI/ML-based Pregnancy Risk Categorization
According to UN global estimates in 2018, about 303,000 women in a year die in childbirth, or as a result of complications arising from pregnancy around the world. This amounts to about 830 women dying each day - roughly one every two minutes. According to WHO, the vast majority of these deaths (94%) occurred in low-resource settings, and most could have been prevented by timely interventions. In Bangladesh, the rate of maternal mortality is 173 per every 100,000 live births according to the World Bank Data in 2017. Most of these deaths happen in rural areas, where more than 60% of the population lives, where doctors are scarce. This chronic health human resource gap is served by community health workers (CHWs).
BRAC’s Health Nutrition and Population Program (HNPP) currently serves more than 75 million people in Bangladesh with total 4,300 trained community health workers (CHWs) called Shastho Kormi (SKs) who visit pregnant women, screen for non-communicable diseases, counsel and refer to health clinics. Because each of these CHWs is responsible for around 4,000-5000 households, their attention is somewhat spread thinly among the pregnant women identified, and hence an accurate risk prediction can greatly enhance their ability to design targeted interventions, and counseling.
Over the last 5 years, BRAC HNPP has digitized its entire service delivery process, including the introduction of a platform to digitally record all patient interactions, both with CHWs and facilities. This dataset now includes longitudinal data of over 63 million beneficiaries, with socio-demographic history, family economic status, pregnancy history, ANC/PNC service records with biological risk factors, GPS locations, and delivery information including complications and ultimate pregnancy outcomes.
For a more targeted resource allocation for timely interventions, HNPP has been using a rudimentary risk assessment method using a simple checklist of risk factors to determine if a particular woman is “risky” or “not risky”. This risk stratification is used for categorization by their need of support, probable complexities, and ensure that health volunteers (a group working under the supervision of SKs) call them with more priority or SKs to counsel them on taking proper care and prepare them for emergency cases, and ensure delivery at a facility.
According to our analysis, this check-list based risk identification method has a high degree of both inclusion and exclusion errors. To be exact, 87% of the pregnant women our system predicted to be “risky” ended up having no pregnancy complications. On the other hand, 9% of the pregnant women our system predicted as non-risky eventually turned up to be at risk as they faced pregnancy complications.
From 2021 BRAC has identified 1.1 million pregnant women and has digitized longitudinal data for .88 million pregnant women by providing antenatal care. This longitudinal data was digitally collected several times over the period of their pregnancy, and not only do we have medical, socio-economic, environmental data (such as family economical status, household head’s education status, pregnant woman’s vitals, diabetes and blood pressure and other health data collected every month, nearest facility etc.) but also data on eventual pregnancy outcomes (such as delivery outcome, delivery complication, health seeking behavior, EIBF, etc).
Using this data, we have already developed a machine learning model to reduce the error rates compared to a check-list based method. We analyzed a total of 38,765 data points from the same number of patients in the Dhaka district. We divided the data into a training set (25,843 samples) and a testing set (6,461 samples) and developed a ML-based model using the “Random Forest” algorithm. While the traditional checklist-based model resulted in 78% accuracy, our ML-based model yielded a 95% accuracy in predicting the risk of the participants. The improvement was more evident in different subgroups within this sample. For example, for the patients who had pregnancy before, the accuracy of our model leaped up from 74% to 95%. Similarly, for participants with a gross family income more than 12 thousand BDT, the accuracy rate increased from 77% to 95%.
Besides the accuracy level, we have seen a significant improvement in reducing the “false positive” cases. For the whole sample, the checklist-based model had a false-positive rate of 89% which dropped to 11% in our model. Similarly, there was an improvement in reducing the “false negative” case. While the traditional model had a false-negative of 9%, our model decreased that to 6%. The improvement that all these outcomes that we observed was due to the probabilistic distribution of weight on different features that we achieved through using ML-model.
While our current ML-based algorithm has shown significant promise, there is still room for improvement in the accuracy of these predictions, especially for scaling up the use of the ML-based method within the HNPP program in all of Bangladesh. One important area for improvement is significant reduction of “false positive” and “false negative” cases, and ensuring that the model is applicable for all of Bangladesh. Unintended exclusion error can cause a potentially “risky” pregnant mother to be labeled as “non-risky”, thereby preventing/ discouraging her from accessing relevant services and timely interventions for more risky cases. By the same token, unintended high rates of inclusion error can lead to suboptimal use of resources for a broader group of pregnant women than ideal.
Under the scope of this project, we will enhance our current machine learning models to increase accuracy of prediction of risky vs non-risky, and include a third intermediary level of “moderate risky” - a category suggesting that the case may move to high risk at any time, and needs close supervision.
We will enhance the model by the inclusion of more social, demographic and economic factors, and also running the model with a bigger national level data set, and using more advanced analytics such as dynamic rating over time. In addition, by identifying the non-traditional risk factors correlated with various types of adverse outcomes (such as stillbirth, early delivery etc.), and the ways they interact with biophysical risks, we will enable novel interventions to manage the risk and pre-empt its escalation, ultimately leading to improved pregnancy outcomes for pregnant women.
While our model worked relatively well on the data from a single region, it needs to be updated to transfer this knowledge for a model that can work for the whole country. To achieve these goals, based on cutting-edge research in public health, we will incorporate the impact of “external factors” including climate, education level, and financial strength of a region in our prediction model. We will develop a deep-learning model that will ensemble the already available data that we have collected with the publicly available data on the climate, education, and economy to produce a better prediction for patients in a particular area. This model will update itself using an active learning technique by incorporating two factors: (a) the change in the external variables, and (b) the success of our prediction.
The second aspect of our solution will be to enhance the communication of the risk stratification to beneficiaries and design interventions based on risk level.
For this, we will be conducting a HCD analysis. In a Carnegie Mellon study it has been shown, a well communicated stratified risk rating (a 3 to 5 stage risk grading or a matrix of multiple indicators) compared to a binomial (risky vs Non-risky) risk rating can increase patient's overall risk awareness and number of prenatal visits from 50% to 85% through temporal risk feedbacks, institutional alerts and targeted suggestions (Krishnamurti et al, 2017). To avail this higher level of outcome, the stratification method and the associated communication might also need to be customized according to the stages of pregnancy, geo-location, socio-economic context, literacy level.
A human centered design exercise connected with an user-experience proto-typing research on the various dimensions of risk-stratification communication targeted towards the CHW will help the team transfer the knowledge gained from the ML model into implementation. For this, in the inspiration phase, a team of HCD experts will create user personas, and outline user journeys through attitudinal qualitative focus groups.
In the innovation phase, the UX researchers will create prototypes to visualize and communicate risk stratification, and notification through participatory design exercises with both direct users (CHWs), and indirect users (supervisors and beneficiaries).
In the implementation phase, the prototypes will then be tested and modified in iterative sprints, validated by qualitative usability testing and quantitative AB testing.
References:
1. Khan, Aneire Ehmar, et al. "Drinking water salinity and maternal health in coastal Bangladesh: implications of climate change." Environmental health perspectives 119.9 (2011): 1328-1332.
2. Kuehn, Leeann, and Sabrina McCormick. "Heat exposure and maternal health in the face of climate change." International journal of environmental research and public health 14.8 (2017): 853.
3. Barman, Bikash, Jay Saha, and Pradip Chouhan. "Impact of education on the utilization of maternal health care services: An investigation from National Family Health Survey (2015–16) in India." Children and Youth Services Review 108 (2020): 104642.
4. Adjiwanou, Vissého, Moussa Bougma, and Thomas LeGrand. "The effect of partners' education on women's reproductive and maternal health in developing countries." Social Science & Medicine 197 (2018): 104-115.
5. Powell-Jackson, Timothy, and Kara Hanson. "Financial incentives for maternal health: impact of a national programme in Nepal." Journal of health economics 31.1 (2012): 271-284.
6. Filippi, Véronique, et al. "Maternal health in poor countries: the broader context and a call for action." The Lancet 368.9546 (2006): 1535-1541.
Currently in 2022, BRAC HNPP is serving 75 million people in rural Bangladesh where at least 2.3% remain in pregnancy status at any given point. Our risk stratification solution serves these pregnant mothers of rural Bangladesh, most of whom suffer from limited access to information and health care services.
A more accurate risk stratification will enable the SKs to inform pregnant mothers of their probable complexities and help prepare them for emergency cases. As a result, pregnant mothers and their family members can take precautions and prepare for facility delivery and other precautionary measures, which can drastically minimize the possibility of negative outcomes. A more accurate risk stratification will also enable the SKs to more optimally use their time for more targeted interventions for those who really need the attention.
Our team is composed of deep-rooted expertise in implementing health, population and nutrition programmes for and with the disadvantaged communities in rural areas through frontline health workers while utilizing technology, human centered design and machine learning approach in those health, population and nutrition interventions.
Mridul Chowdhury, Founder and Chief Executive Officer of mPower, who is leading our technology team also has more than 20 years of extensive experience in implementing ICT solutions for development projects, with particular focus on the health, population and nutrition sector of Bangladesh and 17 other developing countries. Some of these mHealth solutions are being implemented nationwide and intended to reach 100+ million beneficiaries from marginalized communities.
Morseda Chowdhury, Director of Health, Nutrition and Population Programme (HNPP) of BRAC, who is leading our health service implementation team, has more than 20 years of experience in codesigning and implementing a portfolio of 21 projects with an annual budget of USD 28 million including grassroot level interventions and RCTs in the areas of reproductive health, communicable and non-communicable disease and nutrition.
We also have Zaki Haider, Director of Innovation of mPower, as a Human Centered Design (HCD) Expert who has more than 15 years of international experience applying HCD approach in building and launching successful software products and services, ICT based MEL and SBCC platform in the field of Health and Nutrition, Education, Livelihood, Research and Monitoring for governments, UN agencies, INGOs and various donor organizations.
Tanjila Mazumder Drishti, who is currently working as a Senior Manager - Advocacy, Knowledge, Communication and Partnership Management of BRAC HNPP, with 9 years of experience in the development sector in leading the operations of various projects catering to urban poor, adolescent girls, youth and RMG workers is also bringing her expertise in project designing, fundraising and partnership management following HCD approach in our team.
Our machine learning team is being led by Ishtiaque Ahmed, Assistant Professor of Computer Science at the University of Toronto. He has extensive experience in putting social justice and sustainability issues in the academic contexts of Human-Computer Interaction (HCI) and Information and Communication Technology and Development (ICTD). He operates through a wide range of technical and methodological apparatuses from ethnography to design, and from NLP to tangible user interface.
Our technology team also includes Saiyeed Mashkur Al Hakim, Head of Technology for Development (T4D) in BRAC Health, Nutrition and Population Program (HNPP), who has 17+ years of software project implementation experience, with strong knowledge in digital transformation and business analysis. At BRAC he is responsible for driving the digital transformation of HNPP; part of this digital transformation involves digitizing daily activity of 5000+ CHW across 61 districts of Bangladesh, the largest digitization project of community health worker program in the world and covers around 78 million people.
To ensure smooth operation and implementation of our mHealth Solution, we have Dr. Imran Ahmed Chowdhury, Programme Coordinator, COVID-19 response in BRAC, HNPP, who has more than 9 years of research and implementation experience with a specific focus on maternal, neonatal and child health (MNCH), health care financing, Nutrition, and Health system change. He has conceptualized, designed, prepared protocols, and managed the implementation of grants totalling over USD 25 million on maternal, newborn child health and Nutrition and COVID-19, focusing on rural populations, including vulnerable and disadvantaged groups.
We have Rubayat Khan, Chief Innovation Officer of mPower, as a MEL expert and Data Scientist specialized in the intersection between scalable low-cost technologies and international development challenges. Rubayat Khan has led a number of mHealth Initiatives and technology innovations focusing on maternal and child health in Bangladesh e.g., mCare, mTikka that ensure more efficient use of data for making timely and targeted interventions.
- Employ unconventional or proxy data sources to inform primary health care performance improvement
- Leverage existing systems, networks, and workflows to streamline the collection and interpretation of data to support meaningful use of primary health care data
- Provide actionable, accountable, and accessible insights for health care providers, administrators, and/or funders that can be used to optimize the performance of primary health care
- Growth
Connection and expertise: Be one of the SOLVER community, we are expecting to get connections and expertise that is highly concentrate on the risk categorization of pregnant mothers for timely and targeted intervention time alongside machine learning
Increased validity and credibility: Becoming a SOLVER will definitely increase the validity and credibility of the proposed ML based approach for the risk stratification of the pregnant mothers to be used by the frontline health workers
Financier outreach: Being a SOLVER will help us to draw the attention of potential impact investors and donors to replicate our solution in other geographic location having similar context to the areas and beneficiaries we are currently serving
BRAC HNPP currently serves 75 million people with a workforce of 4300 frontline health workers (SK). Each of these SKs is covering around 20,000 beneficiaries in their respective catchment area. By incorporating a machine learning based approach for the risk stratification of the pregnant mothers, and delivering targeted intervention to the pregnant mothers according to the risk level they are at, our proposed solution equips the SKs to utilize their time and resources in the best possible way while dealing with such a large number of beneficiaries. Thus using the same number of SKs, our machine learning based approach for risk stratification and service delivery to pregnant mothers will help HNPP to increase both the outreach and quality of the services than they could provide using conventional methods.
As we are working on increasing the efficiency of our machine learning based approach, which is the first of its kind in Bangladesh, other actors in public health sector who have been using static and predefined risk algorithms for symptomatic treatment for pregnant women, can also replicate and built on our proven model to detect risky pregnant cases and provide appropriate interventions for better outcomes. This model can also be replicated in other countries having similar context to Bangladesh to reach and serve more pregnant women by optimally utilizing the minimal resources they have, and distributing resources more effectively to ensure that those most in need get the right attention.
Our ultimate impact goal (5 years) is to reduce the maternal mortality ratio to less than 70 per 1,00,000 live-births in alignment with SDG target 3.1 in the geographies where we operate. There is ample body of research that shows that an accurate risk stratification of pregnant mothers and subsequent timely intervention can lead to significantly improved outcomes on both maternal and child mortality and morbidity.
China executed Five Strategies for Maternal and Newborn Safety (FSMNS) in 2016 that included strategies for assessing and screening for pregnancy risk and managing high-risk pregnancies on an individual basis could decrease MMR by 21.1% from 2013 to 2018 (Liu, et al., 2020). A color coded method used for identifying the risk level of a pregnant mother during ANC visit is credited for successfully reducing maternal morbidity in Malaysia to 28 per 100,000 live deliveries (Rajbanshi, Norhayati, & Hazlina, 2020). A study conducted in Indonesia found that if the risk level of pregnant women could be identified using routine and thorough check ups, many instances of maternal deaths would have been avoided (Diana, Wahyuni, & Prasetyo, 2020).
We aim to do this by more accurately assessing the risk of pregnant mothers periodically throughout the pregnancy using the new ML-based algorithm, and allocating our limited resources to intensify focus on those women to manage their risk better. This can happen through personalized and more intensive counseling for patients and their families, extra support for transportation to facilities for high-risk cases, customized care packages for patients at various kinds of risk, etc.
Our one-year impact goal is to increase the quality and timeliness of counseling, communication and services for women at higher risk of complications, and increase the quality and timeliness of interventions taken for higher risk pregnancy women (such as percentage of facility-based births among the high-risk cohort)
We will use following indicators for measuring our progress towards impact goals:
Prediction accuracy and rates of error (inclusion error, exclusion error, etc.) with ML-based risk prediction as measured against test set - INTERNAL VALIDITY
Prediction accuracy and rates of error in risk prediction as measured against prospective cases - EXTERNAL VALIDITY
Increase in the quality and timeliness of counseling and services provided based on their risk type, rating and stage of pregnancy
Reduced percentage of women who face adverse outcomes.
Our theory of change is predicated on the thesis that an accurate risk stratification of pregnant mothers and subsequent timely intervention can lead to significantly improved outcomes on both maternal and child mortality and morbidity.
Activities:
More rigorous ML approach to risk assessment with more indicators, larger dataset, dynamic rating over time, etc.
Develop intervention protocols based on different risk types, ratings for various stages of pregnancy
Integration of ML risk rating and intervention protocols in BRAC HNPP Tech platform
Outputs:
More accurate and actionable risk rating
Increased efficiency of frontline health workers to identify the patients in immediate need of care
More targeted, personalized and actionable advice and counseling to high-risk women
Outcomes:
Pregnant mothers receive timely and personalized advice and counseling for their particular risk type and level
High-risk pregnant women receive or seek preventive care to manage and mitigate their risk, including more facility-based delivery
Impact:
Decreased mortality of pregnant women and their infants before and during childbirth
Our existing mHealth platform used by BRAC CHWs and facilities, e.g. - BRAC Maternity Centers and Vision Centers is built on a robust open source platform, OpenSRP and currently caters to more than 4500 CHWs and 500 Project assistants across Bangladesh through an android application, locally installed offline supported EMR and an integrated web-portal for management and data visualization. The integrated solution at community and facility levels is used to record longitudinal health data of each beneficiary having an unique ID and taking services from any BRAC health points (CHWs and facilities), stratify the pregnant mothers based on the risk level they are at for targeted and timely interventions, provide personalized counseling based on predefined logics, trigger referral to nearby health centers, manage financial transactions and inventories, take informed decision based on aggregated and granular reporting and track performance of the community health workers.
To utilize an artificial intelligence based approach for risk stratification of pregnant mothers, we have already devised a model using the “Random Forest” algorithm. The model was trained using data from one district only. Upon introducing more data from other districts, we would have to perform more exploratory data analysis followed by experimentation with different machine learning approaches such as semi-supervised/self-supervised learning, reinforcement learning, and time series forecasts using Long Short Term Memory (LSTM) networks, etc. The core of our proposed solution will be powered by an appropriate machine learning algorithm which performs the best on all of the data, ideally a probabilistic model that can quantify predicted risk levels in the form of a probability distribution. Additionally, we will incorporate active learning approaches so that the machine learning model can continuously update itself with newer data and become accustomed to the variations of the maternity records over time.
- A new business model or process that relies on technology to be successful
- Artificial Intelligence / Machine Learning
- Big Data
- Software and Mobile Applications
- 3. Good Health and Well-being
- 10. Reduced Inequalities
- Bangladesh
- Bangladesh
Community Health Workers(CHW), known as Shastho Kormi, collect the primary health care data through an android application. This application is built on a robust Open Source platform, OpenSRP. CHWs go door to door in rural and urban slum areas to register households, people, and deliver primary health care services to them. They are official BRAC employees. Apart from their salaries, they get incentives for providing certain services.
- Other, including part of a larger organization (please explain below)
HNPP Project: To be specific to the HNPP programme, it primarily focuses on equitable access to primary healthcare services to the disadvantaged people, in particular rural pregnant women from disadvantaged and remote hard-to-reach rural areas of Bangladesh, who otherwise would be excluded. To make the programme more inclusive, we mobilize health workers and volunteers, 100% of them are female, from their respective communities.
BRAC: BRAC’s vision is of a world free from all forms of exploitation and discrimination where everyone has the opportunity to realize their potential. BRAC relentlessly works to ensure that everyone has equal opportunity for expressing and using their potential, irrespective of sex, age, race, colour, class, caste, religion, ethnic background, sexual orientation, disability or political beliefs. Gender equality, equity and nondiscrimination are central to all the activities and operations of BRAC. Therefore, BRAC has clearly defined the principles of Gender Equality and Women’s Empowerment (GEWE) and taken appropriate measures to adopt a shared vision to guide BRAC staff in their work with a clear direction to promote gender equality and equity both at organizational and programmatic level. BRAC has also developed an organizational culture that demonstrates BRAC commitments towards diversity, equity and inclusion. Determining the nature, structure, and causes of gender imbalance and inequity in staff throughout the organization, BRAC set targets for recruitment, retention and promotion of staff accordingly, particularly women in senior positions. BRAC encourages women's participation throughout the organization and develops strategies to increase their representation at decision making levels. To reach diversity, equity and inclusiveness targets, BRAC does implement different strategies including various affirmative actions; career development opportunities, proper application of family friendly and flexible working policies etc. BRAC also ensures an equal pay for equal work value for all staff irrespective of sex, gender, religion, race, language, HIV status, disability, class, ethnicity and sexual orientation etc. BRAC also ensures that all the prevention, protection and safeguarding policies of the organizations in place are applied properly.
mPower: The top management of mPower Social Enterprises Limited is committed to managing a culture that values diversity, equity and inclusiveness. Therefore, they had made it a priority to treat all employees equitably, and to create the conditions to make everyone feel welcomed and included in the organization. To incorporate diversity, equity and inclusion in the workplace, the organization already has an established framework, talent management and gender policies in place, which ensures equal employment opportunities for deserving candidates irrespective of races, religions, genders, and regions. mPower clearly communicates these policies and frameworks through induction and training sessions to all of its internal and external stakeholders and thus, ensures that all of them understand and demonstrate attitudes and behaviors that promote diversity, equity and inclusivity in their respective works for and with the organization.
HNPP provides quality, accessible and relevant health services and packages to the marginal communities to both urban and rural areas at an affordable price. HNPP has introduced a community health worker and center-based approach to ensure timely detection of critical health issues and make quality health services available to the clients, while removing the funding as a major barrier from accessing such services.
Community health workers of BRAC HNPP conduct household visits and community level forums to educate people on emerging health issues and diseases. They also conduct rapid tests for pressure and glucose, provide intranatal checkups and refer patients to HNPP health centers if necessary. The services provided by the community health workers include ANC, PNC, NCD/Adult Service, Family Planning Counseling, IYCF/Child, Women Nutrition and Adolescent counseling etc. Apart from the services provided by the community health workers, different health centers of HNPP also conduct different pathology and screening diagnostics at a subsidized rate. At HNPP health centers in urban areas, teams of doctors and midwives are also providing normal deliveries and post delivery support.
- Individual consumers or stakeholders (B2C)
Since HNPP seeks to reduce the challenges of clients from both rural and urban areas with limited income to access health services by providing healthcare services at affordable costs priced lower than the conventional healthcare market, HNPP would like to improve internal efficiency thus reducing overall cost.
Over the years, HNPP ensuring quality of services and focused marketing efforts has seen a rapid service uptake among the target beneficiaries followed by increased revenue generation to recover the cost of the service delivery. A more accurate risk rating of pregnant women will help HNPP ensure better allocation of resources by more targeted interventions on the group of risky pregnant mothers. Thus, a lower exclusion rate will ensure that more revenues are generated utilizing the same resources through targeted services to the most in need.
In our analysis in the pilot phase, it has been observed that the 13% beneficiaries who have been rightly identified as ‘at risk’ have obtained more paid services concerning ANC, PNC and NCD. Rightly identifying the ‘at risk’ patients not only will ensure the health equity for the most vulnerable patients in need of care, but also increase the uptake of paid services by 6%. This will further improve the current cost recovery rate of HNPP.
With a portfolio of numerous programmatic interventions and over a dozen profit generating enterprises, BRAC, regarded as the largest NGO of the world, ensures sustainability through a combination of donor funding and impact investments with clearly defined and financially viable business cases and measurable social and/or environmental impacts.
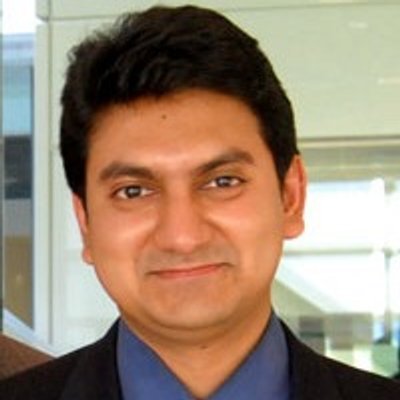
Chief Executive Officer