SMART-CTG
Intrapartum-related disorders are responsible for 25% of the world's neonatal death and contribute to almost 50% of the 2.6 million third trimester stillbirths and are a significant burden to healthcare in low-income countries. In 2010, for example, 1.15 million infants developed neonatal brain damage due to some intrapartum hypoxic events, 90% of these complications taking place in low and middle-income countries.
WHO's recent report on trends in maternal mortality from 2000 to 2017 praised Rwanda for being one of the few developing countries that achieved the highest average annual rate of reduction (ARR) in global maternal mortality ratio (with ARR > 7%). While Rwanda has achieved a remarkable decline in its maternal mortality, a great deal of work remains to be done to meet the Government of Rwanda (GoR)'s target. For example, the GoR's Vision 2050 has the ambition to decrease the maternal mortality rate by 75% and infant mortality rate by 24% by the year 2035. Unfortunately, as echoed by UNICEF's recent report on Rwanda's health budget—due to a shortage of qualified obstetrics and gynecology doctors— such ambitious goals are unlikely to materialize unless the GoR introduces innovations to reduce maternal mortality in the medium and long term. For instance, as of January 2020, Rwanda has a population of around 13 million but only 57 obstetrics and gynecology doctors work in the public hospitals in Rwanda.
In all public hospitals in Rwanda, intrapartum cardiotocography (CTG) is used during childbirth to monitor the heart rate of the baby and the mother's uterine contractions. A CTG graph can help to evaluate the baby's well-being and identify babies at risk of hypoxia during labor and to decide whether the baby will be delivered by instrumental vaginal birth or by cesarean section.
During childbirth, obstetricians visually examine the morphological variations in CTG traces to identify the health status of a fetus. This approach, however, is unreliable and has poor reproducibility because the interpretation is often subjective and varies from one obstetrician to another. Moreover, the fetus's heart rate variability is influenced by the brain's parasympathetic and sympathetic activities and its patterns are affected by several other stimuli such as uterine contractions. Consequently, intrapartum fetal monitoring must also consider a physiological viewpoint instead of just focusing on the morphological appearance of the fetal heart rate. CTG traces contain complex information about the baby's condition and identification of the baby's hypoxemia (e.g., low blood oxygen). Thus, proper CTG interpretation requires the medical personnel to have a good understanding of the physiology of the fetus during childbirth and other compensatory mechanisms of the fetus. Finally, several human factors such as distraction, tiredness, pressure, and stress complicate CTG interpretation and may lead to fatal outcomes. For example, the Royal College of Obstetricians and Gynecologists' report on perinatal mortality in the UK shows that 66% of stillbirths, neonatal deaths, and brain injuries of term babies in labor in 2017 are due to errors of interpretation of CTG and failure to act upon suspicious or pathological CTG.
In the context of Rwanda, due to a severe lack of qualified obstetricians and the large number of deliveries each obstetrician is expected to perform daily, the available CTG machines are often not put to good use and their outputs are poorly interpreted. When CTG interpretation is ambiguous, out of an abundance of caution, obstetricians often resort to unnecessary and costly emergency cesarean sections instead of waiting for a natural vaginal birth.
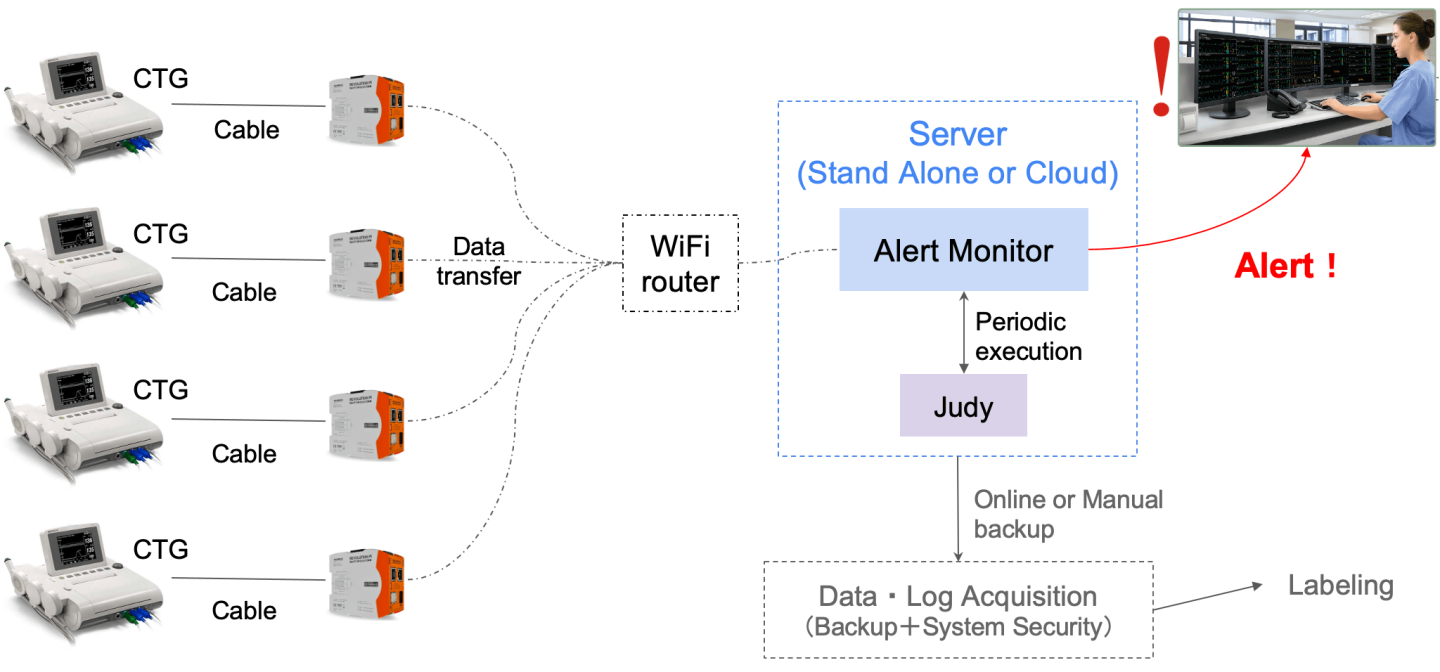
FIGURE. 1. Overview of the system: The device is pluggable into an existing CTG, uses a cheap WIFI router for intranet communication (thus, no internet is required), and provides a centralized monitoring mechanism that allows a single obstetrician to monitor several mothers at the same time on a single large screen.
Spiker Ltd, a startup based in Rwanda, developed a low-cost smart device that is pluggable into an existing CTG and requires no modification to the CTG’s hardware. This device is an Artificial Intelligence (AI) powered clinical decision support (CDS) and analyses the CTG traces in real-time to assess the fetus's well-being. The CDS system requires no internet connection and provides mechanisms to allow early detection of fetal distress and facilitated obstetricians in making a rapid and evidence-informed decision during childbirth. It is also designed to help reduce labor-intensive CTG monitoring tasks; thus, allowing obstetricians to spend time on other high-priority responsibilities. In particular, the proposed CDS system will help in decision-making during labor and will send alerts to medical personnel if an abnormal CTG is detected to expedite investigations of special cases that might need urgent medical interventions.
The device has been designed primarily to serve in hospitals in Rwanda, where, due to a shortage of qualified obstetricians, the available CTGs are underutilized or, worse, their misinterpretations lead to unnecessary and costly emergency cesarean sections instead of waiting for a natural vaginal birth.
In particular, while the device has not yet been deployed in several hospitals, it provided the following benefits in the hospital it has been deployed:
- It achieved a near-human accuracy in interpreting CTG traces
- It allowed one obstetrician to monitor several mothers in a labor ward at the same time and get notifications when any mother needs special attention.
- It has been well received both by the patient but also the obstetricians (including nurses and midwives) who praised it for improving their work and providing an evidence-based interpretation of CTG graphs.
- The hospital's management has also praised the device for increasing accountability and to provide data that drive improvement in maternal wards.
The device is scheduled for a large-scale clinical trial to assess its performance and efficacy and to assess its effectiveness in improving the maternal outcome for mothers and babies. In particular, the device is expected to allow a rapid CTG interpretation during childbirth, improve neonatal outcomes, especially in overcrowded hospitals with few qualified obstetricians, and reduce unnecessary operative deliveries that are often performed when obstetricians misinterpret CTG graphs.
Spiker Ltd is based in Rwanda and its interdisciplinary team (from Rwanda, Japan, India, China and Kenya) possesses various and include researchers, engineers, medical doctors, nurses, and entrepreneurs. The development of the devices closely follows the suggestions of medical doctors in Rwanda and take into consideration technical challenges that are faced by local hospitals. The development phase was preceded by extensive interviews with all stakeholders in healthcare in Rwanda, including policymakers and hospital administrations. The device’s engineering team is also influenced by the feedback it receives from medical practitioners in Rwanda. Finally, the business model is well-aligned with Rwanda’s healthcare sectors, its insurance scheme and the purchasing power of the local population.
- Employ unconventional or proxy data sources to inform primary health care performance improvement
- Leverage existing systems, networks, and workflows to streamline the collection and interpretation of data to support meaningful use of primary health care data
- Balance the opportunity for frontline health workers to participate in performance improvement efforts with their primary responsibility as care providers
- Pilot
Although the development of the device is complete and already deployed in a pilot hospital, we need to expand our services to several hospitals in Rwanda and also introduce the device to other neighboring countries in East Africa. Consequently, there is a need for growth funding to scale the business and mass-produce it to accommodate the demand. There is also a need for funds for marketing and sales and to get the service known and commercialized in other countries.
We propose a low-cost device that automatically analyzes the CTG graphs and provides evidence-based feedback on the health of the baby during childbirth. The device is small, unobtrusive, and pluggable into an existing CTG machine that most medical personnel in Rwanda use and are familiar with. Thus, it requires little to no training, and its installation requires little technical know-how since it seamlessly leapfrog on existing infrastructure.
Moreover, unlike any existing solutions (developed by e.g., researchers in Australia)—which mostly use threshold-based algorithms and hand-crafted features to replicate standards CTG interpretation guidelines—this device use classical machine learning and deep learning (DL) algorithms to improve the accuracy and “intelligence” of the system. In particular, the device follow closely the International Federation of Gynecology and Obstetrics (FIGO)'s guideline on intrapartum fetal monitoring but also provide advanced mechanisms to analyse the impact of the parasympathetic and sympathetic nervous system on the fetal heart rate variability.
The device is expected to provide the following impacts:
- Reduction of unnecessary cesarean section by 20% in the first year and by 50% reduction in the next 5 years. Poor CTG interpretation leads to a high false-positive rate of pathological CTG, which, out of an abundance of caution, obstetricians perform unnecessary emergency intrapartum cesarean sections for fetal distress. Recent studies show that proper CTG interpretation and its use in conjunction with fetal ECG ST segment analysis (STAN) lead to a statistically significant reduction of intrapartum emergency cesarean section and the rate of hypoxic-ischaemic encephalopathy (HIE) due to CTG misinterpretation can be reduced by 50%. In our pilot test, it was found that the device achieved more than 3,000 cases of APGAR (Appearance, Pulse, Grimace, Activity, and Respiration) score greater than 8, and no unnecessary cesarean section was performed when using this device.
- To reduce risks of neonatal seizures by 20% in the first year and by 50% in the next five years. Neonatal seizures are sudden abnormal modifications of a baby's electrographic activity during the neonatal period. It is estimated that neonatal seizures occur in 3 per 1000 live births and are much higher in preterm infants. Neonatal seizure is particularly high in rural low and middle-income countries. For instance, in Kenya's rural districts, neonatal seizures are associated with a 22.5% mortality rate, which is much higher compared to developed countries. Studies conducted around the world show that continuous use of CTG monitoring in labor halved the risk of neonatal seizures, and this reduction is consistent across several subgroups.
- To reduce birth asphyxia by 10% in the first year and by 20% in the next five years. Birth asphyxia (i.e., the insufficient flow of blood or oxygen exchange to or/and from the fetus before, during, or after birth) affects 23% of births worldwide and up to 39% of births at district hospitals in Rwanda. Birth asphyxia is associated with cerebral palsy and long-term impairments such as learning difficulties. The developed device will provide a rapid and accurate interpretation of a cardiotocograph (CTG) during childbirth; thus, allowing rapid identification of pathological CTG that might be associated with birth hypoxia and asphyxia. This will allow the medical personnel to take timely action and appropriate to prevent birth asphyxia.
- To reduce the time and training required to properly interpret a CTG graph. The device is designed to automatically streamline operations and CTG measures (e.g., risk definition, contractions, baseline rate, variability, accelerations, decelerations, and overall impression) that are required to properly interpret a CTG graph. In our test, it was shown that it takes less than 2 days for a novice medical personnel to independently interpret a CTG when he/she used our device.
The success of the proposed solution will be measured based on its impact in hospitals where it has been deployed. Namely, we will measure:
- The impact on the number of c-sections will be measured by comparing the number of c-sections when using the device with the number of c-sections prior to the deployment of the device. The device will go through a one-year large-scale randomized clinical trial in hospitals in Rwanda. The trial, aims, among other things, to assess whether or not using the device reduces the number of unnecessary cesarean sections and to what extent using the device in clinical settings would improve clinical outcomes for the mother and the child.
- Similarly, during the clinical trial, we will use a scoring system such as the Score for Neonatal Acute Physiology with Perinatal extension-II (SNAPPE-II) to assess whether using the device reduces neonatal mortality in neonatal intensive care units (NICU).
- The data collected during the clinical trial will also be used to assess whether using the device reduces the time and training required to properly interpret a CTG graph. This will be assessed by analyzing the delivery room logbooks and comparing them with old data collected prior to the introduction of the device. Additionally, we will keep track of the number of false positive notifications vs false negative notifications and assess whether the notifications are helpful to the obstetricians.
- Apart from these performance metrics, we will also assess the business success of the device. In particular, we will assess the lead management metrics such as the number of new hospitals that adopt the devices per year, and the device's usage engagement metrics.
CONTEXTUAL ANALYSIS
Maternal and neonatal health care was a key component of the United Nations (UN)'s Millennium Development Goals (MDGs) with an objective to reduce the maternal mortality ratio (MMR) by 75% between 1990 and 2015. Although the World Health Organization (WHO) estimates that the MMR decreased by 38% worldwide between 2000 and 2017, this improvement is unequally distributed. WHO admits that up to 94% of all maternal deaths occurred in lower and middle-income countries— with Sub-Saharan Africa accounting for the largest MMR. For example, it is estimated that around 99\% of all maternal-related death occurs in developing countries and that Sub-Saharan Africa alone accounts for 50\% of all these maternal deaths, with 70 to 80\% of the maternal deaths due to direct obstetric causes.
The recently introduced Sustainable Development Goals (SDGs) provide a framework for rectifying this imbalance. SDGs aspire to provide fair access to maternal healthcare for all by reducing the global MMR to less than 70 per 100,000 live births by 2030. Nonetheless, attaining this milestone will be challenging for most low and low-middle-income countries. These challenges include, among other things, a severe shortage of proficient healthcare providers and insufficient training, especially for mid and lower-level providers. Africa, for example, has only 3% of the world's health care workers; yet, bears 25% of the global burden of disease. Consequently, without skilled medical personnel, it is virtually impossible to perform WHO's recommended interventions for improving maternal health.
WHO recognizes that Artificial Intelligence (AI) has an enormous potential to solve several healthcare challenges. For example, deep learning (DL) algorithms achieve human-like accuracy in some diagnostics. Nevertheless, while AI is often utilized to support healthcare in several developed countries, its usage in developing countries remains at the embryonic stage. Moreover, because most AI-based health applications are conceived for rich countries, they are not well-suited and too ambitious to serve their purpose in low-income countries. It is, however, important to note that AI-assisted medical techniques could help improve medical outcomes in resource-poor settings. For instance, in some cases, AI-assisted systems can serve in place of human experts if they are not available— which is often the case in resource-poor hospitals— and provide evidence-based epidemiological recommendations. These intelligent systems would, thus, allow non-specialized healthcare personnel to perform specialized tasks such as diagnosis, prognosis, and treatment of several diseases and only refer to specialists when necessary.
THEORY OF CHANGE NARRATIVE
Intrapartum hypoxia (i.e., oxygen deficiency during birth) emerges before, during, or after birth when a baby does not receive an adequate amount of oxygen. Intrapartum-related disorders are responsible for 25% of the world's neonatal death and contribute to almost 50% of the 2.6 million third trimester stillbirths and are a significant burden to healthcare in low-income countries. In 2010, for example, 1.15 million infants developed neonatal brain damage due to some intrapartum hypoxic events, 90\% of these complications taking place in low and middle-income countries.
In most hospitals in Rwanda, intrapartum cardiotocography (CTG) is often used during childbirth to monitor the heart rate of the baby and the mother's uterine contractions. A CTG graph can help to evaluate the baby's well-being and identify babies at risk of hypoxia during labor and to decide whether the baby will be delivered by instrumental vaginal birth or by cesarean section.
During childbirth, obstetricians visually examine the morphological variations in CTG traces to identify the health status of a fetus. This approach, however, is unreliable and has poor reproducibility because the interpretation is often subjective and varies from one obstetrician to another. When there is a misinterpretation, out of an abundance of caution, obstetricians often resort to unnecessary emergency cesarean sections instead of waiting for a natural vaginal birth. Moreover, the fetus's heart rate variability is influenced by the brain's parasympathetic and sympathetic activities and its patterns are affected by several other stimuli such as uterine contractions. Consequently, intrapartum fetal monitoring must also consider a physiological viewpoint instead of just focusing on the morphological appearance of the fetal heart rate. CTG traces contain complex information about the baby's condition and identification of the baby's hypoxemia (e.g., low blood oxygen). Thus, proper CTG interpretation would require the medical personnel to have a good understanding of the physiology of the fetus during childbirth and other compensatory mechanisms of the fetus. Visually analyzing CTG traces cannot reveal such important diagnostic information and its appropriate interpretation.
Finally, several human factors (e.g., distraction, tiredness, pressure, and stress) complicate CTG interpretation and may lead to fatal outcomes. For example, the Royal College of Obstetricians and Gynecologists report on perinatal mortality in the UK shows that 66% of stillbirths, neonatal deaths, and brain injuries of term babies in labor in 2017 are due to errors of interpretation of CTG and failure to act upon suspicious or pathological CTG.
It is against this backdrop that Spiker Ltd developed an Artificial Intelligence (AI) powered clinical decision support (CDS) system that interfaces to an existing CTG machine and analyses the CTG traces in real-time in order to assess the fetus's well-being. The CDS system provides mechanisms to allow early detection of fetal distress and facilitated obstetricians in making rapid and evidence-informed decisions during childbirth. It is also designed to help reduce labor-intensive CTG monitoring tasks; thus, allowing obstetricians to spend time on other high-priority responsibilities. In particular, the proposed CDS system will help in decision-making during labor and will send alerts to medical personnel if an abnormal CTG is detected to expedite investigations of special cases that might need urgent medical interventions.
The proposed CDS system will also help in decision-making during labor and will send alerts to medical personnel if an abnormal CTG is detected to expedite investigations of special cases that might need urgent medical interventions. In case the hospital is understaffed, it would be possible for one obstetrician to monitor several mothers at the same time and alert a specialist when necessary. Finally, in most hospitals in Rwanda, due to a large number of patients and a limited number of medical personnel, CTG interpretation is often done under great pressure; thus, doctors must make decisions in hurry and while stressed with other responsibilities. Indeed, studies show that working under pressure and in unpredictable settings is rooted in several human factors (e.g., lack of communication, distraction, fatigue, pressure, stress, etc.) that are the root cause of most CTG interpretations. The proposed system is not affected by these human factors and would provide numerical facts and allow the medical personnel to make an evidence-based decision.
The proposed system will be made in Rwanda. Thus, it will be designed to meet the local technological and human resource context. Thus, there will be ample local support and technicians to ensure its long-term operations. Moreover, the proposed system leapfrogs on existing CTG machines that are available in most hospitals in Rwanda. It is pluggable into an existing CTG device. Thus, it is low-cost and requires little training because the local medical personnel is already familiar with its parent CTG machine.
EXPECTED LONG THERM GOALS
- To reduce unnecessary cesarean sections by 20% in the first year and by 50% reduction in the next 5 years.
- To reduce risks of neonatal seizures by 20% in the first year and by 50% in the next five years.
- To reduce birth asphyxia by 10% in the first year and by 20% in the next five years.
- To reduce the time and training required to properly interpret a CTG graph.
The device is pluggable into an existing CTG and uses the International Federation Of Gynecology And Obstetrics (FIGO)'s consensus guidelines on intrapartum fetal monitoring to provide guidance on CTG interpretation. It continuously monitors a CTG trace, records the fetal heart rate (FHR) variability, and extracts several attributes of the FHR such as the baseline heart rate, acceleration and deceleration, uterine contractions, and their timing.
The device uses advanced signal processing and segmentation of the fetal heart rate feature and assesses their correlation with the uterine contradiction in order to help the medical personnel quickly assess and diagnose issues due to fetal distress. The CDS will analyze the raw CTG traces and compute several parameters (e.g., acceleration and deceleration due to the uterine contraction or movements). These characteristics allow to perform common human CTG analysis (e.g., FHR baseline recognition, FHR variability, and acceleration rate). These features will be integrated into an Artificial Intelligence (AI) model to replicate existing guidelines (e.g., the International Federation of Gynecology and Obstetrics [8]) on CTG interpretation. Moreover, the CDS system also uses advanced machine learning logarithms to detect subtle physiological changes in a baby’s heartbeat that a visual inspection cannot detect. Finally, it will alert and provide evidence-based recommendations when abnormal cases are detected.
Three approaches will be used to enhance the performance of the system. Namely, the system will use advanced signal processing and threshold-based algorithms for the human method of CTG interpretation as per the International Federation of Obstetrics and Gynecology (FIGO) consensus guidelines on intrapartum fetal monitoring. Machine learning and deep learning algorithms will also be used to detect complex patterns that are due to the influence of the nervous system on the baby’s heartbeat patterns. Once the models are trained, they are deployed locally and through a REST API for easy consumption. The deployed models are subsequently used to predict the status of the baby during childbirth and give appropriate feedback to the nurse and the gynecology specialists. The system also records the actual clinical decision the medical doctor makes and uses this feedback to improve its future predictions and rectify its mistakes.
The classification and interpretation will follow FIGO's guidelines as shown in Table I and Table II.
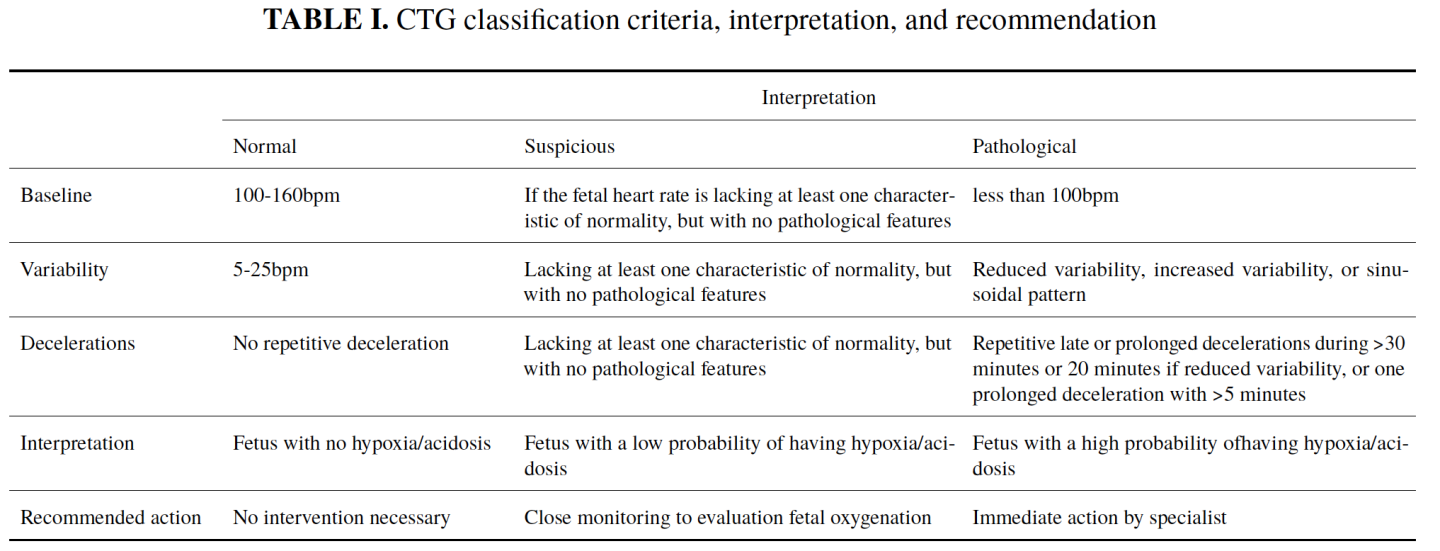
TABLE II. Interpretation of CTG and actions required per FIGO guidelines
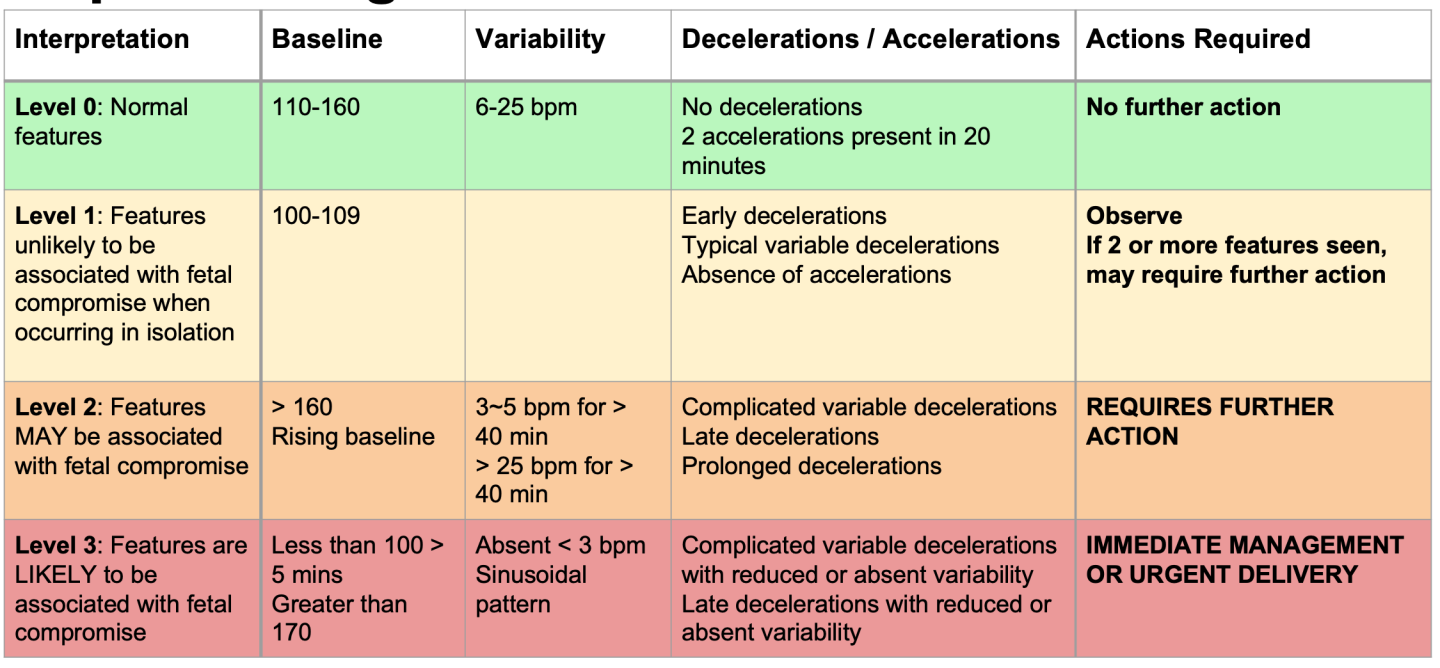
The analysis is provided by three different CTG classifications: normal, suspicious, or pathological.
- Baseline denotes the number of heartbeats per minute considered as a reference for future measurements. It expresses the mean of the horizontal and less oscillatory FHR.
- Variability denotes the oscillations in the FHR on the CTG traces. Normal variability is assumed for a bandwidth amplitude of 5-25bpm. Reduced variability happens when the amplitude is below 5bpm for more than 50 minutes in baseline segments. Increased variability occurs when the bandwidth value exceeds 25 bpm for more than 30 minutes.
- Accelerations refer to the abrupt increases in fetal heart rate beyond the baseline of more than 15bpm and lasting for more than 15 seconds but less than 10 minutes.
- Decelerations refer to the decrease in fetal heart rate below the baseline with an amplitude of more than 15bpm and lasting for more than 15 seconds.
- Tachysystole is the excessive and frequent uterine contraction that occurs more than five times in 10 minutes, in two successive 10-minute periods, or averaged over a 30-minute period.
As shown in FIG 2 below, the device continuously monitors a CTG during childbirth and automatically provides suitable diagnoses and alerts to the medical personnel. In particular, it allows e.g., a nurse to monitor several patients at the same time and can give alerts to remote specialized gynecologists.
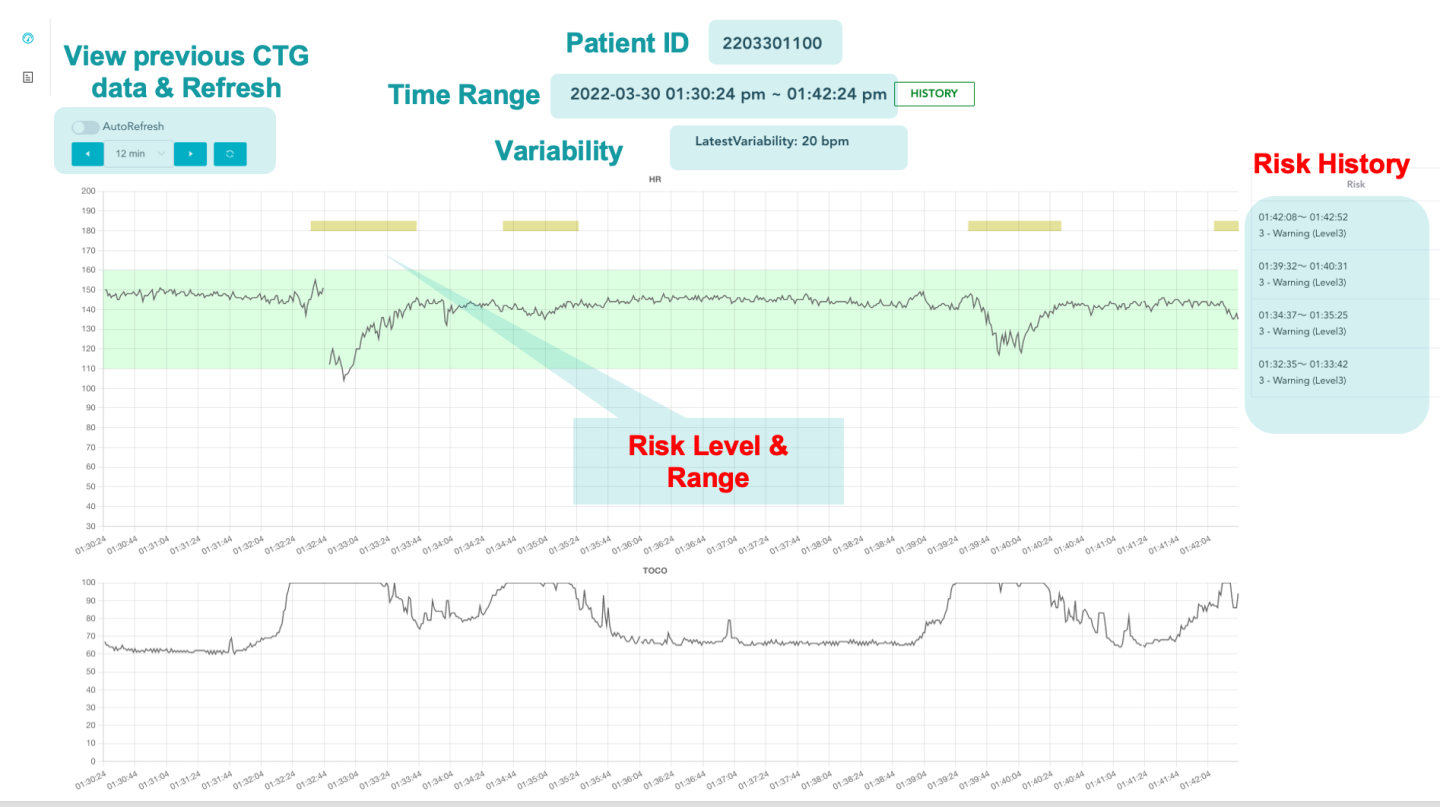
FIGURE. 2. Sample screenshot of the CDS system for a rapid intrapartum CTG interpretation. A baby’s heartbeats are monitored using a CTG machine. The resulting CTG traces are sent to an AI engine. The AI engine uses the International Federation of Gynecology and Obstetrics (FIGO)’s guidelines on intrapartum fetal monitoring and utilizes large datasets of fetal heart rate (FHR) and uterine contraction (UC) features on cardiotocography classified by expert obstetricians to detect abnormal or suspicious fetus and give evidence-based recommendations on the best course of action.
As shown in FIG 3, In the upcoming iterations of the device, the CDS device is expected to implement an end-to-end intelligent CTG interpretation that further enhances its performance. Namely, the system will use advanced signal processing and threshold-based algorithms for the human method of CTG interpretation as per the International Federation of Obstetrics and Gynecology (FIGO) consensus guidelines on intrapartum fetal monitoring. Machine learning and deep learning algorithms will also be used to detect complex patterns that are due to the influence of the nervous system on the baby's heartbeat patterns. Once the models are trained, they are deployed locally and through a REST API for easy consumption. The deployed models are subsequently used to predict the status of the baby during childbirth and give appropriate feedback to the nurse and the gynecology specialists. The system also records the actual clinical decision of the medical doctor makes and uses this feedback to improve its future predictions and rectify its mistakes.
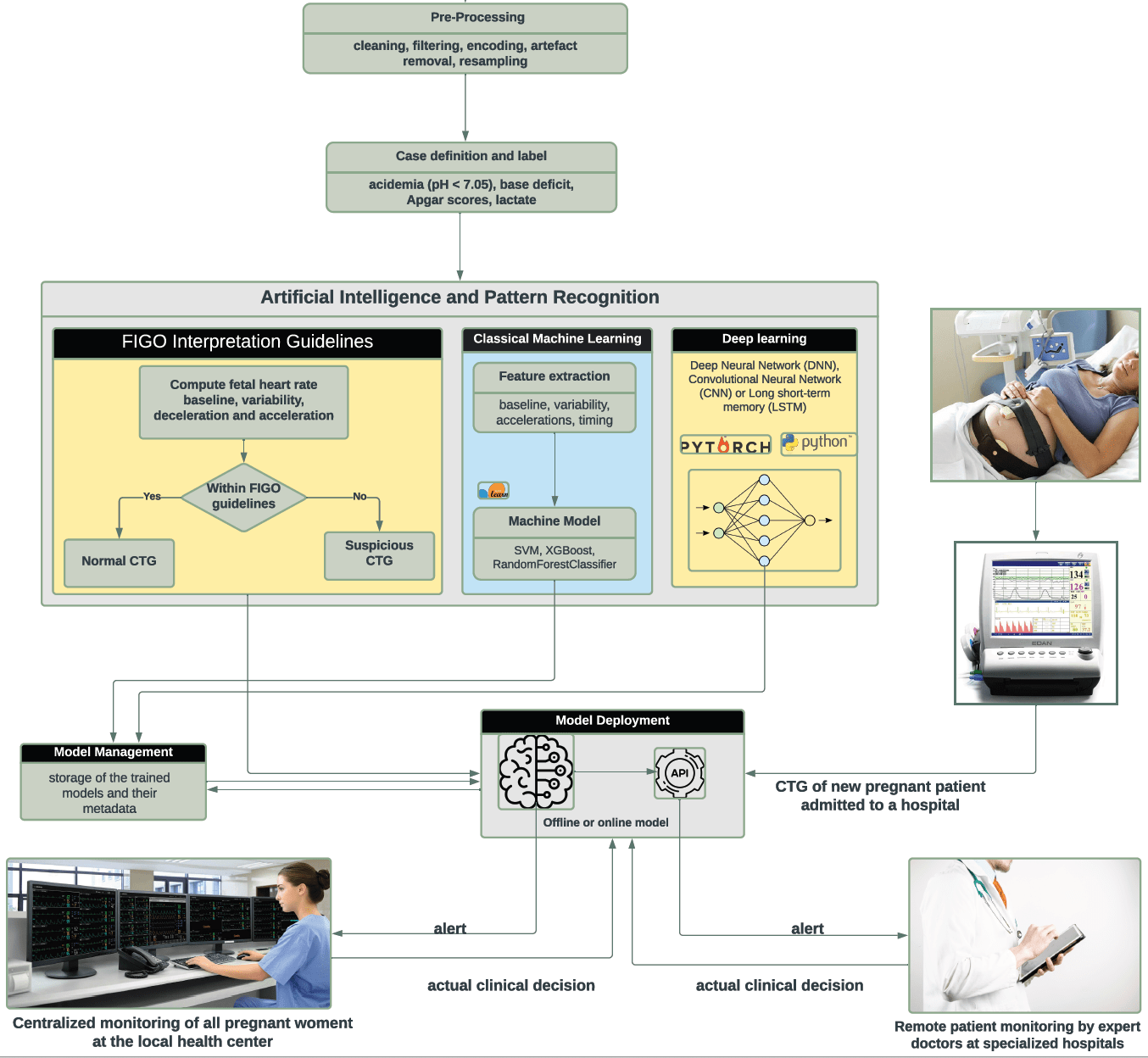
FIGURE 3: Working principle of the completed system
- A new application of an existing technology
- Artificial Intelligence / Machine Learning
- Big Data
- Biotechnology / Bioengineering
- 3. Good Health and Well-being
- 9. Industry, Innovation, and Infrastructure
- Rwanda
- Kenya
- Rwanda
- Uganda
The device collects all the CTG information (e.g., the fetal heart rate (FHR) variability, the baseline heart rate, acceleration and deceleration, uterine contractions, and their timing) automatically and without any human intervention. Other factors that may influence the health of the fetus are assessed by the hospital's medical personnel during a mother's scheduled prenatal visits. During the pilot stage and during the clinical trial, we will hire medical personnel to oversee the clinical trial operation and to keep records of all notable activities and health conditions of all participants.
- For-profit, including B-Corp or similar models
This research is multidisciplinary and will involve computer scientists, engineers, researchers, and community health workers. Thus, the research team includes both male and female researchers. In particular, the project will recruit several female nurses during the clinical trial. Gender balance for this research is primordial for the success of this project, not least because female researchers could help break down social barriers when meeting with pregnant mothers who are due for delivery during the clinical trial but also, they are best positioned to help the research team better understand the best way to address maternal care. After all, this research aims to improve maternal care. Thus, this research aims to address a problem that only women face and they will be involved in all steps of the projects
Spiker Ltd will use a Business-to-Business (B2B) usage-based pricing as shown in FIG 4 below:
- A hospital pays a nominal fee for initial installation and system setup. Depending on the available resources, only the pluggable CDS system is provided and installed into the existing CTG machine. In case the hospital does not possess the necessary CTG machine, the hospital pays around 2,800USD to acquire 4 CTG machines and other accessories required for the complete setup of the system.
- A patient is charged a one-time usage fee of around 10 USD, 20% of which goes to the hospital.
- In this arrangement, spiker also provide training and maintenance of the CDS system.
With this arrangement, in a typical hospital in Rwanda, the initial investment cost could be recovered in less than one year.
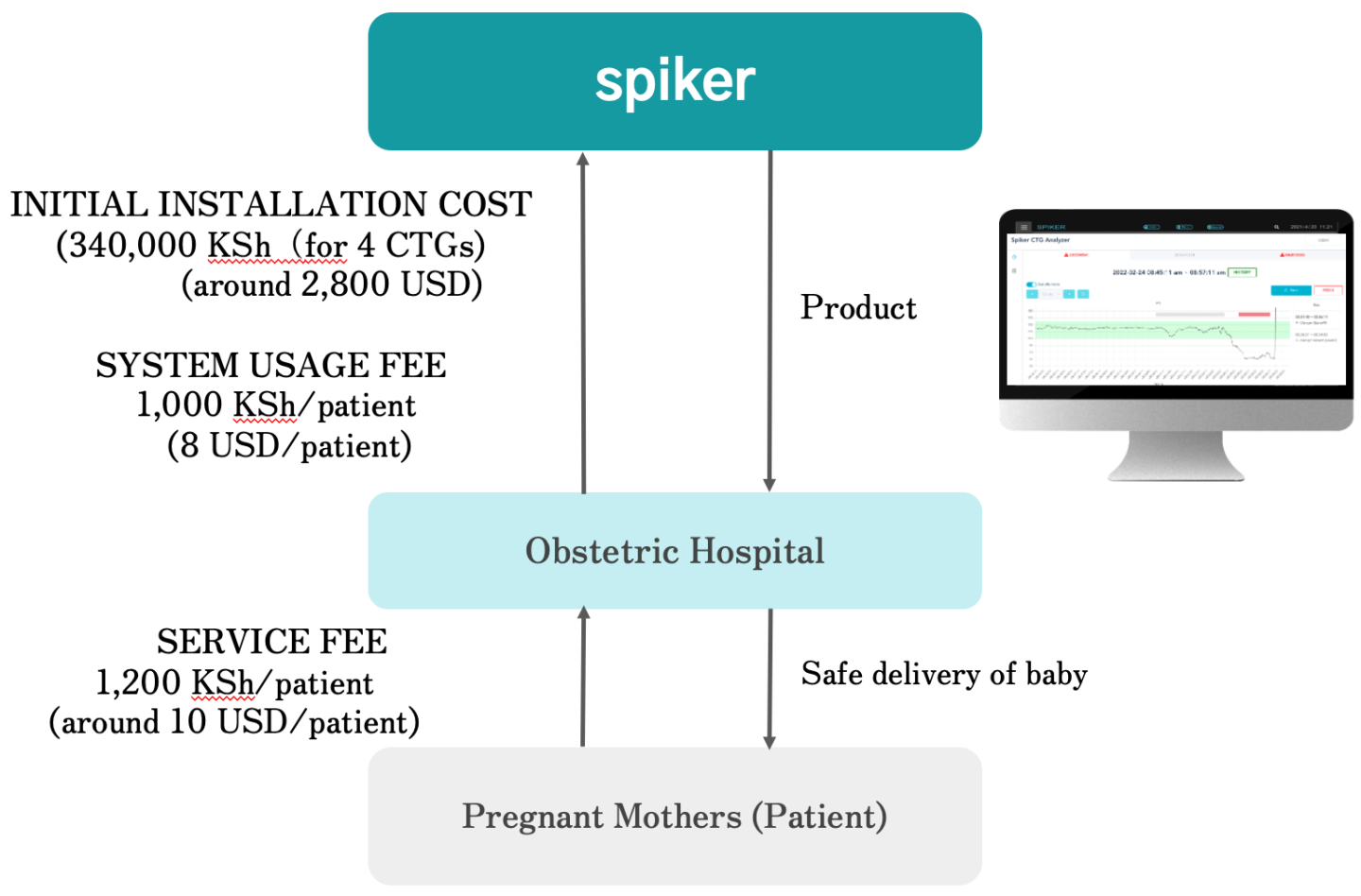
- Organizations (B2B)
Spiker Ltd will use a fee-for-service business model tailored to hospitals. Hospitals will charge a small (around 10 USD/patient) fee to its customer and the hospital will earn a revenue of 2 USD/patient while the remaining 8USD/patient will serve as a revenue stream to our business. Given the large number of deliveries performed per day, our business model will cover all our expenses and allow us to do further research and development and maintenance and customer service for our product.
The proposed CDS system has attracted several positive feedback both from the patients, the hospitals, and governmental institutions. While the device is still waiting for approval from Rwanda's Food and Drug Administration, we have received a couple of international grants to support the development of the system:
- 300,000 USD grant through the Japanese Ministry of Economy, Trade and
Industry in its grants for Subsidy for the Technology Cooperation and Emerging Market Development Project for the year 2022 - In 2021, Spiker Ltd also received a 450,000 USD grant from the Japanese government grants fro Small and Medium Enterprise Agency.
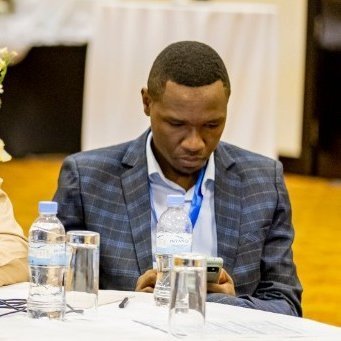
Principal Investigator and Researcher