AI Tuberculosis Screening System
In 2020, an estimated 9.9 million people fell ill with tuberculosis (TB), of which a staggering 4.1 million were left undiagnosed and thus untreated, resulting in 1.5 million lives lost to this curable disease. In fact in 2020 Tb deaths rose for the first time in a decade, due to reduced diagnosis resources during the Covid-19 pandemic. Much like most of the high TB burden countries, South Africa adopts a subjective symptom-based TB screening method that is only 77% sensitive, this means that 23% of TB positives are missed by the TB diagnostic algorithm from the get-go. The resulting delayed or absent treatment extends the patient’s suffering in both time and severity, significantly increase their chance of dying, and causes the patient to remain contagious for longer. In fact, 45% of untreated TB patients die from the disease, patients coinfected with HIV are almost certain to die if left untreated for TB. 80 to 90% of all TB cases are infections of the lungs, this most contagious classification is called pulmonary tuberculosis (PTB).
The TB epidemic also inflicts a significant financial burden on a county’s national health system. The same subjective symptom-based TB screening method, as currently used in South Africa, is only 65% specific. This means 35% of every TB Negative patient screened is sent for expensive bacteriological confirmed testing. In South Africa, that relates to roughly 90% of these expensive TB tests being deemed unnecessary. This costs the already resource-limited national health system both time and money. Much like some of the high TB burden countries, South Africa currently spends 20% of their entire TB budget on final bacteriological confirmation tests alone.
The current TB diagnostic algorithm is reliant on patients self-presenting at the clinic. Due to screening inefficiency, active TB case detection (detection outside the clinic structure) has proven to be too expensive and resource-intensive to warrant the few extra TB positives captured.
Our solution is an Artificial intelligence (AI) enabled, pulmonary tuberculosis (PTB) screening tool, which analyses digital stethoscope recordings to indicate whether a patient should be sent for further TB bacteriological confirmation testing or not.
Our PTB screening system employs two main hardware units, namely the data acquisition device (in this case our digital stethoscope) and a custom local processing unit with a touchscreen user interface called the AIDA. The AI model and operating software are stored and run locally on the AIDA to facilitate offline use. A cloud TB database is updated with the relevant patient data when an internet connection is established, which is used for continuous retrospective model validation and training - (and the future potential to provide breakout warning etc.)
Our solution is a consumable free (and disposal free), portable, point of care (POC) screening tool, with no waiting period for results. Our solution is set to halve the number of TB positive patients currently missed due to the currently employed TB screening tool (as outlined in the problem statement), while at the same time halving the number of TB negatives sent for resource-intensive secondary TB testing unnecessarily.
This screening method upgrade will result in an efficiency gain set to increase the screening sensitivity from 77% to 90% (screening accuracy in TB positive patients). This will roughly halve the number of TB positives currently missed by the system. Earlier diagnosis for these patients will significantly reduce their suffering, it will increase their treatment outcomes while having the potential to reduce the length of their treatment regimes (essential to minimise treatment defaulting). Earlier diagnosis and thus early treatment will also reduce the disease's contagious period, reducing the chance of disease transmission to already vulnerable family members.
This screening method upgrade will result in an efficiency gain set to increase the screening specificity from 65% to 80% (screening accuracy in TB negative patients). This will roughly halve the number of unnecessary expensive bacteriological confirmation tests (currently 90% of tests are done on TB negative patients). This will save nurses much need time while saving the South African TB budget roughly 19.3 million USD annually on direct secondary TB tests.
These screening efficiency gains are likely to make active screening (detection outside the clinic structure) viable, thus ensuring the TB diagnostic algorithm is less reliant on patients having to self-present at clinics, ensuring these currently missed patients are serviced.
Active TB infection strongly correlates to one's socioeconomic circumstances. Unfortunately, being infected with TB perpetuates this situation. The relative household cost of TB is enormous, often removing the primary breadwinner from work for extended periods or permanently. Delayed diagnosis and treatment exasperates this issue resulting in longer patient recovery and reconditioning. Early detection provided by our solution will increase patient integration rate back into society, preserving both their livelihoods and the livelihoods of their loved ones.
The AI Diagnosisis based team has 15 years of medical device development experience.
I completed a BSc in Mechatronic and MSc in Mech. Eng. After which I developed novel heart valve replacements and delivery systems that were designed primarily to serve patients suffering from rheumatic heart disease.
Johan completed his BTech Chem Eng. and MSc in Biomaterials. After working in the medical product development field he switched careers and trained as a data scientist, specialising in AI. He has previously worked on multiple audio interpretation projects.
Mark completed his BSc in Electrical Eng. and has 33 years of experience launching and managing business ventures, the last of which is a care facility for Alzheimer’s patients.
We are aware of our shortcomings as a team and will continue to seek consultation in the areas we lack expertise. Most importantly the team shares a desire to change the circumstances of those in need.
Each team member grew up or lives in areas where the TB prevalence is of the highest in the world. We have all witnessed the perpetuating devastation of TB in the communities around us.
Truly understanding the needs of a community is to become part of it. We have established our research facility in the centre of Mitchell's Plain, Khayelitsha, Philippi, Nyanga, Gugulethu and Delft communities. These marginalised communities are of the most TB burdened communities in the world. All our paid employees come from within the heart of these communities.
I have been lucky enough to spend individual time with 28 different TB nurses in the area, understanding the user's needs. These encounters helped developed our user needs and design inputs.
I have also had the privilege of chatting to numerous TB patients, who have enrolled in our study. Although these conversations are casual in nature and haven't necessarily provided technical input, I've felt their desperation. These conversations act as an abundant source of motivation to ensure that patients don't need to wither away before their TB infections are detected and treated.
- Build fundamental, resilient, and people-centered health infrastructure that makes essential services, equipment, and medicines more accessible and affordable for communities that are currently underserved;
- Prototype
Our solution needs to be used to make an impact. Our solution is designed to fits into and complement the overall diagnostic algorithm. The WHO has developed TB management guidelines for countries like South Africa to follow. Realistically for our solution to be adopted we would need to form part of the WHO's TB management guidelines. We have therefore developed our solution to achieve the WHO's specifically laid out requirements for a TB screening tool. We are now proving our solutions detection accuracy in our post-pilot clinical study. We then intend to conduct an infield clinical trial. I am applying to Solve with the hope that they could partner us with the right clinical/research expertise to guide us in the development of our infield clinical trial. Ensuring it is scientifically sound and sufficient for WHO endorsement. We would also benefit tremendously by having an independent review of our solution giving us credibility when we approach local governments and these international organisation.
- Product / Service Distribution (e.g. expanding client base)
Innovation has been crucial but not the goal itself. Beyond detection accuracy, our product design process has been centred around usability and operating optimally in the bustling rural clinic environments. It is these constraints that has given birth to the usage of existing technology in an innovative way.
Nursing and especially community health worker don’t typically know all the names of the auscultation positions. Therefore AIDA’s Graphical User Interface (GUI), includes an easy-to-use touchscreen, which guides the health worker through the data collection process, indicating when and where to record. Furthermore, an image of an inflating and deflating balloon is used to guide the patients breathing to ensure data consistency.
To accommodate times of load shedding (routine scheduled power outages) or active TB detection fieldwork, the AIDA is fitted with a jetson nano which is perfectly equipped for fast local model inference. The AIDA has a smart rechargeable battery system designed to last more than 12 hours and it is compatible with solar and car battery charging systems.
Our cordless digital stethoscope is fitted with two microphones, one aimed at the auscultation diaphragm and the other pointed outwards to pick up ambient noise. The bustling clinic background noise is digitally subtracted from the auscultation signal prior to the AI analysis step to maximise accuracy.
Image recognition is one of the most researched and advanced sectors in AI. To leverage this established technology, we convert the auscultation recordings (sound files) into a spectrogram (a frequency domain visual representation of a sound file). We then use established image analysis neural network architecture to analyse these spectrogram “images”.
Our TB data collection has been pivotal to developing our solution. To overcome challenging COVID-19 restrictions and funding constraints we have needed to innovate in this area as well. We developed a digital data collection system, with synced secure cloud-based data backups. Furthermore, we have developed an automatic participant booking system (in three languages), where potential participants initiate contact by WhatsApping ‘hi’, they are then automatically checked for eligibility on the National Health Laboratory Service (NHLS) and booked an appointment on google calendar.
Our goals for the year are to complete product development and the infield clinical trial.
Within the next 5 years, our goal is to have rolled out across South Africa and have started rollout across the other 8 highly TB burdened countries.
Our primary impact goal is to halve the number of TB positive patients missed by the current overall TB diagnostic algothithm. Our secondary Impact goal is to halve the number of TB negative patients samples being sent for lab tests.
Halving the number of Tb positives missed will significantly reduce patient suffering, it will increase their treatment outcomes while having the potential to reduce the length of their treatment regimes (essential to minimise treatment defaulting). Earlier diagnosis and thus early treatment will also reduce the disease's contagious period, reducing the chance of disease transmission to already vulnerable family members.
Halving the number of unnecessary expensive bacteriological confirmation tests (currently 90% of tests are done on TB negative patients) will save nurses much need time while saving the South African TB budget roughly 19.3 million USD annually on direct secondary TB tests.
These screening efficiency gains are likely to make active screening (detection outside the clinic structure) viable, thus ensuring the TB diagnostic algorithm is less reliant on patients having to self-present at clinics, ensuring these currently missed patients are serviced.
Active TB infection strongly correlates to one's socioeconomic circumstances. Unfortunately, being infected with TB perpetuates this situation. The relative household cost of TB is enormous, often removing the primary breadwinner from work for extended periods or permanently. Delayed diagnosis and treatment exasperates this issue resulting in longer patient recovery and reconditioning. Early detection provided by or solution will increase patient integration rate back into society, preserving both their livelihoods and the livelihoods of their loved ones.
We plan to achieve them by replacing the current TB screening tool with a system that is ~90% sensitive and ~83% specific (Pilot results).
Maximising the Capture Ratio and Efficiency Ratio will be the main metric indicators of success. (linked to detection sensitivity and specificity)
Capture Ratio: Number of bacteriological confirmation tests conducted on TB-Positive patients vs the WHO's national TB incidence rate.
Efficiency Ratio: Number of bacteriological confirmation tests conducted on TB-Positive patients vs Number of bacteriological confirmation tests conducted on TB-Negative patients.
Root Problem:
- Inaccurate TB screening methods.
- Sensitivity > 77% -> 23% positives missed
- Specificity > 65% -> 35% Negatives sent for TB tests unnessearially
- Reliance on TB patient's self-parenting at the clinic.
- The socio-economic living conditions
Actions (inputs):
Develop and rollout new TB screening method. Main technical requirements:
- Sensitivity > 90%.
- Specificity > 80%.
- Portable.
Link up with contact tracing and active screening initiatives.
Results (Outputs):
Increased Sensitivity -> Halve the number of TB positives currently missed -> Earlier TB detection.
Increases Specificity -> Halve the number of TB negatives sent for lab tests -> Reduce TB resource waste.
Portable (and accurate) -> Non-Clinic based active TB screening technically possible and financially viable -> Non-Clinic presenting TB positives identified earlier.
Outcomes/Long term goals:
Early detection -> earlier treatment -> better treatment outcomes -> Improved health.
Non-Clinic presenting TB positives identified early. -> earlier treatment -> better treatment outcomes -> Improved health.
Earlier treatment -> reduced contagious period -> reduced spread of the TB -> Improved community health.
Reduce TB resource waste. -> Redirection of TB budget to medication adherence and other health necessities -> better treatment outcomes -> Improved health.
Improved health -> Fewer costs & ability to work -> better financial outcomes.
Ethos:
Equity in Medical Care
The AI model in our solution core technology. First creating the correlation between stethoscope recorded audio signitures and pulmonary TB (model training) then, detecting TB in new patients (inference).
The AI model used is a residual neural network architecture. We use ResNet34 in particular. This architecture allows for the training of exceptionally deep neural networks, making it extremely well suited for image classification tasks. We've leveraged this fundamental breakthrough by converting our lung sound files to image-like spectrograms using a Fourier transformation. We then do the training/inference on these "images" (Converted audio recordings).
- A new application of an existing technology
- Artificial Intelligence / Machine Learning
- Imaging and Sensor Technology
- Internet of Things
- 1. No Poverty
- 3. Good Health and Well-being
- 10. Reduced Inequalities
- South Africa
- South Africa
- For-profit, including B-Corp or similar models
Diversity is essential to minimize biases and blindspots. We as the leadership group recognise the importance of this. It is our intention to ensure we developed a diverse and representative team as we grow.
Our business is centred around creating equity in medical care, we plan to mirror this ethos in our hiring policies.
Customer: National Department of Health - TB management programs.
Beneficiaries: TB patients.
User: TB Nurses Community Health Workers.
Business model (Values based on the South African market only).
Per unit license fee revenue model. We maintain hardware ownership - facilitating national adoption due to no capital requirements.
Customer Benefits: (based on TB detection accuracies achieved during the pilot study 80% and 83% sen and spec, respectively)
- 23K more TB positives captured P.A
- USD 19.3M saved on unnecessary secondary TB lab tests.
- USD 10M net saving for the DoH, after the cost of service, has been subtracted.
User Benefits:
- Halve the number of sputum sample collections from TB negative patients req.
- Empowered, extra diagnostic capability.
User Benefits:
- Detected earlier, treated earlier, improved treatment outcomes.
- Government (B2G)
We intend to complete the product dev. using funds raises through investment capital and grants.
We then intend to charge the state a monthly per-unit license fee to use our product, the license fee of ~USD 200 is sufficient to overtime cover the operational costs and cover developmental expenses incurred and to pay back investors with interest. Our product induces a direct gross state saving which is double that of our entire cost of service.
The co-founders have provided the sum of development capital up until now.
So far our success has been on the other end, minimising cost.
We have developed automated WhatsApp based booking systems to manage participant eligibility screening and enrollment (saving on time and salaries). We've worked with consultants on "out of expertise" problems instead of taking on permanent employees (lean). We have leveraged the state's nursing network for participant recruitment. The unpaid leadership team is primarily technically based and so the hardware prototypes, operating software, AI model etc. have all been developed in house.
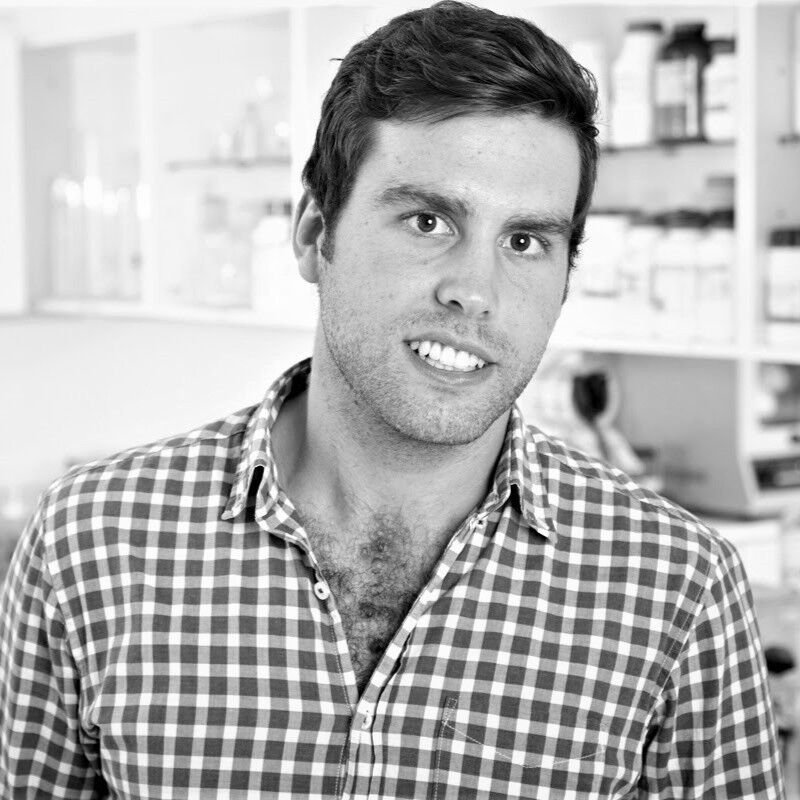
Mr