Global Tuberculosis AI Initiative
Worldwide in 2020, 10 million people developed tuberculosis (TB) and 1.5 million died according to the World Health Organization (WHO) estimates. Deaths are thought to have risen in 2021 due to the COVID-19 pandemic and interruptions and delays in care, more in developing countries. It is thought that 30% of cases may go undiagnosed. To reduce the global burden of TB and achieve the WHO objectives of a 80% and 90% reduction in TB incidence and mortality by 2030, more patients with TB need to be identified and treated, promptly and systematically.
The WHO has recommended that computer-aided detection (CAD) be used for screening and triage of TB, when expert radiologists are not available, for those 15 years of age and older. Artificial intelligence techniques have developed to allow for more accurate image recognition capacity to identify TB based on plain films.
In low resource settings, TB may have different radiologic presentations, which may affect the validity and replicability of algorithms developed with datasets from resource-rich settings. Artificial intelligence is only as ‘intelligent’ as the data used to train it. Many datasets on which machine learning (a form of artificial intelligence) tools were trained come from more resource-rich settings, in North America, Europe, and Asia, which will not represent the realities in lower resource settings. Therefore, the usability of such technologies is limited in lower resource settings.
The tools in use also are intended for screening in healthy populations and we want to ensure clinicians have more tools to identify sick TB patients from sick patients with other pulmonary diseases. We also want to engage clinicians, who often have access to fewer digital tools, by using Chest Xray images to build CT scans, to allow for better visualization of the disease. We also are looking to ensure the tool can identify different types of TB, as this can change management.
To face these challenges, the Global Tuberculosis AI Initiative comes from over a decade of work on healthcare in Haiti and on TB by two of the project members. It comes from many conversations with colleagues working on tuberculosis and radiology in Haiti and the aim is to ensure that it develops a usable technology that benefits clinicians and patients on the ground.
We propose an AI-powered application to screen for tuberculosis in resource-limited settings based on plain chest radiographs. At this stage, we have developed tools to better allow AI to meet the needs of clinicians and patients in low resource settings. We are working to ensure the datasets the tool is built on reflect the populations affected by TB, which are often younger and more likely to have prior TB or HIV, which can affect the tools. We also want to ensure the tool can help not just in screening healthy populations, as other tools have focused on, but in better diagnosing TB among those with sick with TB or another pulmonary disease, reflecting a true need in this context.
We are looking to build the technology as an app and test with providers the usability and accessibility of the technology. We would work with clinicians, seeking their feedback, as well as patients, to see how the technology works in real life. Our goal is to reduce bias in global healthcare systems, and make the data used by artificial intelligence algorithms more representative of the people who will use the tool.
Our solution targets people who live in low-income countries. At this stage, we specifically target the Haitian population. Haiti is a country of 12 million inhabitants, with a GDP of $846 per capita, of which health care spending made less than 10% in 2018. Port-au-Prince, where the project will start, is the most populated city (more than 2 million inhabitants). In 2018, Haiti reported the highest incidence of tuberculosis in the western hemisphere, with 176 cases per 100,000 inhabitants. However, radiology services are limited. After the 2010 earthquake, the entire country only counted 19 attending radiologists. Therefore, the identification of patients with TB typically based on interpretation of chest radiographs, and ensuring diagnosis confirmation and initiation of treatment have been limited. Following the World Health Organization's recommendations, an artificial intelligence-based solution is critically needed to detect and contain this deadly disease in Haiti.
The Global Tuberculosis AI initiative was born from over a decade of work on healthcare in Haiti and on tuberculosis by two of the project members. The project comes from conversations with colleagues working on tuberculosis and radiology in Haiti. Furthermore, the staff comprises Haitian clinicians, public health and clinical researchers, and AI experts and researchers and includes collaborations from colleagues in Africa. The team includes Dr Elena Sizikova who has been been designing the tool, with colleagues, as well as Dr Megan Coffee an infectious disease physician, who first started working on TB in Haiti in 2010 and has worked on other AI tools for infectious diseases and has been working with colleagues in West Africa interested in exploring the tool further. Therefore, we are constantly communicating with our collaborators in Haiti, Ghana, Nigeria, and Sierra Leone to ensure that the project is feasible and accepted and responds to the needs of these communities.
- Build fundamental, resilient, and people-centered health infrastructure that makes essential services, equipment, and medicines more accessible and affordable for communities that are currently underserved;
- Prototype
We are seeking Solve's support to reach our goal of developing the Global Tuberculosis AI initiative in our targeted communities at no charge for the recipients.
- Legal or Regulatory Matters
Our goal is to trial a viable product in our targeted communities by next year. And assist health systems in lower resource settings in harnessing the power of artificial intelligence to alleviate the burden of tuberculosis by rapid screening.
Our solution is powered by machine learning, a type of artificial intelligence. We propose shape induction, that is, learning the shape of 3D CT from chest X-Ray without CT supervision, as a novel technique to incorporate realistic X-Ray distributions during training of a reconstruction model.This technique, built upon the system proposed in X2CT, aims to improve both the perceptual quality of generated CT and the accuracy of downstream classification of tuberculosis.
- A new application of an existing technology
- Artificial Intelligence / Machine Learning
- 3. Good Health and Well-being
- 10. Reduced Inequalities
- Haiti
- Ghana
- Haiti
- Nigeria
- Sierra Leone
- Nonprofit
Diversity is a core value of the Global Tuberculosis AI Initiative. Our team develops and supports an equitable work environment where we value each collaborator's historical, social, and cultural integrity across many different countries. A Haitian physician-researcher leads the team, and our collaborations spread across diverse backgrounds. Moreover, our project aims to foster more inclusion and equity in the artificial intelligence world by centering on those most afflicted by tuberculosis who will use the tools we develop.
The Global Tuberculosis AI Initiative strives to provide an AI-powered application to clinicians and patients to help screen for tuberculosis and diagnose the disease in the most afflicted populations, free of charge. We aim to partner with academic institutions, governments, and start-up companies in the communities we target. Their collaboration will go towards research, development and implementation that will diminish the burden of tuberculosis across the mainly affected regions.
- Organizations (B2B)
To become financially sustainable, we will develop long-term partnerships with academic institutions, university hospitals, governments, and start-ups to fund our research, development, and implementation and lower production costs through regular donations and grants.
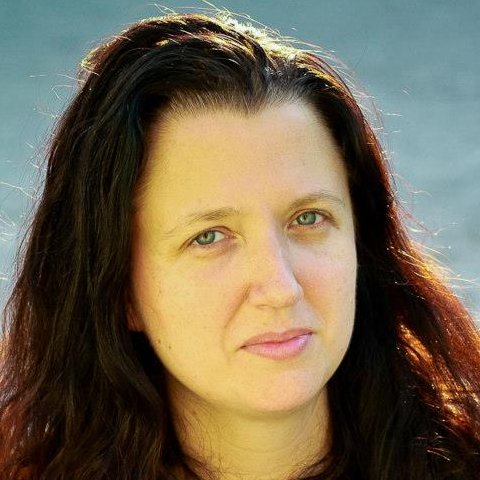
Director
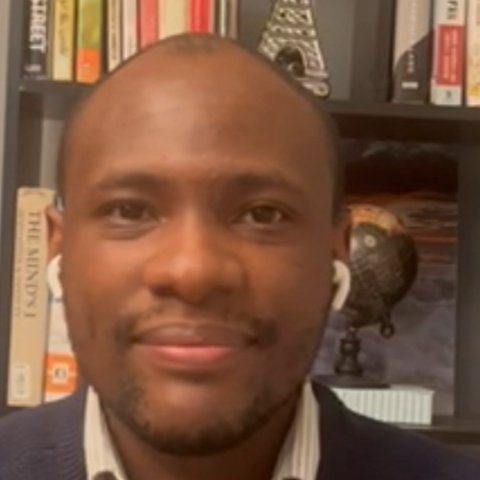
Public Health Researcher