SensitiveNets
A new agnostic biometric representations capable to lead the best performance, transparency and trust on biometric systems
Discrimination can be defined as the unfair treatment of an individual because of his or her membership in a particular group, e.g. ethnic, gender, etc. The right to non-discrimination is deeply embedded in the normative framework that underlies various national and international regulations, and can be found for example in Article 7 of the Universal Declaration of Human Rights, and Article 14 of the European Convention on Human Rights, among others. Algorithms have an increasingly important role in decision-making in several processes involving humans. These decisions have therefore increasing effects in our lives, and there is an increasing need for developing machine learning methods that guarantee fairness in such decision-making.
Researchers from MIT, Oxford, Michigan State University and University of Maryland have demonstrated that biometric technologies are permeable demographic information such as gender, ethnicity or age. Their studies have demonstrated that different ethnic groups receive differential treatment. These unfair treatments increase the gap between classes and promote a not inclusive technology.
We have developed a new patented agnostic feature representation capable to remove sensitive information from the decision making of automatic processes. The resulting networks trained with the proposed representation, called SensitiveNets, can be trained for specific tasks (e.g. image classification, face recognition, speech recognition, …), while minimizing the contribution of selected covariates, both the task at hand and in the information embedded in the trained network. Those covariates will typically be source of discrimination that we want to prevent (e.g. gender, ethnicity, age, context).
SensitiveNets removes sensitive information from the decision-making of biometric systems. Our technology allows the accomplish of the new legislation to guarantee rights of citizens and promote fairness in biometric systems.
- Prototype
Biometric technology has a great potential to improve identity management systems all over the world. There are numerous ongoing initiatives involving billions of citizens. However, there is an increasing demand of transparency and accountability for these technologies.
SensitiveNets is a new technology that allows training discrimination-aware biometric recognition algorithms. We have developed a patented learning process capable to remove sensitive information (e.g. ethnicity, gender, age) from the most popular biometric representations. SensitiveNets is a new technology developed to lead a new generation of biometric systems focus on civil rights, transparency, and trust.
Our technology has been developed to guarantee fairness, transparency and accountability on the decision-making of biometric recognition systems. SensitiveNets generates new representations trained to remove sensitive information from biometric templates. SensitiveNets tranforms the feature space of traditional biometric representations. SensitiveNets is compatible with feature representations based on deep-learning architectures and it can be applied to existing technologies or new algorithms. These transforms are compatible with the most popular encryption technologies adopted by ISO/IEC standards.
SensitiveNets are compatible with the most popular biometric template representations. The sensitive information removal is based on an iterative learning process. This learning process results in a linear transformation trained to remove such sensitive information.
SensitiveNet can be incorporated to the most popular biometric recognition technologies based on deep learning architectures (e.g. face, iris, speech, etc…). To incorporate the technology it is not necessary to re-train the models. The sensitive information removal can be applied either pre-trained or new modes.
SensitiveNets is transparent to the final user. However, this technology guarantee a fair treatment of citizens. We have evaluated the technology on the challenging face recognition systems. These systems have to deal with several covariates including: different poses, illumination changes, orunconstrained acquisition. The results demonstrate the usefulness of the agnostic representations.
SensitiveNets are applied to transform the feature representation of existing biometric technologies and new ones. The learning process is based on two-steps: 1) training the new representation based on the biased features and the sensitive information to be removal; 2) apply the learned transformation to the feature templates.
The learning process developed is data-driven. The feature transformation is applied into the embedding space. This transformation is learned specifically for each biometric system and the learning process determines it. Interoperability is ensured for systems using the same feature representation. However, different feature representations (e.g. face recognition algorithms based on different deep architectures) need their own learned transformations. SensitiveNets include a set of libraries to perform the sensitive information removal.
Not applicable for this technology. SensitiveNets are transparent for the final users of the biometric systems. SensitiveNet can be integrated in existing biometric recognition technologies.
The right to non-discrimination is deeply embedded in the normative framework that underlies national and international regulations. There is an increasing concern about algorithmic discrimination and its effects on citizens. New regulation such as EU GDPR includes specific articles. According to paragraph 71 of GDPR, data controllers who process sensitive data have to: “implement appropriate technical and organizational measures to prevent, inter alia, discriminatory effects”.
It is necessary to develop a new generation of biometric technologies capable to guarantee fair and transparent decision-making. SensitiveNets can play a key role on this new generation of biometric systems.
- Academia/Research
- Academic/Researcher
- 6-10
- 3-4 years
This technology was developed by the Biometrics and Data Pattern Analytics - BiDA Lab from Universidad Autonoma de Madrid.
BiDA-Lab has more than 20 years experience on biometric technologies. BiDA group have contracts with private companies and national agencies to develop new biometric recognition solutions.
The members of BiDA-Lab are authors of more than 100 articles published in top international journals, more than 300 papers in International Conferences (with several best paper awards), and more than 25 international book chapters (altogether more than 12,000 citations in Google Scholar).
Continued participation of the group in a series of competitive projects of the National and European Projects and Actions, focusing on biometric recognition, always with excellent ratings in the evaluations of the proposals and the results obtained.
Licensing agreement and R&D development agreement.
We consider necessary the development of this technology together with both industry and public agencies. New regulations are need in order to protect citizens against new sources of discrimination and new technologies are need in order to prevent it.
The market is global. SensitiveNet can be applied to the most popular biometric representations. For example face recognition is a $9.6 Billion market. There is an increasing need to develop transparent biometric technologies and new regulations start to rise. SensitiveNets was developed to lead this new generation of biometric systems.
Algorithmic discrimination is an important concern attracting an increasingly interest by public agencies and private companies. Citizens must be informed about their rights against these new types of discriminations. Mission Billion Challenge is an opportunity to shift the spotlight to these new sources of discrimination.
Biometric community must improve transparency and trust on biometric technology. SensitiveNets is the first of several initiatives to come. A new generation of biometric recognition systems is needed and Mission Billion Challenge will be our first stop.
Existing and new regulations must be adopted. Awareness on this topic is necessary. There is a need of information. Most of citizens unknown that biometric technologies can be discriminant.
Public agencies, private companies and researchers need to collaborate to promote a new transparent and discrimination-aware biometric technology.
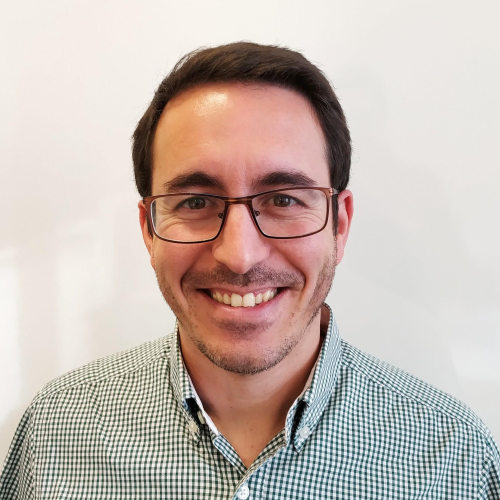