A Novel AI powered Smart IoT Wildlife Warning System
This AI power IoT solution can both warn drivers of animal presence and collect wildlife activity data which solve both safety and conservation challenges in combating roadkill.
The Smart Wildlife Warning Sign(SWWS) is an AI powered IoT system designed to warn drivers of wildlife road-side presence and concurrently collect wildlife activity data for ecological conservation. When animal presence is detected, the warning sign sends out a traffic warning signal and the on-board trap camera simultaneously activates to snap a picture of the environment to scan for the wildlife in question. Using a pre-trained machine learning model, the animal in view is classified on-site.
Identified wildlife species combined with image metadata such as location and time are saved to a database file that is offloaded wirelessly via a drop-off mobile app system. Wildlife activity is then used to merge with other data collection to create an adaptable network of information, providing valuable insights such as wildlife activity hotspots, changes in population, and predicts migration patterns for effective conservation infrastructure placement.
Along America’s highways, roads cross through the natural habitats of many native wildlife species. As we continue to expand and build new infrastructure, it’s inevitable that we run into conflict with existing nature. Out of the 10.6M km of roads in the US, 59% of Roads are NOT traversable by animals. Thus, when these man-made paths intersect with animal migration, roadkill collisions occur. This presents a real danger to both human safety as well as wildlife survival which results in ecological deterioration, severely hurting the livelihood of wildlife species living in low densities.
According to the crash analysis report of the Oregon Department of Transportation, there were 1,250 large animal-related collisions reported in Oregon for 2014. It’s estimated that 1.25M large animals are killed by vehicles across roads in the US and 26,000 wildlife related collision cases of critical human injury annually. In addition, with each animal-vehicle collision costing $7,890 on average, there’s an estimated $1.1billion in property damage from these accidents.
Roadkill has become a serious problem that endangers both animal and human livelihood.
After talking to park rangers and road ecologists, I learned that tracking wildlife activity has been an extremely labor-intensive task. Existing wildlife monitoring systems and road infrastructure for roadkill prevention are insufficient and inefficient. Further, they are either incapable of scaling or physically exhaustive, severely limiting the active impact such solutions could have.
The SWWS is a way to solve both challenges in developing active animal warning systems and wildlife monitoring networks: pushing AI towards deployable devices that can both warn drivers of animal presence and collect wildlife activity data.
Current solutions are all passive which have clearly under-represented the challenge at hand. With traditional signage, short reaction times for drivers and poor visibility at night or with bad weather still result in dangerous collisions.
In order to prevent roadkill collisions from occurring there must be an active warning system that’s able to warn drivers ahead of time. SWWS aims to bridge this gap by using infrared heat-sensing and radar-based depth detection to incorporate a field-sensitive vision for fast and accurate animal detection.
Live collision prevention helps save the lives of both drivers and wildlife.
Consequently, wildlife monitoring today is limited to tagging efforts and observational sightings which is only a peak into the black box. We can only estimate where they might move. When planning for new overpasses or fencing, ecologists are only able to guess at where infrastructure is needed. We’re limited to chasing wildlife conservation with limited information and funding. Big data helps cover this need.
Whilst warning drivers for animal presence SWWS simultaneously captures images of wildlife nearby in order to identify wildlife hotspots and map migration patterns. These valuable clues into wildlife activity can be used for road ecology research and surveys and plan efficacious conservation infrastructure.
Over the course of the past 5 years, this project has been continuously prototyping and revising designs in order to best address challenges in roadkill prevention and wildlife conservation.
Beginning with talks with the ODOT and ODFW, preliminary designs were advised under the supervision of road engineers and ecologists.
Taking sensing technology and computer vision for wildlife monitoring the next step forwards, the SWWS was then provided guidance from Portland-based WildMe conservation group. Over the course of the 2018-2020 development season, the SWWS project continued collaboration with WildMe and applied for incubation programs at Conservation X Labs and MIT Think in order to advance prototype capabilities through resources and mentorship.
Maturing AI capabilities and rounding off a fourth prototype in 2021, the SWWS began field-testing at the Tualatin-River National-Wildlife-Reserve. Since then, the prototype has been continuously deployed for performance testing and off-grid capabilities on a monthly basis and begun mentorship at the UC Davis road ecology lab.
During this engineering period, discussion has continued with organizations involved to incorporate input from ecologists, road engineers, computer vision developers, and wildlife conservation workers. It’s this project’s hope that it can best serve human and animal populations, ecological research efforts, and wildlife conservation by collaborating with it's target audience of researchers and engineers.
More recently, beta-testing for warning signals, sensor technology, and migration pattern mapping involved in the SWWS and it’s mobile app ecosystem are being advised by previously mentioned organizations.
- Other: Addressing an unmet social, environmental, or economic need not covered in the four dimensions above
With wildlife and biodiversity all around the world suffering as a result of human impact, it’s more important than ever to step up and fight for greater environmental justice. One such is the devastating problem of roadkill and vehicle-animal collisions. As a direct result of our human expansion, it’s also our duty to best protect existing wildlife populations our traffic is impacting. In addition, road networks become abiotic barriers to wildlife migration between regions creating ripple effects on ecosystems. Among millions of animal-related traffic accidents occurring annually in the US alone, not only are these collisions dangerous to the ecological well being of wildlife populations, they also have a sustained impact that reaches even our own livelihood.
Current implementations addressing roadkill collisions are ineffective, meaning that there is a significant need for smarter solutions to step up to the challenge and fully address ecological issues using the power of new technologies such as the Internet of Things and AI.
- Prototype: A venture or organization building and testing its product, service, or business model
Currently, the SWWS project is in a prototype phase where features are being tested and continue to be matured in order to advance the solution into an implementable and reliable real-world applicable system for deployment. Field-testing results are being used to continue in the improvement of existing features and incorporate new approaches. Although the state of the project is close to closing out the prototyping design period, it is not yet a complete product and may still take up different forms and features in order to best address target users/beneficiaries.
- A new technology
The smart wildlife warning system involves numerous technological components but focuses primarily on the melding of IoT hardware and AI-based deep learning software to adapt to diverse challenges when working in road ecology. In order to detect roadside wildlife presence, the SWWS relies on smart infrared and radar-based sensor technology. Simultaneously warning drivers, the system then takes images of the surrounding environment in order to classify wildlife species present through AI-based deep learning models. To transmit it’s collected information for further analysis, the SWWS relies on direct wireless connectivity in the field to transmit it’s database files via mobile app. Incorporating behavioral clues through working with big data in wildlife species, time, and location information collected, the SWWS identifies patterns in wildlife population migration and hotspots of activity.
- Artificial Intelligence / Machine Learning
- Behavioral Technology
- Big Data
- Imaging and Sensor Technology
- Internet of Things
- Software and Mobile Applications
- Other
- United States
As the smart wildlife warning system is a prototype, it is expected to serve a population of 10,000 as estimated by it’s deployment in a small town in Oregon. It aims to directly provide safety and warning mechanisms to protect those it is deployed for. Furthermore, it's protection for wildlife populations from roadkill is expected to be for around 40 large mammal species. With the prototype matured and established as an effective solution among, it hopes to service entire cities of size around 100,000 people. At this stage, the SWWS aims to protect entire ecosystems of wildlife populations estimated around 5,000 terrestrial small to large-sized wildlife species. Past it’s deployment in cities and significant rugged terrain, the final goal is to create a community of warning signs to create a warning network that will service entire states and regions through the power of the Internet of Things. It's also the warning system's hope to be able to protect all wildlife within it's deployment region from road-related collisions.
In the next year, the project hopes to receive the government approval or contract allowing deployment of the most recent prototype which will then be matured via continuous testing as an established solution in small towns. With success as a traffic solution and ecological sentinel, the project then hopes to receive a grant large enough to recruit a small team and deploy 100 units across the roads in the state of Oregon for a small test run for analysis by June of 2022. Ultimately by the end of a year-long engineering period, the SWWS project hopes to have achieved preliminary success with seeing through it's primary features of reliable wildlife detections/warning, accurate animal classification, and insightful animal activity data analysis via hotspot maps/migration patterns in real world situations safely.
Following the sustainable development goals 9 and 15, the SWWS project has gauged it's progress by the impact it hopes to make once it has passed it's current prototype stage in using innovation to protect livelihood of terrestrial wildlife. One such impact is the amount of verifiable collisions it can prevent when deployed in real-world road situations. The SWWS hopes to surpass 500 correct and reliable true-positive detections via driver witness. This demonstrates it's ecological sustainability in preventing wildlife collisions along roads. It also underlines the SWWS' ability to protect wildlife populations from road-related deaths. In addition, measuring the amount of wildlife it has detected and collected animal image data over time, it hopes to be able to recognize and track over 40 large mammal species native to the Pacific Northwest. This monitoring capability intends to further understand ecological population health and better optimize existing road infrastructure to providing safety for migrating wildlife.
First, the SWWS needs to be tested in the real world. The Smart Wildlife Warning Sign has never been tested on public roads due to traffic laws and government regulations regarding the logistical and environmental impact of the warning sign, privacy of images collected from the field to test, and consistency of long distance data transfer with low wireless signal strength or no wireless network at all in field.
Second, the wild animal image database trained on was smaller than desired. Images had a limited variation of lighting, animal species, and positioning. Thus, testing my computer vision and machine learning algorithms with real photos collected in the field would be extremely beneficial.
Furthermore, the Smart Wildlife Warning Sign contains wiring and electronic components that need to work reliably in the harsh wild environment. Current field-testing deployment has consistently prevented weathering from the elements, but battery and deployment lifetime is still of concern for extreme durations of time. The SWWS would like to lower the power consumption of data transmission and image recognition in order to make it energy efficiency and comply with the FCC radio regulations.
As a team consisting of one engineer and supported by numerous mentors, the manpower onboard is quite limited. However, extensive coding experience and hardware familiarity over the course of continued development during a 5 year period has resulted in strong capabilities to design components in CAD software, manufacture materials, engineer features using both hardware systems and software system code, incorporate IoT smart sensor/communication, and feature deep-learning based AI models for wildlife image and migration pattern detection. It's been an absolute pleasure to work on this project as tinkering and wildlife conservation has always been my passion. Supported by some of the most experienced, passionate and resoundingly helpful team of mentors in all the work described above, I am confident in continuing to prototype and engineer this sustainable solution for a better future. Furthermore, since the conception of this project, consistent discussion and dialogue with potential users/researchers that would benefit from utilizing this tool in the future has helped shape the project's direction and accessibility for maximized impact among drivers, wildlife, and ecologists alike.
Currently, the SWWS project has been guided by the WildMe Conservation group, mentored at the UC Davis Road Ecology lab, conducting testing at the Tualatin River National Wildlife Reserve, and advised by the ODOT and ODFW. Collaborating with WildMe has entailed guidance on computer vision and wildlife detection systems that they've worked extensively on. At UC Davis, the project is mentored by Director Shilling and focuses on maturing solution features as well as close supervision over research conducted at the wildlife reserve. Working with park rangers and ecologists on-site at the wildlife reserve, SWWS prototypes are deployed, performance tested, and improved on based off results collected when conducting in-field research. ODOT and ODFW engineers and ecologists respectively have been advising this project in guidance as potential users/customers of the SWWS service.
- No
N/A, not available for this project.
- No
N/A, not available for this project.
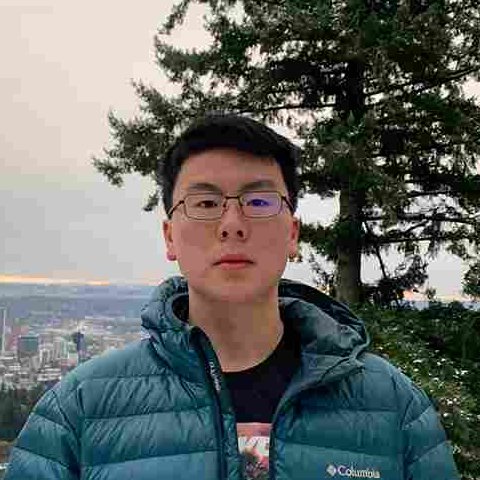