What If…? Pandemic Policy Simulator
Epidemics require specific mitigation strategies to avoid preventable mortality and saturation of the healthcare system. With emerging infections such as COVID-19, mitigation strategies are often untested and their efficacy may fluctuate over time and vary across geographic and socioeconomic contexts, making it particularly challenging to manage.
The What if…? platform aims to assist policymakers to tailor mitigation strategies according to their socioeconomic, geopolitical, and cultural setting and provide live data-driven insights from a massive and diverse global datastream in an intuitive web interface. Finally, as policies depend on public compliance for efficacy, What if…? also seeks to communicate the costs/benefits of interventions to promote compliance through evidence.
If scaled globally, the platform will be able to assist policymakers in enacting efficient responses to future pandemics as well as improve public compliance by adding transparency to the expected effects of the enacted policies.
Epidemics are, by definition, a sudden, unanticipated surge of
infectious spread that requires specific mitigation strategies to avoid
preventable mortality and saturation of the healthcare system. With
never-seen-before infections such as COVID-19, mitigation strategies are often untested and their efficacy may fluctuate over time and vary across
geographic and socioeconomic contexts, making them particularly
challenging to manage. For instance, despite a full year of intensive
research on COVID-19, governments still struggle to find the optimal set
of policies to respond to the continuously evolving epidemic.
Moreover, a large number of enacted policies are subject to non-compliance by the public due to a lack of a transparent communication tool between the policy makers and the public. Many a times, this lack of trust stems from the inability of the public to access the rationale behind these policies.
While this solution specifically seeks to find the optimal RESPONSE to health emergencies, it does so by DETECTING them and then computing a data-driven response personalised to its socioeconomic, demographic and geographic setting. The platform is additionally designed to create pandemic simulations and recommend tailored responses that can guide PREPARATION strategies for future health threats.
THE WHAT IF…? PLATFORM
We propose What if…?: an interactive pandemic policy simulator, available on an intuitive, open-source web platform that leverages a massive live global data stream to predict the progression of a pandemic as well as its economic and health consequences. It aims, to:
PREPARATION: By allowing policymakers to simulate health emergencies and visualise the estimated costs of the recommended interventions. They can identify and quantify their needs and undertake data-driven preparation strategies.
DETECTION: By screening a massive live stream of data, the system can also be used for early detection of anomalies and the required actions for mitigation.
RESPONSE: The greatest strength lies in its ability to guide policymakers in tailoring mitigation strategies according to their specific geographic, socioeconomic and cultural contexts. What if...? predicts the efficacy of various policies and computes the optimal combination of strategies to suit a specific context.
EFFICACY & PUBLIC AWARENESS: By providing an intuitive platform for visualising all the above, our platform also works to improve transparency in public health decision making by allowing the public to appreciate the data supporting the efficacy of the intervention in various contexts and thus encourage evidence-based compliance.
In short, the platform hosts machine learning driven models (specifically reinforcement learning) that predict the healthcare and economic consequences of the epidemic in order to determine a set of policies that optimises a trade-off between lives and livelihoods.
Our approach serves several communities with tangible impact
Policymakers: Our interactive and intuitive platform and automatically updating model allows policymakers to make data-driven decisions based on the latest information, personalised to the socioeconomic, geopolitical and cultural features of their specific context.
General public impacted by mitigation strategies: This same platform can be accessed by the public to encourage transparency in the decision making process and evidence-based compliance.
Data scientists in resource-limited settings: This solution funds 5 internships for aspiring data scientists from resource-limited settings via our well established machine learning exchange program: https://www.epfl.ch/labs/mlo/m... Each of these students will be supported for a distance-learning diploma in machine learning from the applicant’s institution (EPFL).
- Strengthen disease surveillance, early warning predictive systems, and other data systems to detect, slow, or halt future disease outbreaks.
The What if…? platform is specifically designed to perform the exact goals of the challenge i.e. PREPARE, DETECT, and RESPOND to pandemics.
It goes further to engage the public and reduce MISINFORMATION to better ensure efficacy by promoting evidence-based COMPLIANCE.
PREPARE: Simulates future health emergencies and visualises the estimated costs of recommended interventions.
DETECT: By screening a massive live stream of data and predicting future events.
(personalised)RESPONSE: Predicting the efficacy of policies and computing the optimal combination of strategies to suit a specific geographic/socioeconomic/cultural context.
MISINFORMATION: The intuitive public platform will also improve transparency in public health decision making.
- Pilot: An organization deploying a tested product, service, or business model in at least one community.
Currently, the model is fully functional and predicts the country-specific R-value and policy effectiveness for COVID-19 in over 150 countries with excellent accuracy.
It continues to learn from evolving pandemic data and is fully integrated into the SwissRe/Palantir Risk Resilience Center platform to leverage their massive and diverse global datastream. This latter research framework is already used by several large-scale companies and universities.
Thus, the What if...? model is ready for growth: testing its efficacy on new communities (i.e. the public and policy makers) as well as extending it to new countries and data streams.
Specifically we seek to expand the model to economic indices and to add reinforcement learning to predict the optimal policy set for health emergencies beyond COVID.
Our machine learning exchange program to promote data scientists in resource-limited settings (integrated into this application) is ready for a GROWTH phase with 5 further interns from sub-saharan Africa (https://www.epfl.ch/labs/mlo/mlx-machine-learning-academic-exchange-programme/).
- A new application of an existing technology
The What if...? pandemic policy simulator is innovative not only in its methodological approach and use of the uniquely diverse and massive-scale DATA STREAM but also in its high predictive PERFORMANCE that provides PERSONALISED insights to various socioeconomic, geographic and demographic contexts.
Particular novelty is also found in its wide GEOGRAPHIC SCOPE (>150 countries) and the broad target audience (with a specific interest in acting as a communication platform between the public and policymakers, to promote transparency, reduce misinformation and encourage evidence-based compliance to recommended policies).
Of these, we specifically highlight the following:
1) Methodology: What if..? uses time-tested machine learning approaches to extract personalised insights on a global scale. It is also unique in its approach on being able to analyse past policy decisions, plan for current decisions from a realtime data stream and even predict into futrue hypothetical scenarios through simulation.
2) Data stream: The massive and uniquely diverse data stream is secured via an established collaborative effort with SwissRe/Palantir which is constantly updated and expanding, allowing our tool to grow similarly in scope.
3) Academic sustainability model: By integrating this effort into an academia framework, we not only offer open access to ensure wide audience, but also benefit from peer review, which works to foster trust, reduce misinformation and increase the quality and performance of our models as well as encouraging innovations. Finally, it also benefits from future financial sustainability through academic grants.
- Artificial Intelligence / Machine Learning
- Big Data
- Software and Mobile Applications
- Rural
- Peri-Urban
- Urban
- Low-Income
- Middle-Income
- 3. Good Health and Well-being
- Switzerland
- Rwanda
- South Africa
- Switzerland
- Tanzania
Current: Pilot study (group of <20 researchers)
1 year: Growth phase (broader research community in machine learning and global health--> via publication in peer reviewed scientific journals with open access code.)
5 years: Scale (global, platform accessible to the general public, policymakers and researchers)
Our goals are inherently measurable and time bound:
In the first phase, the model will be expanded to predict economic consequences of the pandemic. In the second phase, the platform will include reinforcement learning to predict the optimal policy set which will be evaluated retrospectively for coherence. (i.e. Model performance metrics such as mean absolute error, sensitivity, specificity and 95% confidence intervals)
A later phase will test the platform on other re-emerging diseases such as malaria, turberculosis and dengue (i.e. number of diseases evaluated)
The machine learning exchange program will produce several data scientists in resource limited settings with diplomas from EPFL (one of the top-10 universities in the world) https://www.epfl.ch/labs/mlo/mlx-machine-learning-academic-exchange-programme/ (i.e. number of qualified data scientists trained in low-resource settings)
The open source peer review approach will also mean that we track the number of publications as well as the number of pushes and suggestions to the open access github repository.
- Nonprofit
Full-time MSc thesis students (4)
- no cost
Part-time postdoctoral scientist and MD (1)
- no cost (funded by alternative sources)
Full-time PhD students (2)
- USD60'000/year = USD120'000
Full time interns (5)
- USD5'000/year diploma course with the machine learning exchange program = USD25'000
- USD20'000/year salary = USD100'000
TOTAL = USD250'000 (incl. overhead)
Our core research team is deeply interdisciplinary and are all based at Intelligent Global Health, EPFL, Switzerland.
We comprise several data scientists with special expertise in various data types and models (socialepidemiology, big data, reinforcement learning etc), multiple full stack developers, a medical doctor, global health researchers, and an epidemiology/infectious disease specialist.
We further team up with the established data science and web development teams of Palantir and SwissRE to access large scale datastream and intuitive user interface.
Over the past 15 months we have built our prototype with the following diverse team:
- Mary-Anne Hartley (MD, PhD, MPH), Head of intelligent Global Health, EPFL (Clinician in rural health, Epidemiologist, Emerging infectious disease)
- Martin Jaggi (Prof), Head of Machine Learning and Optimization Laboratory, EPFL
- Prakhar Gupta, (PhD) Data Scientist, Mathematician
- Tatjana Chavdarova (PhD), Data Scientist, Mathematician
- Francesco Posa (MSc), Data Scientist, Full stack developer
- Giorgio Mannarini (MSc), Data Scientist, Full stack developer
- Thierry Bossy (MSc) Data Scientist
- Lucas Massemin (MSc), Data Scientist
- Pablo Cañas (MSc), Data Scientist
- Franziska Lippoldt (MSc), Machine Learning Engineer
- Andrea Pinto (BSc), Data Scientist, Full stack developer
With this expertise and experience at one of the top 8 universities in the world (EPFL), we feel that we are uniquely capable of delivering this ambitious and extremely feasible project.
Our group firmly believes in equal opportunities in research and has already established a program to employ promising students from low resource settings called the machine learning academic exchange program: https://www.epfl.ch/labs/mlo/m...
We aim to use MIT solve as an opportunity to scale this program to establish its utility and thus promote it in other research labs.
The program provides world-class training in data science for students in Sub Saharan Africa (distance learning to better secure local expertise) and then offers internships at EPFL as part of their sponsored studies.
We aim to engage 5 such students in the program via MIT solve.
Otherwise, the iGH research group is naturally diverse and headed by a female of African citizenship. Due to the nature of the research, the students applying are more or less gender neutral and resource limited settings are heavily represented.
The gender and race balance in the team is monitored and when imbalances occur, efforts will be implemented to correct them in a strictly merit-based approach.
- Government (B2G)
The MIT solve challenge is so uniquely suited to our target that we feel it would be a perfect fit as the main and only initial sponsor of our work.
As this specific project relies on peer-review to foster public trust and transparency, we feel that being selected by MIT Solve would be invaluable by vetting via their independent expert judging panel.
The adjudicating process at MIT solve is also more transparent and diverse than at academic journals, so we feel strongly that peer review should not only include experts but also the public. Thus MIT solve is the perfect choice for our inaugural funding.
We are also specifically applying for access to essential funding. While we can get always ensure continuity and sustainability to maintain the platform with our endless supply of master thesis students (free), the option is too slow. We need seed funding to kick-start the effort with dedicated researchers.
It would accelerate activities to ensure delivering the platform in time for policymakers to use it to respond to the ongoing epidemic, or to perform "post-mortem" analysis on their interventions or better plan for the next waves or new diseases.
- Human Capital (e.g. sourcing talent, board development, etc.)
- Legal or Regulatory Matters
- Public Relations (e.g. branding/marketing strategy, social and global media)
- Monitoring & Evaluation (e.g. collecting/using data, measuring impact)
- Product / Service Distribution (e.g. expanding client base)
1) Human capital As one of the top 10 universities in the world, we have an excellent pool of experts from which to draw talented human capital. However, we require funding to do so. This is an important motivation for our application.
2) Public relations (and Monitoring) The peer review process and vetting procedure of MIT solve is an important component of our monitoring and evaluation strategy. We would be very grateful for frequent independent peer review which we will also seek when publishing our work in peer-reviewed conferences and journals.
3) Service Distribution We also see MIT Solve as an excellent opportunity on which to develop our user base and better distribute the service to policy makers and their already established network of stakeholders.
We would like to propose to partner with the Patrick J. McGovern Foundation and SOLVE member Vilas Dhar, for the following reasons:
1) Getting support in advancing the project as a non profit, especially for support at finding/collecting/collaborating with data as a non profit entity
2) Getting support in education in data science based upon the foundations project/partnership with the hidden genius project
In particular, we would like to discuss participation in the data and society program for further acceleration.
- No, I do not wish to be considered for this prize, even if the prize funder is specifically interested in my solution
- No, I do not wish to be considered for this prize, even if the prize funder is specifically interested in my solution
- No, I do not wish to be considered for this prize, even if the prize funder is specifically interested in my solution
- Yes, I wish to apply for this prize
Our work is designed to be a public good and serve the public through data-driven insights on health emergencies.
It not only informs them using objective analyses but also provides evidence for the efficacy of public health measures. It guides policymakers on approaches to to minimise both economic and health impacts of interventions and reveals inconsistencies in collected data.
This is a project that is benchmarked on the COVID pandemic, but that can be extended to other diseases and hypothetical scenarios that help countries prepare, respond and assess their responsiveness to public health emergencies.
- Yes
Our work is designed to be a public good and serve the public through data-driven insights on health emergencies.
It not only informs them using objective analyses but also provides evidence for the efficacy of public health measures. It guides policymakers on approaches to to minimise both economic and health impacts of interventions and reveals inconsistencies in collected data.
This is a project that is benchmarked on the COVID pandemic, but that can be extended to other diseases and hypothetical scenarios that help countries prepare, respond and assess their responsiveness to public health emergencies.
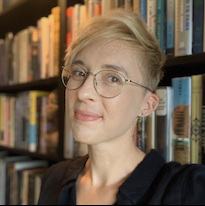
Group lead
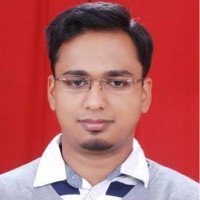
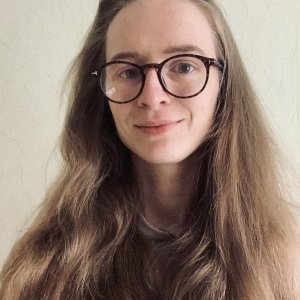