AI-led Infectious Disease Early Warning
Poor surveillance and delays in response to suspected ID cases leads to the spread of major outbreaks, highlighting the important role that primary care plays as the first port of contact.
Our solution makes use of the Big Data in Patient’s eHR (electronic health record) in primary care and the application of machine learning (ML) to develop a system that can identify and monitor emerging pattern of symptoms and presentations of common and novel IDs in REAL TIME; hence, enable early identification of community outbreaks. To ensure our solution is ethically sound, we have conducted a systematic review and a large qualitative study to frame the inherent ethical issues, critical to medical surveillance systems using Big data and ML.
The value of early detection of ID outbreaks within a primary care setting, measured in hours or days, can be of immense significance in predicting, controlling ID spread and reducing mortality.
High population density in urban China, combined with cultural practices of rural living in close proximity and consuming (wild) animals, is likely to have led to serious, frequent ID pandemics such as SARS and COVID-19. As of 29 April 2021, WHO has reported >149million confirmed COVID-19 cases with >3.1million deaths.
Over the past decade, China has made remarkable progress in its primary healthcare with >34,000 community health clinics (CHCs) and 300,000 general practitioners (GPs) serving over 13billion people. In 2017, the first team of national AI champions was developed for healthcare and service delivery, leading to the development of an intelligent robot by iFlyTek (Chinese IT company), that passed China’s written national qualification exam for doctors, hailed as a game changer for the country’s strained healthcare system.
Importantly there are considerable complex ethical and legal concerns on accessing, using and sharing of mass patient eHR including the potential misuse of personal information, on social media for example, and informed consent that infringes on citizens’ human and civil rights. The application of ethical regulations or rules on privacy, confidentiality, transparency and security for ML transmission required are sparse.
Our solution is to extract eHR data from the IT system of the Department of Family Medicine, at the University of Hong Kong-Shenzhen Hospital (HKU-SZH), China from Jan 18-Dec 20 for model construction and testing in the community.
A simplified illustrate example:
1. Patient-X goes to a CHC for “fever”.
2. During consultation, the clinical history, symptoms and demographics will be entered into the eHR in real time.
3. Anonymous eHR data will be uploaded to cloud after gaining informed consent. Diagnosis models will calculate the possibilities of this case for any known ID. If the possibility is higher than the pre-set threshold, the system will return the risk of specific ID to the team and subsequently to the GPs for further investigations.
4. Uploaded data will also be applied to a warning model that factors in historical temporal trend, multiple known/suspicious ID cases and abnormal pattern or clustering of symptoms reported over certain short periods. If the risk produced by the warning model is higher than the pre-set risk threshold, the system will generate a warning message along with the summary of all suspected cases, which can be passed to the CDC for further investigation.
Our solution serves to protect community and national health against the spread of common and/or novel IDs through developing an early warning system. This will improve response time and strategies for ID control. We intend to develop early warning models for 16 different IDs (8 infectious causes out of the 30 most commonly presenting complaints in primary care, such as influenza, gastroenteritis, and 8 commonest notifiable IDs in China, e.g. hepatitis B virus (HBV), tuberculosis etc.).
Depending on the ID in question, potential predictors include, but are not limited to: clinical signs and symptoms related to western medicine and TCM (which may yield unknown risk factors and new inter-relationships of the symptoms and IDs), clinical observations (e.g. heart rate, respiratory rate, blood pressure, oxygen saturation, temperature, BMI), past medical history and co-morbidities, vaccination history, social history (occupation, smoking, alcohol, substance abuse history), drug history and allergies, sexual history, recent travel history, demographics (e.g. age, sex, residential address, family members). Recent eHR on COVID-19 patients at HKU-SZH (first receiving hospital during the pandemic) will also be used in model calibration.
Two levels of data will be extracted from eHR of HKU-SZH. One is direct input structured data such as age, sex, temperature, weight, height, blood pressure, diagnosis as international classification of diseases (ICD-10)/ international classification of primary care (ICPC). The other level is the doctors’ eHR that include text related to common symptoms and signs, such as headache, chills, nausea and vomiting, loss of appetite, diarrhea etc. These will be transferred into structured data using natural language processing techniques. Data extraction and pre-processing, model development and calibration of the ID warning system will involve several well recognized ML-based computation techniques (more details below).
This system will operate at the primary care level (CHCs), and serve end users general public healthcare professionals, Centers for Disease Control and Prevention (CDC) and the government. This system will enable the CDC to act swift in response to an outbreak e.g. contract tracing, quarantine and social distancing measures, border control etc., in order stop the transmission chain and prevent further loss of life, adverse social and economic impacts
The early predictive alerts to authorities any potential ID outbreaks enable them to take proportional responses for containment e.g. healthcare resource allocation, which serve to prevent escalation into a pandemic scenario. Furthermore, trends, predictions the pattern of the ID spread, and associations, especially relating to human factors, in turn, improving our understanding of ID, unknown risk factors and inform our ID control policy.
The construction of our system will be underscored by an ethical framework including legality of data collection and use, data privacy and security, robustness and interpretability of results, and authorization and responsibility in model application. Right at the start, we undertook a systematic literature review on 29 peer-reviewed articles and secondly, a national qualitative study with frontline GPs and patients is being conducted in China to identify the l ethical implications of big data analytics. We intend all steps in early warning system to be underscored by an ethical decision-making framework.
- Strengthen disease surveillance, early warning predictive systems, and other data systems to detect, slow, or halt future disease outbreaks.
Our solution can detect unusual ID patterns against seasonal changes e.g. in flu, giving days advance notice to plan healthcare resource. It can detect increases in STI-like symptoms or treatment on Syndromic Approach alerting local CDC to investigate and initiate contract tracing/ mandatory testing for at-risk groups. Early identification of HBV outbreaks can alert GPs to test for HBV in at-risk groups and linkage-to-care. It can also reveal trends, predict the pattern of ID spread and associations, especially on human behavior and interactions, which would improve our understanding of ID, identify unknown risk factors and would guide ID control policy.
- Prototype: A venture or organization building and testing its product, service, or business model.
We gained IRB and completed the data transfer and began model development based on 300,000 patients’ eHR from the department of Family Medicine at the HKU-SZH in the period Jan 2018-Dec 2020.
Our system will be assessed in terms of:
- Effectiveness: We target our case detecting models to have AUROC of 70-80% and AUPRC > 0.5, higher than those of clinicians. Using historical Covid-19 eHR as validation data, we target that our early warning model will successfully detect COVID-19 within two days from the first positive case with false alarm rate <50%.
- Timeliness: Target response time of suspected case detection models to be < 2sec, in order to facilitate busy clinical practice, and that of early warning system to be < 1hour.
- Privacy and security: Data security protection strategy will be built ensuring possibility of re-identification is close to zero at all stages of data lifecycle.
- A new application of an existing technology
The innovation and uniqueness of in our solution lies not only in the creation of an ID early warning system at primary care level using Big Data and ML technology, which not only has a global benefit in ID control and prevention, but also by providing a novel rigorous ethical governance framework to ensure the integrity and transparency in the day-to-day application of our ID early warning systems.
Accordingly, we shall setup an independent Ethics Steering Committee to steer the development of and guidelines for all steps of this project. Furthermore, we will set up a public website to promote social awareness explaining the purpose, risks and benefits of the AI-led early warning system, disclosing the model algorithms, other relevant information concerning the data security to maximize transparency.
· To ensure our models are robust and interpretable, we will visualize the models and unfold the “black box” using Shapley values. Clinical experts will be invited to assess our models.
· To ensure the legality of data usage, we will obtain informed consent before using the models.
· To guarantee the data privacy and security, we will conduct data anonymization, encrypted data transmission, data masking, and follow Principle of Least Privilege, use cloud to store the data and will build a disaster backup and recovery plan.
- Artificial Intelligence / Machine Learning
- Big Data
- Software and Mobile Applications
- Rural
- Peri-Urban
- Urban
- 3. Good Health and Well-being
- China
- China
Currently our solution is at concept stage.
In the first year, we plan to develop and our prototype system in the Department of Family Medicine at the HKU-SZH, China. The Department was established in 2011 currently draws 100,000 patient visits per year from the municipality of Shenzhen, Guangdong and nearby provinces.
Upon successful completion of the testing phase, we plan to integrate the early warning system into the IT systems of all CHCs (>4000 CHCs) in Shenzhen City which has a population of 12.5 million (timeline: 3 years)
By 2026 we intend to integrate our system into consented CHCs (total 15,000) in China which currently serve a population of 1.3 billion people.
The goal of our solution (AI-led early warning system) is to prevent and control the spread of common and novel IDs in the community.
During the model training stage, we will build an iterative system to update the structures and the parameters of this early warning model by the update of eHR information, to make sure it could be automatically upgraded and optimized as data grows. Our initial goal is to build a prototype early warning model for 16 different IDs with 80-90% prediction accuracy within 95% CI.
Here are some measurable indicators we will use to measure our progress:
- During the pilot phase - the number of common (and novel) ID outbreaks identified in the first year of implementation of early warning system at Department of GP at HKU-SZH and one selected CHC;
- Post pilot phase: the number of CHCs adopted our ID early warning system in CHCs in China and elsewhere
- the incidence of common (and novel) IDs
- the mortality rate of common (and novel) IDs
- Estimated number of cases prevented and mortality rate prevented
- the time lag (measure in days and hours) of early warning and the prediction of a flu outbreak
- Number of complaints or ethical-related issues addressed this project;
- Number of other guidelines/ systems that would adopt our ethical approach.
Furthermore, we will adopt UNPD framework to measure the social and healthcare impact of our work.
- Nonprofit
Jia LIU (JL), Weinan DONG (WD), Qin PANG (QP), Xiao Qin LU (XQL), Eleanor HOLROYD (EH), Alex MOLASIOTIS (AM), Ivy Yan ZHAO (IYZ), Yexuan MA (YM)
WCW will be the team leader. He is the Chief of Service at the Department of GP’s at HKU-SZH and an expert in primary care and infectious diseases. He will be ultimately responsible for liaising with various stakeholders on implementing the project, data collection, data analysis and report writing.
JU (Deputy Director of Laboratory of Engineering and Scientific Computing at SIAT) and WD will take on the lead role in model building and testing; modify and add more IDs into the models and advise on its incorporation into practice. PQ co-ordinates the transfer of eHR data from HKU-SZH for SIAT and adaption of the model into existing consultation system.
XQL coordinated the qualitative study and advise on its adoption and implementation into the community.
EH (Professor of Nursing, the Research Head of School of Clinical Sciences, Co-director Centre for Migrant and Refugee Health) and AM (Director of WHO Collaborating Centre for Community Health Services) supervises the systematic review, oversee the qualitative project, analysis and publications, and provide further steerage guidance for developing the ethical framework.
IYZ and YM will help coordinate the project, liaise with study team, stakeholders and participants, literature review, data collection, data analysis and report writing.
Diversity
Our leadership team is comprised of a culturally diverse pool of academic individuals with different expertise and experiences, in the field of Primary Care, Nursing, IDs, Medical ethics and Computer sciences from different academic institutions in Hong Kong, mainland China and New Zealand. Also our steering committee will consists of a diverse pool of representatives from legal, medical, healthcare, human right communities as well as government bodies and general public.
Equity
Our team is also made up of frontline health professionals, academics to PhD students and post-doc fellows. We have regular meetings when open, robust recorded discussions. Since this project is done without major funding, the team members join because they see its value and are committed to improving health care outcomes d support provided. Our solution will serve at the Primary Care and benefits everyone in the community, in particular the vulnerable groups, and therefore embodies the values of social health equity.
Inclusion To ensure our early warning system serves its purpose and operate in an ethical manner, we conducted a national qualitative study with 16 frontline general practitioners (GPs) and 32 patients in China to identify potential ethical implications of big data analytics using patient’s eHR. We respected everyone’s opinions on this topic and strive to addresses their concerns when building and applying the ML models, with the setup of an ethic steering committee to oversee the use of eHR and establish guidelines on data usage for this project.
- Organizations (B2B)
The HKU-SZH has been the forerunner in raising standard in China healthcare reform. In this project, we pride ourselves in setting up Primary Care ID early warning system which serves its purpose in an ethical manner. Gaining recognition in the Solve Challenge will serve to reaffirm the international benchmark and our commitment to upholding a high ethical standard in the use Big Data Analytics in healthcare. This could help the team to provide further evidence that such approach is needed and convince our partners to implement it in a wider context. We also hope to get reviewers’ comments to further improve our work.
- Business model (e.g. product-market fit, strategy & development)
- Financial (e.g. improving accounting practices, pitching to investors)
Our team made up of IT, ethics and primary care clinical academics but we are short of business/ financial experts who can help to build the model and disseminate to the communities for maximum impacts.
We are open to suggestions for the expertise needed above.
- No, I do not wish to be considered for this prize, even if the prize funder is specifically interested in my solution
- No, I do not wish to be considered for this prize, even if the prize funder is specifically interested in my solution
- No, I do not wish to be considered for this prize, even if the prize funder is specifically interested in my solution
- Yes, I wish to apply for this prize
Poor surveillance and delays in response to suspected ID cases leads to the spread of major outbreaks, highlighting the important role that primary care plays as the first port of contact.
Our solution makes use of the Big Data in Patient’s eHR (electronic health record) in primary care and the application of machine learning (ML) to develop a system that can identify and monitor emerging pattern of symptoms and presentations of common and novel IDs in REAL TIME; hence, enable early identification of community outbreaks. To ensure our solution is ethically sound, we have conducted a systematic review and a large qualitative study to frame the inherent ethical issues, critical to medical surveillance systems using Big data and ML.
The value of early detection of ID outbreaks within a primary care setting, measured in hours or days, can be of immense significance in predicting, controlling ID spread and reducing mortality.
- No
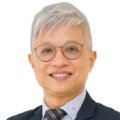
Doctor