Machine learning the lessons of Covid-19 to predict virus spillover
In which animals do viruses hide? This is the question we must answer to prevent future pandemics. Integrating machine-learning and complex-networks, we will predict viral hosts. We will verify predictions, and enhance predictive capabilities, using results from ongoing field sampling and our own in vitro experiments.
Dr Marcus Blagrove
- Identify (Determine & limit the disease risk pool & spill over risk), such as: Genomic data to predict emerging risk, Early warning through ecological, behavioural & other data, Intervention/Incentives to reduce risk for emergency & spill over
Two-thirds of emerging human diseases are zoonotic; ie, caused by pathogens which spillover from animal into human populations. Many viruses of public health concern are zoonotic – eg, HIV, avian and swine influenzas, Zika and Ebola, and three coronaviruses (SARS, MERS and SARS-CoV-2).
There are signs that zoonotic viruses are emerging more frequently, with viruses of public health concern now arising every 2-3 years. Left unchecked, this trend may continue. However, the global health, social and economic costs from just SARS-CoV-2 make an incontrovertible case for investment to prevent future spillover events that could trigger a pandemic. The challenge is to know where to look: where are future pandemic viruses hiding?
Armed with this knowledge, we can implement measures to minimise spillover risk; heighten surveillance for spillover events; and even lay the groundwork for diagnostics, therapeutics and vaccines, should an outbreak commence.
There are, however, too many animal species to get this knowledge with untargeted sampling. Targetted sampling is needed to maximise chances of success. Our problem is therefore as follows: how can we identify those animal species which are most likely to harbour viruses of public health concern, and where is spillover into human populations most likely to occur?
There are two main direct recipients of our solution:
1) Policy makers and governmental agencies.
We hold meetings every three months with government agencies (described later), in these meetings we discuss the outcomes of our findings, specific high-risk examples and our recommendations. Agencies reciprocate with their specific targets. These meetings help ensure our work is of most use to the direct recipients and people implementing mitigations as a result of our work.
2) Scientific beneficiaries.
We will produce robust frameworks, trained models and large volumes of data, which will enhance the quality of research undertaken globally. We will make our data and models open-access, and our codes open-source, and we will release them to accompany publications. Archiving in repositories will enable further use by any interested party or to advise on any ongoing policy/strategy. We will host virtual meetings with scientists working in similar areas, and organise seminars and hackathons to address specific mini-challenges, and exchange codes and data.
The indirect recipients are the global populations of both humans and animals. Policy changes and surveillance aimed at decreasing virus sharing and spillover will benefit everyone.
- Pilot: A project, initiative, venture, or organisation deploying its research, product, service, or business/policy model in at least one context or community
- Artificial Intelligence / Machine Learning
- Big Data
- GIS and Geospatial Technology
Our project will produce two major ‘gold open access’ peer-reviewed publications: 1) the Initial host/virus associations and spillover predictions at the end of year 1, incorporating our expanded framework. 2) The final predictions at year three, informed and enhanced by experimental testing. We also expect it to contribute to other publications in our groups (e.g. from the data curation and model development).
All of our code, once published in a journal, is made fully publicly available as standard (see our previous publications).
The predictions of host/virus associations, and geographic hotspots, will be publicly available. Our intention is for our work to be utilised as much as possible to inform and enable geographically targeted surveillance programmes, & to detect spillover and viral sharing as it is happening and before a major outbreak. Such information will help inform mitigation strategies as well as provide a vital early warning system for future viral outbreaks.
Our work will impact policy makers world-wide, who will be able to see improving predictions of animals at risk for specific viruses, and locations of sharing and spillover to humans. Armed with accurate predictions, they will be able to target surveillance and mitigation strategies against viral sharing and spillover.
As part of our onging work we regularly meet with government agencies including the UK Department for Environment, Food & Rural Affairs (DEFRA), Public Health England (PHE), and the World Organisation for Animal Health (OIE). These meetings enable us to disseminate our findings directly to government policy makers, ensuring rapid utilisation of our work.
We will produce a resource, in the form of an open access database of all interactions we predict, which will be continuously updated as our data are generated.
Finally we will have a considerable impact for animal health, both domestic and wild. Practitioners and policy makes will be able to see which viruses are predicted to infect animals they work with, and, geographically, where they are most at risk. Integral to ‘One Health’, improving animal health and minimising virus sharing risk will ultimately reduce the risk of spillover to humans.
Our prediction work is at a global scale – we predict virus/animal associations all over the world. In this project we will incorporate geographic, behavioural and habitat utilisation data in order to predict hotspots of viral sharing and spillover. These data and predictions will be global, utilising all available information for all animals and viruses in our work.
Consequently, our work will have the potential to impact policy and therefore the public world-wide. As our project progresses through the three years, our predictions will become more refined and accurate, enabling more precise strategies and impact.
We work closely with numerous governmental agencies, including Department for Environment, Food & Rural Affairs (DEFRA), Public Health England (PHE), and the World Organisation for Animal Health (OIE). As part of another project (Global trade of coronavirus hosts) we meet with government agencies every three months, we will report findings from this project in these meetings, to maximise exposure, and to enable policymakers to be directly and immediately advised of our findings, thus greatly speeding up any public health policy response.
External impact
For our publications we will use the altmetric service to determine the media/policy/etc. attention our work has received. For example, our coronavirus host prediction paper’s altmetric (https://nature.altmetric.com/details/100260216) puts it in the top 1% of outputs of a similar age, and top 5% of outputs of all time, including coverage by 61 major news outlets.
In addition, we meet regularly with key agencies including DEFRA, PHE and OIE (as mentioned above). we will be able to measure utilisation of findings by these agencies, and any further impact, in those meetings.
Performance assessment
During year one, we will assess the performance of our predictive framework using traditional hold-out tests against varied metrics, each capturing different assessment criteria (e.g., AUC, true skill statistics, and F-score).
Throughout the project newly field-observed host-virus associations, and their locations, will also be used to validate our predictions, prior to any integration into the framework.
Following our initial predictions (circa 12 months), we will begin laboratory testing our predictions. We believe this step is essential as it provides a level of validation not attainable through any other method (e.g. including negative associations).
- United Kingdom
- United Kingdom
Our only barrier is financial. Although we have so far been very successful in raising the funds required, further investment would allow for the staff hiring and expansion of the project as described in this proposal.
- Academic or Research Institution
All four team members are employed by the University of Liverpool, UK.
We are applying to the Trinity challenge for two main reasons
- Whilst we have been successful in raising funds for our solution so far, in order to expand our predicative capability beyond the proof-of-principle stage we require further financial backing to fund our work for the next three years.
- We also are continuously seeking to increase our impact and visibility of our solution. The breadth and influence of the judges and founding members, including the Global Virome Project, Bill & Melinda Gates foundation, universities, and health agencies will all directly and indirectly increase the visibility of our work to such audiences.
And winning a prestigious and competitive award also helps to increase our visibility and impact.
We already have the partners needed for our project to be a success, however, in order to increase our works visibility and impact further, partnering with Trinity Challenge member organisations such as the Global Virome Project would help in the dissemination and application of our findings to end users and policy makers around the world.
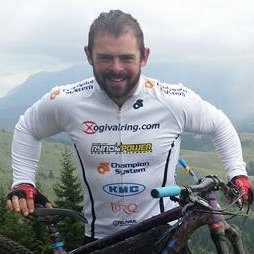
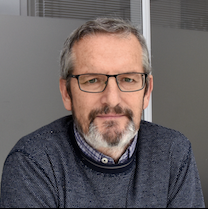
Professor
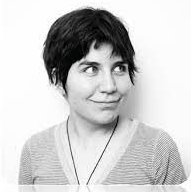
Dr