What If…? Pandemic Policy Simulator
What if …? A data-driven approach to quantify the efficacy of the type, duration, and stringency of epidemic mitigation strategies.
Dr Mary-Anne Hartley (MD/PhD/MPH) Senior scientist, Swiss Federal Institute of Technology (EPFL); Clinician/Epidemiologist in resource-limited-settings and emerging infections
- Respond (Decrease transmission & spread), such as: Optimal preventive interventions & uptake maximization, Cutting through “infodemic” & enabling better response, Data-driven learnings for increased efficacy of interventions
Epidemics are, by definition, a sudden, unanticipated surge of infectious spread that requires specific mitigation strategies to avoid preventable mortality and saturation of the healthcare system. With emerging infections such as COVID-19, mitigation strategies are often untested and their efficacy may fluctuate over time and vary across geographic and socioeconomic contexts, making it particularly challenging to manage. For instance, despite a full year of intensive research on COVID-19, governments still struggle to find the optimal set of policies to respond to the continuously evolving epidemic.
The What if…? platform aims to assist policymakers to tailor mitigation strategies according to their socioeconomic, geopolitical, and cultural setting and provide live data-driven insights from a massive and diverse global datastream in an intuitive web interface.
Indeed, mitigation policies aim to optimize a trade-off between lives and livelihoods according to ethical calculations that are not universally shared...and at a financial expense that may quickly saturate their cost-benefit ratio in low-resource settings, leaving lasting damage and secondary mortality. Thus, it is critical to design mitigation strategies according to their context-specific efficacy. Finally, as policies depend on public compliance for efficacy, What if…? also seeks to communicate the costs/benefits of interventions to promote compliance through evidence.
Our approach serves five distinct communities with tangible impact
Policymakers: Our interactive and intuitive platform and automatically updating model allows policymakers to make data-driven decisions based on the latest information, personalised to the socioeconomic, geopolitical and cultural features of their specific context.
General public impacted by mitigation strategies: This same platform can be accessed by the public to encourage transparency in the decision making process and evidence-based compliance.
Clinicians in resource-limited settings: the data capacity building component trains clinicians at rural hospitals in low-resource settings to perform point-of-care lung ultrasound and systematic data collection for improved disease surveillance.
Patients in resource-limited settings: The patients in the care of these clinicians will directly benefit from improved diagnostic capacity of point-of-care ultrasound and better antibiotic stewardship.
Data scientists in resource-limited settings: The data from the ultrasound and respiratory disease surveillance will be collected in the context of a clinical study with ethical approval (building on our existing and successfully implemented protocol (attached). The lung ultrasound images will then be analysed by local data scientists who will be sponsored for a distance-learning diploma in machine learning from the applicant’s institution (EPFL).
- Proof of Concept: A venture or organisation building and testing its prototype, research, product, service, or business/policy model, and has built preliminary evidence or data
- Artificial Intelligence / Machine Learning
- Big Data
- Software and Mobile Applications
Our work provides the public and policymakers with intuitive and interactive information about the effectiveness of pandemic policies. It allows them to find the optimal set of policies that balances the compromise between lives and livelihoods, and lets them analyse the various options for measures taken and assess the consequences of decisions made. It also functions as a communication tool to encourage transparency and interact with the public to explain decisions through data, cut through the infodemic and promote evidence-based policy compliance.
The capacity building component, which train rural clinicians in point-of-care ultrasound (a virtually consumable-free, easy to use, inexpensive, non-invasive and multipurpose diagnostic tool) seeks to improves access to diagnostics in resource limited communities and leave lasting knowledge and tools to improve disease surveillance and better participate in medical literature.
The What If…? Platform and its associated data capacity building project are specifically designed to have tangible impacts that can be intuitively visualized by our platform.
The What if…? model serves to guide policymakers in selecting the policies that are most effective in their setting thus minimizing secondary costs. The interactive visualisations in this tool, compute the consequences of various what-if…? scenarios, delivering tangible estimates in an intuitive interface to guide decision making and public understanding.
On the other hand, it also serves as a communication tool between policymakers and the general public allowing for better accountability and transparency allowing maximum compliance.
The data capacity building component in low-resource settings, directly improves patient care through enhanced access to diagnostics(e.g. lung and point-of-care ultrasound) while creating opportunities for clinicians to participate in clinical research. Thus, it builds rural research capacity, which also improves representation of marginalised communities and diseases in scientific literature whilst building careers in data science through our machine learning exchange program. The outcomes are certified data scientists trained via EPFL as well as scientific articles, improved diagnosis for emerging disease surveillance and antibiotic stewardship.
Our approach is inherently scalable, using the established and sustainable framework of research funding to build on the successes only possible through initial Trinity support.
Indeed the Trinity support would allow us to kick-start a large scale multi-site research effort in under-represented and data-impoverished communities at high-risk of emerging infections. Integrating this data capacity building effort with a global AI platform for emerging disease prediction (i.e.our What if...? platform) will consolidate and standardise efforts between the collaborating sites. By the end of the Trinity support, we will have clinicians and data scientists in several resource-limited rural settings, highly trained in systematic data collection and clinical research. We can then directly leverage this success to apply for further research funding to further expand the effort whilst further improving disease surveillance and patient care as well as promoting rural representation in clinical research.
Our goals are inherently measurable and time bound:
In the first phase, the model will be expanded to predict economic consequences of the pandemic. In the second phase, the platform will include reinforcement learning to predict the optimal policy set which will be evaluated retrospectively for coherence. (i.e. Model performance metrics such as mean absolute error, sensitivity, specificity and 95% confidence intervals)
A later phase will test the platform on other re-emerging diseases such as malaria, tuberculosis and dengue (i.e. number of diseases included)
The machine learning exchange program will produce several data scientists in resource limited settings with diplomas from EPFL (one of the top-10 universities in the world) https://www.epfl.ch/labs/mlo/mlx-machine-learning-academic-exchange-programme/ (i.e. number of qualified data scientists trained in low-resource settings, number of publications)
The rural research efforts will be designed to monitor and report diagnoses in intervention and control arms and thus establish the utility of disease surveillance with point of care tests such as lung ultrasound and biomarker type tests like CRP. (i.e. number of clinicians trained, number of unnecessary antibiotic prescriptions, probability of improving the diagnosis with these tools, number of respiratory diseases reported)
- Switzerland
- Benin
- Burkina Faso
- Cameroon
- Rwanda
- South Africa
- Switzerland
- Tanzania
ADEQUATE FUNDING: In order to maximise representation of data-empoverished settings in our data capacity building project, we require significant initial funding. While the effort is inherently scalable, a large kick-start would allow more sites to then become independently funded through further research grants awarded based on their success during the Trinity funding period. Thus we apply for the maximum amount of 2 million GBP to maximise the representation of limited-resource settings.
GEOGRAPHIC RESOLUTION: Currently, our model predicts outcomes at a country level, however many policies are undertaken on a sub-national level and indeed, policy efficacy can vary across subregions of a country. Thus we aim to include a more fine grained scale like state and city level legislations and also allow users to create their own hypothetical “countries” by inputting the socioeconomic, geopolitical etc features of their sub-region.
RANDOM EVENTS: Statistically unlikely, random events (e.g. super-spreader events) cannot be captured by our model (or any model). This cannot be fully mitigated, but can be explained to users and the effects on these events can be incorporated into future predictions.
- Academic or Research Institution
EPFL, Switzerland (Intelligent Global Health, Machine Learning and Optimization Laboratory)
Unisanté, Switzerland (Tropical Medicine and Vaccination Department)
Tshemba foundation and Wits Rural Health, South Africa
We feel that Trinity is one of the only funding bodies that appreciates the critical importance of improving representation in data-empoverished settings. This long term view is perfectly in line with our vision for building sustainable data collection and analytics in resource limited settings as a key approach to disease surveillance. Our What If…? Platform not only enables the prediction of personalised policies but also highlights areas where predictions are unreliable due to poor data collection.
Our interdisciplinary team has decades of experience in implementing large scale data collection and capacity building projects for public good and we thus sought a funding partner who shares this vision.
We believe that collaboration with the Bill and Melinda Gates Foundation would be highly complementary to your data capacity building goals. The PI of this application (Dr Hartley) has worked several times with BMGF for emerging disease surveillance, and we feel they would be extremely helpful in directing us to the latest disease surveillance approaches in the biobanks we aim to collect in rural hospitals.
For privileged data access, we would be interested to see if the “Institute for Health Metrics and Evaluation” could help provide finer-grained geographic resolution for the data feeding our models (i.e. sub-national).
The London School of Economics and Political Science is another potential partner to guide us with insights as well as raw-data concerning the economic consequences of the pandemic to develop our reinforcement learning objective function.
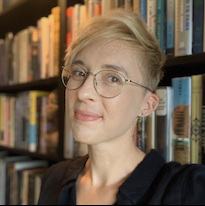
Group lead
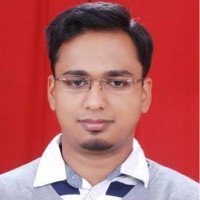