AI-model for detecting COVID-19 using CT Images
This strategy trains an AI model that uses CT images for rapid screening of covid-19 cases. To improve accuracy and speed of processing, instead of using volumetric CT Scan data, initially, boundaries of the volumes are identified, each boundarys' density, area, and geometric values are used for feature engineering.
Amir Akhavan Masoumi
- Respond (Decrease transmission & spread), such as: Optimal preventive interventions & uptake maximization, Cutting through “infodemic” & enabling better response, Data-driven learnings for increased efficacy of interventions
COVID-19 was discovered first in late 2019 in Wuhan, China, and since the discovery had infected more than five million people by mid-May 2020. COVID-19 became a pandemic in less than three months since its emergence. The characteristics of the virus and its ease of spread along with a wide range of social and economic outcomes have led to diverse strategies and policies in different parts of the world. The clinical studies conducted since the COVID-19 outbreak on the confirmed coronavirus patients have shown the presence of radiographic ground-glass opacification (GGO)signs. Therefore, CT (computed tomography) scan images can be used for diagnosis of COVID-19 when the coronavirus test kits are less accessible or the test results could be not reliable or take a longer time to get results and also when the patients present severe respiratory problems. However, large sizes and varieties of CT Scan images reduce the accuracy and speed of training a deep learning algorithm with the goal of detecting positive COVID-19 cases from CT scan images.
The final product can be used as an automated algorithm for the general MD practitioners, radiographers and pulmonologists, and other researchers in this field and can speed up the process of diagnosis of COVID-19 using CT images and other possible symptoms of the patients.
- Proof of Concept: A venture or organisation building and testing its prototype, research, product, service, or business/policy model, and has built preliminary evidence or data
- Artificial Intelligence / Machine Learning
- Big Data
- Crowd Sourced Service / Social Networks
- Software and Mobile Applications
By increasing efficiency and ease of access to a quick COVID-19 (and its variants) testing mechanism, it would be possible to detect possible cases and quarantine, or treat them in a shorter lead time, and reduce effective reproduction number (R), which in return reduces the number of the cases and helps in achieving a Zero COVID society.
In this study, the main goal is to use to propose a machine learning algorithm that uses CT images for rapid screening, model creation, and reducing/preventing the discrepancies and malpractices in the diagnosis of pulmonary embolism, lung cancer, and pneumonia have the potential to cause the loss of lives. A large database of labeled cases and a deep learning model trained based on it along with customized image processing algorithms to detect the prone areas in the CT images can assist rapidly screening the patients in cases where access to skilled pulmonologists and reliable test kits is difficult. It also can help pulmonologists with identification of the irregularities such as ground-glass opacities, lung consolidation, and cavities, nodules, pleural effusions, fibrosis in the respiratory system as well as reducing the risk of malpractice.
Having a quick and efficient screening mechanism can save the lives of people, and also the proposed mechanism can be used for the detection of other types of pulmonary complications, therefore we believe this system if applied in a crowdsourcing system, can improve the COVID-19 and other complications much faster than PCR tests.
The rapid growth of the CT Scan images database size in a very short time from the start of the project gives us this perspective that before the data collection gets to a saturation point, will collect thousands of images, that is well enough for accurately detecting COVID-19 cases along with other pulmonary complications in one year.
The crowdsourcing effect, along with the algorithm can improve the mechanism and add new dimensions to it in 1 to 3 years, making it an efficient and easy to access CT scan images analyzing system accessible online.
The provided mechanism has improved very rapidly with the help of various centers and help of the crowdsourcing method involved in the data collection process. The current accuracy of the COVID-19 screening algorithm varies based on the clarity, quality and size of the CT scan images, but with the increase in the amount of the training data, and improvement of the modeling the algorithm can successfully detect any abnormalities automatically.
- United States
- United States
There are four major barriers in the process of accomplishing goals which can be listed as below:
1. Having to deal with different sizes, and accuracies of CT scan images
2. Difficulty in finding more CT scan images to improve training databases.
3. Backend processing and computation resources
With the aid of the crowdsourcing mechanism embedded in the project more CT scan images, with different types will be collected with will automatically improve the training mechanism. Financial help can help in acquiring more processing machines, or cloud-based scaleable processing power for backend processing.
- Nonprofit
New England Complex Systems Institute
One of the main barriers in the expansion of the project is the difficulty of access to data sources and computational power, along with the research hours. Our expenses include dedicating research hours to this project, as well as computing costs utilizing AWS. We estimate such costs at $48,000. The Trinity Challenge can help us overcome these barriers for us.
Not any yet.
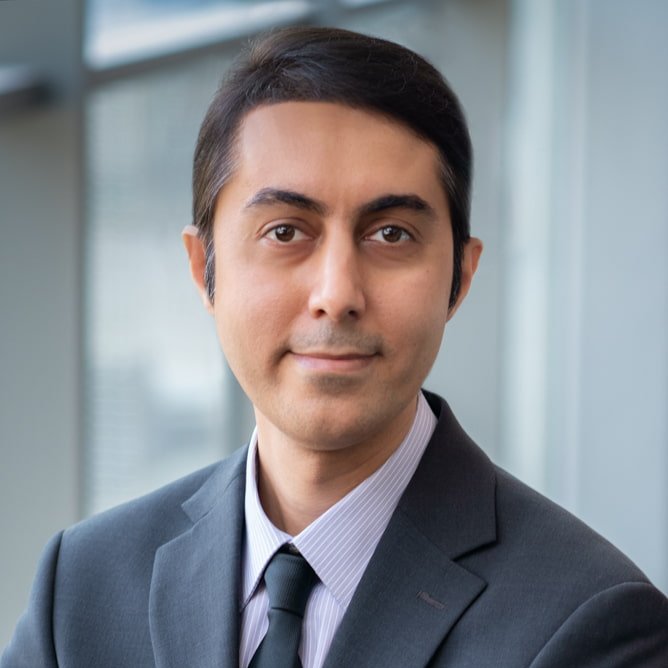
Researcher
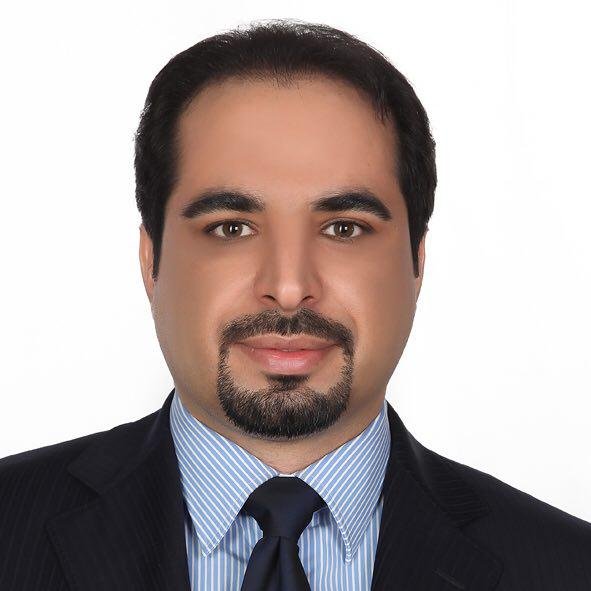