Chitra: Visualizing Community Health
Supporting data-driven localized healthcare provision by community health workers using a mobile mapping, analytics, and visualization tool.
THE CHALLENGE: Around 1,000,000 community health workers (CHWs) collect demographic, environmental, and medical data across India, using paper forms. Despite huge efforts to collect data in the first place, this data is currently underutilized. There is a huge scope for (1) digitizing the data collection process, and (2) analyzing collected data to help healthcare providers deliver personalized care to communities. Particularly, low-resource communities that rarely access hospitals and clinics could benefit greatly from targeted interventions.
OUR SOLUTION: To meet this need, we propose Chitra - a mobile application that (1) helps community health workers (CHWs) collect hyperlocal data, and (2) builds community profiles by applying machine learning on collected data. These profiles include information about potential diseases that may exist in the community or are at risk of manifesting, and suggestions to mitigate these risks. This can help CHWs and healthcare providers provide personalized care to communities.
WHY CHITRA? Health and health-seeking behaviors are a product of environmental, socioeconomic, and cultural factors. The current healthcare system follows a one-size-fits-all approach and provides band-aid solutions that provide a cure but do not address underlying reasons for disease. Recording and understanding these location-based factors can help develop health interventions that take into account these aspects and community resources. This can result in improved health outcomes, particularly among underserved communities that are most affected by their surroundings. Outcomes that Chitra can result in include organizing community members to improve sanitation in their area, install toilets and clean drinking water, and convincing community members to vaccinate.
HOW DOES THIS WORK? We build upon existing open source mapping tools such as Local Ground and ODK to enable hyperlocal data collection using map annotations. We aggregate collected data based on location and then apply machine learning algorithms to help identify communities that are at risk of disease and the underlying factors that may be responsible for the threat. User-friendly insights are presented as community profiles to health providers such as doctors, community health workers, and policymakers to help them make data-driven decisions. The project thus leverages and supports healthcare providers' existing competencies and deep understanding of local communities.
EXAMPLE? The current common preventative approach to dealing with malaria is distributing mosquito nets, which are often not user-friendly and require behavior change and intensive resources. The curative approach is for community health workers to refer patients to local clinics, where community members often do not agree to go due to the cost, time, or belief in herbal and traditional treatments. Chitra provides an alternative solution. A health worker who knows and has on record (on the mobile application) that malaria in a community is being propagated by the stagnant water, can now inform local authorities to take action and also motivate the community to fill this in with sand. This addresses the root problem, providing a long-term sustainable solution. There is a lack of a medium for aggregating and presenting such information in a user-friendly way so that health workers have the knowledge, legitimacy, and power to affect such outcomes.
- Workforce training, recruitment, and decision supports
- Coordination of care
- Other (Please Explain Below)
Global organizations such as Medic Mobile, DHIS2, Dimagi, and Nafundi focus on data collection tools, often for community health workers. However, current efforts to analyze collected data rarely go beyond recording disease outbreak and service coverage. CHWs also do not get to use the population health data (beyond coverage statistics) and rarely receive qualitative feedback. This results in underutilization of rich collected data despite massive efforts to obtain them in the first place. We go beyond current efforts that are focused on data collection to also analyze the collected data and make hyperlocal disease predictions and healthcare delivery recommendations.
Our core competency lies in the use of machine learning techniques to provide customized care. We build upon existing open source mapping tools such as Local Ground and ODK to enable hyperlocal data collection using map annotations. We aggregate collected data based on location and then apply machine learning algorithms to help identify communities that are at risk of disease and the underlying factors that may be responsible for the threat. User-friendly insights are presented as community profiles to health providers such as doctors, community health workers, and policymakers to help them make data-driven decisions.
We are currently conducting codesign with community health workers (CHWs) to determine appropriate visualizations and modalities for mapping and presenting community health profiles. Using these insights, we will develop a mobile application (3 months). After training (2 weeks), we will carry out mapping exercises with 100 CHWs and 100,000 people (2 months). We will tune machine learning algorithms using this data (2 months). Finally, we will monitor healthcare provision activities during deployment (5 months).
Our vision to scale is two-fold:
1. To partner with data collection organizations and integrate with their technology. Our current prototype already builds on Nafundi’s open-source technology (Open Data Kit).
2. To develop strategic partnerships with governments to bring mobile data collection to regions currently relying on paper-based surveys.
Both of these will help us scale with each partnership. Additionally, with the increasing penetration of mobile phones and highly affordable rates of mobile data in India, the region is an excellent fit for this solution.
- Urban
- Lower
- South Asia
- India
- India
We are closely working with community health workers (CHWs) and slum communities in Delhi on this project. We follow an iterative process, taking input from CHWs on the functionality, design, and appropriateness of our proposed intervention. Our proposed solution leverages the existing data collection activities of CHWs by digitizing the process and providing CHWs with detailed feedback on the health of the communities they serve.
We have conducted participant observation with community health workers to better understand their interactions with local communities and the challenges they face. From January to May 2018, we deployed an initial data collection prototype with 5 community health workers and 600 slum residents. The collected data is being analyzed and preliminary machine learning algorithms have been developed. In February 2018, we also conducted 2 co-design sessions with 17 community health workers for the mobile application. Using insights we gained during the co-design process, we have developed a design for the mobile application, on which we are currently collecting user feedback.
Users: Community Health workers (CHWs)
Beneficiaries: Slum communities
Impact measures:
Two health interventions per CHW per month
Increase data collection frequency and coverage by 10 percent
Increase immunization and maternal care coverage by 5 percent
We will increase the number of CHWs we reach by partnering with data collection organizations. They already cover more than the population we cite below:
1 year: We expect to reach 100 CHWs, at least 30,000 households, and a total population of over 100,000 people.
3 years: We expect to reach 7000 CHWs, at least 2,100,000 households, and a total population of over 8,000,000 people.
- Not Registered as Any Organization
- 2
- Less than 1 year
We have a combined 8 years of experience on healthcare technologies in the Global South.
One member is joint faculty in the School of Public Policy and the School of Interactive Computing at Georgia Tech. We will leverage these connections to develop partnerships and gain technical expertise.
The other is a PhD student in Human-Centered Computing at Georgia Tech. She has industry and research experience working on projects leveraging machine learning, including health applications. She has conducted prior research from 2016-2018 on the healthcare infrastructure in the region of deployment and has been in constant touch with health workers there.
To achieve scale and sustainable growth, we will be partnering with organizations such as Dimagi, Nafundi, and Medic Mobile. This will allow us to integrate with existing data collection technologies which are already in use around the globe. We will be helping these companies achieve their objective of increased population coverage, in addition to enabling new kinds of healthcare provision for population health. We will provide this service for a percentage in their profits from each deployment. Thus, we are well positioned for expansion.
We will also provide our service to governments and health organizations at a fee per health worker.
In both these cases, we will provide ongoing technical support in the form of a yearly subscription to generate a long-term revenue stream.
As a non-profit, we will also be approaching foundations and individuals with the results of our deployments, for funding.
Our solution addresses the last mile in healthcare delivery by supporting the efforts of overworked and under-trained healthcare workers. This fits with Solve's challenge to provide solutions for the frontlines in health, we are literally arming frontline health workers with better tools!
We think that the resources and partnerships Solve can provide would go a long way in accelerating our progress and achieving sustainable impact. We are also looking forward to learning from the successes and failures of existing health and technology organizations in this space.
Key barriers we anticipate that Solve's network can help us with:
1. Adequate data and mobile literacy of community health workers and mobile and data storage resources in partner organizations. We will conduct training sessions for the community health workers and Solve's network will be advantageous in locating the right resources for the latter.
2. Developing strategic partnerships for expanding growth within and beyond Delhi, India.
3. Developing a deployment structure that is widely applicable, with results being replicable elsewhere, in the absence of us providing merely technical assistance and no human support on the ground.
- Peer-to-Peer Networking
- Technology Mentorship
- Impact Measurement Validation and Support
- Media Visibility and Exposure
- Grant Funding
- Other (Please Explain Below)
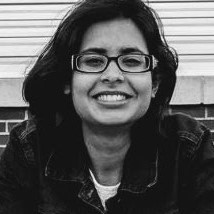
Cofounder at MakerGhat, and Assistant Professor at Emory University