Breath based COVID-19 detection
The system will detect indicators of possible COVID-19 infection based on the volatile organic compounds (VOCs) found in human breath using existing biosensors. These sensors can measure the compounds relevant to the pathogenesis and asymptomatic effects of COVID-19 infection. This system would allow untrained personnel to conduct real-time (quick), noninvasive COVID-19 detection that is inexpensive and generates minimal biohazard waste. It would only require a person to provide a breath sample that will be analyzed within a few seconds to provide a COVID-19 "respiratory infection score." This system would enhance the safety of enclosed spaces like airports, airplanes, hospitals, shopping centers, and buildings globally.
Current COVID-19 detection methods are labor-intensive and require a considerable interaction between people that present some risk of adverse side effects (eg., spreading the disease to either or both). Samples often need to be collected through a somewhat invasive procedure (eg., swabbing nose/throat samples or blood samples via finger prick). These procedures often require trained personnel, specialized devices, reagents, and certification (i.e., CLIA) while also taking between several minutes and days to obtain a result.
In comparison, the human breath can be easily sampled repeatedly and analyzed in real-time for a noninvasive method to measure a patient's physiological status. It is also relatively inexpensive and generates minimal biohazard waste.
This system innovatively combines technology from several disciplines into a complete and integrated system. The breath phase is correctly sampled and processed for sensors to normalize against background noise and other environmental factors. This standardized breath sensor data, combined with other sensor inputs, utilizes a deep learning neural network to risk score COVID-19 infection that is globally scalable, standardized, and compatible. This global database of breath sensor data improves over time, accounting for global variances and human conditions, while having the additional benefit of detecting known and unknown future respiratory pathogens.
This system leverages e-nose technology to detect COVID-19 with high accuracy, sensitivity and precision, quick response, and fast system recovery time to be utilized by untrained individuals.
The sampler implements breath partitioning to purge and trap multiple breath samples and flushed before each use. An individual breathes into a disposable mouthpiece, and an automatic 4-way valve collects "alveolar air" while maintaining a fixed temperature and humidity.
The sensor array is sensitive towards several VOCs, and several analytes (i.e., NOx) often associated with several respiratory diseases such as SARS, URTI, and ARDS in concentrations within the sensor detection limits.
The system contains a secondary sensor array to measure exogenous VOCs to establish measurement baselines to eliminate environmental pollution readings, bias, and other "noise."
Additional sensors measure an individual's CO2 levels, temperature, and O2 saturation, while external sensors measure environmental data, including temperature and humidity.
The system utilizes a recurrent neural network with reinforced learning to identify VOC biomarker combinations correlated with COVID-19. This normalized breathprint data is combined with other sensor data, environmental data, and individual information (e.g. age, gender) using a deep learning neural network to produce an overall individual "respiratory infection score."
The rapid and accurate detection of the COVID-19 infection is of paramount importance. With this solution, an individual simply provides a noninvasive breath sample, and the system will rapidly and accurately detect the presence of COVID-19. The system requires little staff training, is easy to use, uses minimal consumables, and generates little waste.
It is also possible to use this system to test for other respiratory illnesses and future pandemics. By detecting viral infection quickly, efficiently, in vitro and at the point of contact, this technology could detect and significantly halt the spread of COVID-19 and other respiratory diseases.
Eventually, using this technology, a person would quickly detect whether they have a respiratory infection by analyzing their sample against a global database. This technology could also be deployed at venues where people congregate, including hospitals, cruise ships, sports stadiums, hotels, convention centers, public buildings, malls, and restaurants. Ultimately, the system will become small and cheap enough for individual homes, automobiles, and mobile phones.
Pandemics will only increase in this globally interconnected world. Most pandemics (e.g., COVID-19, SARS, and MERS) are respiratory in origin and helps facilitate the transmission of disease globally. Using this mode of transmission, the system measures the breath of an individual for biomarkers associated with respiratory disease. In doing so, the system can detect for signs of COVID-19 disease but any other known and unknown respiratory diseases that fall off the "baseline" of normal human breath. This project aligns with the Challenge by improving solutions for prevention of COVID-19 through accurate detection, and rapid response.
- Prototype: A venture or organization building and testing its product, service, or business model
- A new application of an existing technology
This system uses a technique which measures the volatile organic compounds (VOCs) present in an individual's breath for biomarker signatures associated with COVID-19. A person blowing noninvasively through the device can automatically be sampled repeatedly and analyzed in real-time. This system's disruptive nature would displace existing technologies, such as PCR, for real-time detection applications.
Research in e-nose sensor technologies have already detected, with greater than 95% accuracy, various respiratory diseases in clinical settings.
This project innovatively combines technology and data from several disciplines that focus on replicability by developing standards to facilitate compatibility, consistency, and repeatability. The sampler automatically cleans, calibrates, and effectively collects the relevant breath VOCs from an individual. The samples are sent directly to a sensor array in a consistent and controlled manner. The primary breath sensor array is tuned to detect endogenous breath VOCs while a secondary sensor array measures exogenous VOCs. Other sensors measure the person's vitals (e.g., temperature, CO2, pulse) and local environmental data (e.g., temperature, humidity, barometric pressure). Data from both VOC sensor arrays are processed using a neural network to remove environmental background noise from the breath VOC sample. This data is combined with the vital, environmental, and individual data (e.g. age, gender), using a hybrid graph neural network (GNN) to develop an overall "respiratory infection risk score." The complete system would be comparable in size and shape of a suitcase.
Sampler
The samplers uses VOC-filtered room air to flush the sensor chamber to reduce interference from ambient VOCs. An individual breathes into a disposable mouthpiece where an inline spirometer provides an expiratory resistance to standardize sampling flow. An automatic 3-way valve removes the initial phase of "dead space" air volume, and the next phase of "alveolar air" is captured for analysis. CO2 sensors measure for this alveolar phase change. The sensor chamber maintains a fixed temperature and humidity.
Sensor system
Breath samples are analyzed using an "electronic nose" consisting of polymer composite sensors that are measured at ~1 Hz.
Environmental controller
Room air is pumped into a second sensor array to provide the baseline sensor response that is normalized and auto-scaled to eliminate background noise and exclude outliers.
AI/ML system
The breathprint data is sent to a recurrent neural network (RNN) with reinforcement learning allowing the model to retain memory over time and receive sequential inputs.
This model and additional temperature, O2 level, age (increasing oxidative stress-related VOCs), and gender (VOC differences) information is leveraged in a graph neural network (GNN).
Ancillary systems
The initial target environment for the system would be an airport, given its controlled environment, security mindset, and global need.
The system will utilize a passport scanner to retrieve personal information (i.e., age, gender) from the Passenger Name Record (PNR) system. The "health risk score" is further leveraged by other aviation industry systems to ensure the safety of other passengers, ground staff, and flight crew.
Hippocrates was said to use breath odor as a means of diagnosing patient disease and was first demonstrated by Linus Pauling over 50 years ago. In humans, typical exhaled breath gases consist of over 3,000 VOCs and analytes based on the direct exchange of gases between air and blood within the lungs.
Abnormal volatile metabolites (biomarkers) are often a result of oxidative stresses that result from internal hormonal or metabolic changes due to infectious disease, disease-predisposition, pathogens, and gut-microbiome activity. In respiratory diseases, infections often result in inflammation associated with immune cell response.
Each sensor within the e-nose sensor array undergoes a reversible change in electrical resistance when exposed to a VOC or analyte unique to the sensor material. This technology has already detected and diagnosed, with greater than 95% accuracy, inflammatory lung diseases such as acute respiratory distress syndrome (ARDS), asthma, chronic obstructive pulmonary disease (COPD), invasive pulmonary aspergillosis (IPA), malignant pleural mesothelioma (MPM), acute bacterial infections, pulmonary tuberculosis (TB), upper respiratory tract infections (URTI), and ventilator-associated pneumonia (VAP).
After the influenza (H1N1) pandemic of 2009, breath VOCs were used to measure the efficacy of vaccine inoculations. In these tests, measured biomarkers correlated and peaked three days after administering an attenuated H1N1 vaccine. Furthermore, several breath tests are approved by the FDA for clinical use, including tests for H. pylori infections and the asymptomatic detection of malaria.
For AI, the GNN models have achieved state-of-the-art results in the prediction of biophysical, biological, physical, and electronic quantum properties of molecules.
- Artificial Intelligence / Machine Learning
- Big Data
- Biomimicry
- Materials Science
- We are living in an increasingly interconnected and global world.
- Pandemics are occurring with increasing frequency.
- Most pandemics to date have been respiratory disease in origin. COVID-19 is not the first, nor will it be the last.
- Global air travel and direct human collaboration will increase (or has to) for the global economy to grow.
- Regardless of the economy, many of societal infrastructure designed today assume many people in close quarters where "social distancing" is not possible (i.e., airplanes, sporting venues)
- Early, asymptomatic detection of known and unknown respiratory diseases will become increasingly important.
- Given the respiratory nature, it is best to conduct a root cause analysis of the exhaled breath from the transmission source - the person's lungs.
- Systems will need to be accurate, fast, non-invasive, repeatable, inexpensive, and easy to use by untrained individuals.
- Tests will have to be cheap, should not require reagents or consumables that generate a lot of biohazard waste.
- Methods and techniques need to focus on data compatibility (standardization), consistency, and repeatability for global scalability.
- Components of the system should be modular for independent innovation and the ability to license to other stakeholders.
- Eventually, the system will become small and cheap enough for (in order of deployment) hospital bedsides, individual homes, automobiles, and mobile phones.
- Urban
- Low-Income
- Middle-Income
- 3. Good Health and Well-Being
- 9. Industry, Innovation, and Infrastructure
- 11. Sustainable Cities and Communities
- United States
- United States
COVID-19 detection with benefits provided by the system has global implications that would serve a significant population. Even with the initial targeting of airports and airlines, this system would potentially serve over 4.3 billion flying passengers (2018 ICAO statistics). Thus, the system would increase the health and safety of passengers, ground staff and flight crew and also facilitate the rebuilding of trust and confidence in global air travel.
Eventually, using this technology, a person would quickly detect whether they have a respiratory infection by analyzing their sample against a global database. This technology could also be deployed at venues where people congregate, including hospitals, cruise ships, sports stadiums, hotels, convention centers, public buildings, malls, and restaurants. Ultimately, the system will become small and cheap enough for individual homes, automobiles, and mobile phones.
Within the next year: Have a functioning system that can be deployed in key use cases.
Five years: Broad deployment of systems for use in a various applications and settings.
- Obtaining COVID-19 samples for sensor calibration and AI database training.
- Funding to create the first prototype device.
- Next level funding to create commercial device.
- Scaling and global expansion funds.
We are currently pursuing many funding sources, including government grants, SBIR loans, and angel/VC funding. We have also applied for NIH grants that include the assistance in COVID-19 sample gathering.
We will also look at various partnership opportunities to help offset costs and/or leverage experience.
After the prototype phase, we will investigate various equity financing forms, including strategic investments by likely stakeholders.
Post commercialization and international expansion would rely on regular equity markets for funding.
- For-profit, including B-Corp or similar models
2 FTE, 2 part time, the rest will be an unspecified number of contractors.
The company founder and team members consist of seasoned managers with a variety of technical experience and backgrounds. The company and its members have experience taking similar products and services from the concept phase through to full commercial deployment.
The company sees tremendous opportunities in bringing the proposed solution to the market. Initially, the aviation sector presents several advantages to help accelerate adoption and deployment. However, the core technology can be leveraged into many other industries and address a wide number of other issues/problems. Applications not only includes the detection COVID-19, other respiratory diseases (known and unknown), but also applications as broad as public safety and law enforcement. The company currently works with several organizations which include aviation-related companies.
Other members have extensive experience in the field of information security, other aspects of the airline industry, and decades in product design and UI/UX experience. Members also have extensive experience in fundraising, prototyping, commercialization, scaling, marketing, sales, and customer support.
N/A
The initial phase includes developing a system that can screen passengers in or around existing airport security checkpoints, the system can leverage the unique characteristics of an airport, its inherent security focus, the availability of passenger data, and its role in global connections. In this initial "aviation phase," the system would expand existing airport security checkpoints and become an additional "health security check" layer for passengers before boarding or entering the airport.
Given the broad deployment possibilities of this technology, once the implementation of the initial phase has begun, licensing the design, core system, software engine, and/or components to existing manufacturers of aviation systems such as walkthrough metal detector and body scanning manufacturers becomes more efficient. Revenue opportunities may also include licensing, maintenance and certification fees to ensure compatibility.
Upon initial acceptance and deployment into the aviation market, the system continues to collect standardized breath measurements from around the world, including various environmental and human conditions. Thus, this database of historical sensor data becomes the "barrier to entry" from even large competitors. Ultimately, following the "aviation phase," other industries and markets are addressed through the aforementioned licensing model. All certified systems can utilize this database to obtain accurate measurement information while further increasing the database's accuracy and the collective systems that utilize it. Therefore, the penultimate business model is to provide access via an MRR based subscription model that leverages this database, creating a network effect of breath sensor data regardless of the application deployed by industry-specific leaders in different markets.
- Organizations (B2B)
We are currently applying for federal funds and various grants to demonstrate that this technology works for COVID-19, and then build reliable prototypes that can be scaled up for mass production and field deployment. One a prototype can be developed, we will seek pre-A round VC funding and strategic investments from companies that are in targeted industries. Eventually, revenues will be based on sales of systems and license of module/components.
This project will focus on the optimization and integration of:
- Sampling
- Sensors
- Environmental factors
- AI/ML
- Commercialization
The selected sampler methods and sensor technology will need to optimize for sampling requirements, equipment size, speed, need for reagents, sensory recovery time, and signal-to-noise ratio.
The selected sensor array will need to optimize for sampling size, sensor performance, and sensitivity towards relevant biomarker VOCs. The sensor array can detect VOCs and several analytes (i.e., NOx) often associated with several respiratory diseases such as SARS, URTI, and ARDS in concentrations within the sensor detection limits.
The system contains a secondary sensor array to measure exogenous gases and VOC concentrations to establish measurement baselines to eliminate environmental pollution readings, bias, and other "noise." Signal and noise will require optimization.
COTS-based sensors measure an individual's CO2 levels, temperature, and O2 saturation, while external sensors measure environmental data, including temperature and humidity. This information also needs to be optimized for use in the AI/ML system.
- Solution technology
- Funding and revenue model
- Other
- Obtaining the proper COVID-19 samples at a statistically appropriate level to test sensors and develop/training the AI models.
- Gaining the assistance for entering initial health parameters of a given sample and to classify data for the AI algorithm.
- Trained, debugging, analyzing and tuning the AI algorithm.
- Validate and test the AI model against real results based on existing COVID-19 testing procedures.
- Faculty with multi-label classification problem experience in AI.
- Organizations with gas sensor and sensor system experience.
- Eventually, organization with commercial fluid and environmental engineering experience.
This project is transformational and has a powerful and tangible outcome if successful. It is purpose-driven as it addresses a vital industry initially for its unique qualities, but can expand into other industries after that. Finally, this project is at a tipping point where the work is well-positioned to inspire others globally, but if successful, it will allow everyone to reconnect physically.
The impacts of this project are clear, and the components that make up the system have already been proven feasible. Obviously, with the success of the system, scaling is the next step to have a broad impact globally. One of the project's main goals is to make the data consistent and repeatable to facilitate scalability.
The team has many decades of experience in taking ideas and innovative concepts through development and commercialization. The project is an exemplar of innovation representing both application and product innovation. It takes existing technologies and reduces cost, improves quality, and enhances functionality for a new COVID-19 market to address a critical need.
Given the market opportunity and size, partnership potential is tremendous but would require exposure to a broader audience and resources offered by the Elevate Prize.
This project will utilize a recurrent neural network (RNN) with reinforcement learning allows the model to retain memory over time and continue to receive sequential inputs of breath biomarker data.
As the system utilizes other pieces of non-breath data, the dataset evolves into a multi-label classification problem. Representing all this data into graphs, a graph neural network (GNN), designed to operate on graphs as input, will directly predict the likelihood of COVID-19 infection without using any handcrafted rules.
However, besides the methods and techniques used to obtain consistent temperature, humidity, and sampling rates, the AI models need to focus on enhancing compatibility (standardization), consistency, and repeatability. This component will be necessary for the global scalability of the system.
This project qualifies for the People's Price as it addresses two UN Sustainable Development Goals. Specifically, goal 3.D of strengthening the capacity of all countries through early warning, risk reduction and management of national and global health risks via early detection of COVID-19.
Furthermore, goal 9.1 to develop quality, reliable, sustainable and resilient infrastructure, including regional and transborder infrastructure, to support economic development and human well-being, with a focus on affordable and equitable access for all by proving a fast, cheap and easy method for COVID-19 detection at transborder venues.
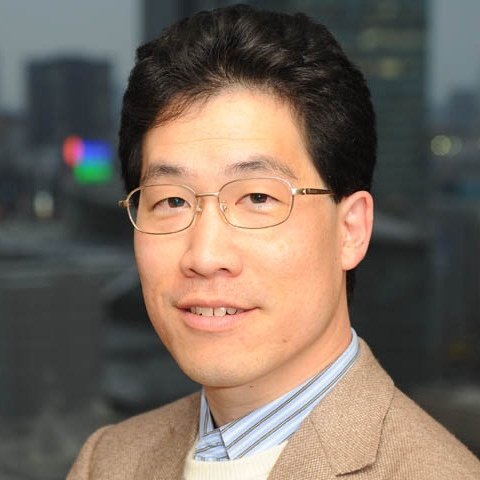
CEO