EWOR AI
Today, even workers with decades of professional experience see their jobs wiped away by new technologies. Often, their lack of digital literacy keeps them unemployed. Our current education system, i.e., 'studying in the first part of our lives and working in the second part,' is partly responsible. Learning must become an everyday practice to cope with the fast-paced digitalized world.
Paradoxically, high-quality content about new digital tools and methods is vastly available on the web, for example, via edX and KhanAcademy. EWOR's AI-powered adaptive education recommender system scrapes, orders, and presents high-quality educational content to enable everyone's continuous personalized learning.
EWOR AI's algorithm finds many applications: It can scrape and compile Bachelor's and Master's degree equivalents from the web. It can assemble skill-transformation journeys for corporate employees. EWOR AI tailors its content to the individual based on their strengths, preferences, goals, achievements, and in-app progress.
Who: Gartner stated in December 2019 that someone, somewhere in this month, started a new job without realizing that they have become the billionth knowledge worker. The global need for knowledge work (which requires higher education) has doubled from 1980 to 2015.
What: Knowledge work is fragile. It builds on the usage of cutting-edge techniques, tools, and technologies, many of which become outdated and replaced quickly due to today’s rapid speed of change. It has become increasingly difficult for workers to cope with a high velocity of change, to attain durable skills, and stay up-to-date.
Why: An analysis of Jones (2012) with over 80.000 students shows that congruence of personality and major predict GPA better than ACT scores. EWOR believes that knowledge workers face a similar problem. Lifelong learning needs to be fun. It needs to fit the individual. Simply exposing knowledge workers to the vast educational content on the web leads to paralysis (choice overload) instead of action.
EWOR AI connects learners with the right educational content at the right time. It is based on three technologies.
Content aggregator: It gathers, modularizes, and orders publicly available education content. Initially, the aggregator focuses on select subjects (computer science, data science, and entrepreneurship). Later, any conceivable subject will be covered. The platform’s early version exclusively aggregates content from select educational content providers, including Google Books, Coursera, and Khan Academy. Most importantly, EWOR makes sense of the concepts and orders them appropriately.
Preference discovery engine: EWOR ensures that the recommended contents suit our learners’ preferences, abilities, and needs. We approximate individual preferences based on micro-surveys and in-app behavior. Moreover, our engine continuously suggests “lateral” ideas from topics not yet covered. Our application will become every learners’ personal “mentor”: guiding them through the relevant educational content towards their goals.
Content Designer: Our learning management system (LMS) will allow paying B2B partners (educational institutions and corporates) to host and manage their content. Affiliated learners have exclusive access to “local content” which is seamlessly combined with “global content” in a single, easy-to-use interface. Through our digital foot-prints, we compile skill profiles to help businesses identify the right workers for the right tasks.
The one billion knowledge workers across the globe are our focus. Our beachhead segment is workers in especially fast-paced markets: Tech entrepreneurs. Our pilot customers were particularly eager to learn new skills hands-on that help them get a competitive advantage in their field and were concerned that university education already uses outdated content.
We have engaged these learners over several months in different activities, for instance, by creating map-like structures to visualize entrepreneurship content. Our learning map functioned like a ‘Blinkist’ for education powered by visualization techniques. Tracking and watching people’s engagement with our map made us aware of its usefulness compared to rigid curricula.
We realized the next step is a completely dynamic ‘Google’ for education. Our new Google-like solution gives workers a tool to cope with the ever-changing world as content is scraped directly from the web and is rated for its usefulness. We have built Wizard of Oz Prototypes to test users' reactions. Our learners rated the experience higher than the learning map, and asked for more content immediately after completion.
As our learning journeys are adaptive and flexible, they ensure that knowledge workers can continuously learn up-to-date, relevant content next to their jobs.
- Equip workers with technological and digital literacy as well as the durable skills needed to stay apace with the changing job market
Vast educational content exists on the web, but individuals struggle to find the right resources to navigate through a fast-changing job market.
EWOR’s AI-powered learning engine equips knowledge workers with technological and digital literacy by creating adaptive individual learning journeys based on their strengths, preferences, goals, and in-app progress.
For example, if an individual in Lagos wants to start an eCommerce platform, they could learn accounting, web development, and product management in a personalized manner to the degree they wish through EWOR AI. We will display overarching concepts and their relation, but link them to high-quality existing educational content.
- Prototype: A venture or organization building and testing its product, service, or business model
- A new application of an existing technology
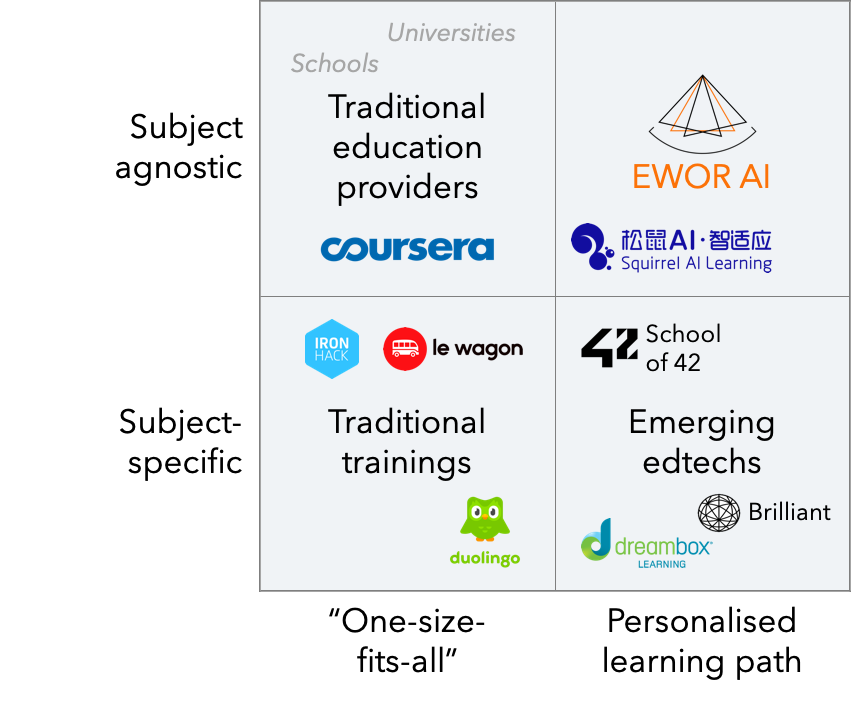
While we are unaware of any competitors who use a similar education-focused content aggregation model in the West, there is a Chinese competitor: Squirrel AI. Their fuzzy-logic engine crawls content from digitized education books. It creates an ontology of “subjects” and “concepts” (~30.000 to date), which are fed to individuals based on their background, learning preferences, and in-app progress.
EWOR adapts this idea to non-Chinese learners and extends it to include the abundant learning resources available on Youtube, Khan Academy, and similar platforms. Our algorithm orders, modularizes and recommends all of these concepts to users based on their strengths, preferences, goals, achievements, and in-app progress. As learners progress in any given subject, more demanding content will be recommended.
Its innovative character comes from the combination of a content-agnostic aggregator with an AI-powered preference discovery engine. This combination enables the creation of an individualized learning journey for every learner: adjusted according to the required depth of content (e.g., KhanAcademy vs. EdX Micro-Masters), learning type (e.g., auditory vs. visual), and a variety of other factors. Our way of finding, ordering, and visualizing educational content is truly unique, more effective, and applicable to life-long learning. Instead of merely displaying one type of content (as in MasterClass, Coursera, EdX, Udacity, and KahnAcademy), our approach works as a hyper-layer for educational content that amplifies the reach of existing providers and does not compete with them.
Our competitive analysis map is attached.
EWOR AI aims to connect learners with the right educational content at the right time. To present the individual with a viable learning journey, EWOR AI builds three core technologies that combine existing cutting-edge tools:
Content aggregator
Web scraping: Pre-selected, indexed educational websites are scraped for their content based on cutting-edge web scraping techniques.
Filtering: Based on a variety of hard factors, specific content is filtered. We use a logical, structured framework for filtering while applying probabilistic machine learning models for our further recommendation framework.
Preference discovery engine (AI-powered recommender system)
PMF and RBM: Based on an individual's and other users' ratings, we use a probabilistic matrix factorization model, such as the one used by Amazon, to identify content with a high likelihood of being rated positively by the user. We pair our PMFs with generative, stochastic neural nets to improve performance (restricted Boltzmann machine (RBM)).
Content Designer
Our ontological system adds flexibility to our LMS. Different keywords are tagged with several (often overlapping) attributes, such as analytical, soft-skill, creative, mathematical, etc. Content designers, such as HR departments or lecturers, can add preferences based on these keywords for automatically scraped learning journeys.
Moreover, content creators can design full educational degrees, which follow a structure but offer certain flexibility. For example, an HR representative may design a data science course for marketers with 20% mandatory components and 80% electives, close to data science, but chosen by and/or tailored to the individual learner.
There are two dimensions of evidence:
First, EWOR has tested users’ responses and the adoption of the initial product. We have already built an educational product before, a three-month educational entrepreneurship program that educated tech entrepreneurs (our initial beachhead segment) through a learning map instead of a curriculum. The learning map, primarily linking to existing educational resources on the web, was liked and actively used by our learners. Yet, as the learning map grew, it became increasingly difficult to use. This inspired us to create individual learning journeys. We have tested a first MVP, which presented our learners with an individual journey (admittedly having used Wizard of Oz prototyping), which all of our learners adopted and rated highly.
Due to the innovative character of our product, its elements have only been proven effective in isolation. Probabilistic matrix factorizations have been a useful tool to recommend different products to different people. Datasets with a multitude of entities in scope, but very limited ratings per user, such as ours (i.e., a very ‘empty’ matrix with users on the horizontal and educational concepts on the vertical), PMFs have been proven especially useful. For example, Salakhutdinov and Mnih (2008) from the University of Toronto were able to trump the Netflix recommender system’s error rates by combining an RBM with a PMF model. Tarus, Niu, and Mustafa (2018) did a meta-analysis of recommender systems in the education space. They demonstrated that collaborative filtering techniques work well for knowledge-based recommender systems in e-learning.
- Artificial Intelligence / Machine Learning
- Big Data
- Software and Mobile Applications
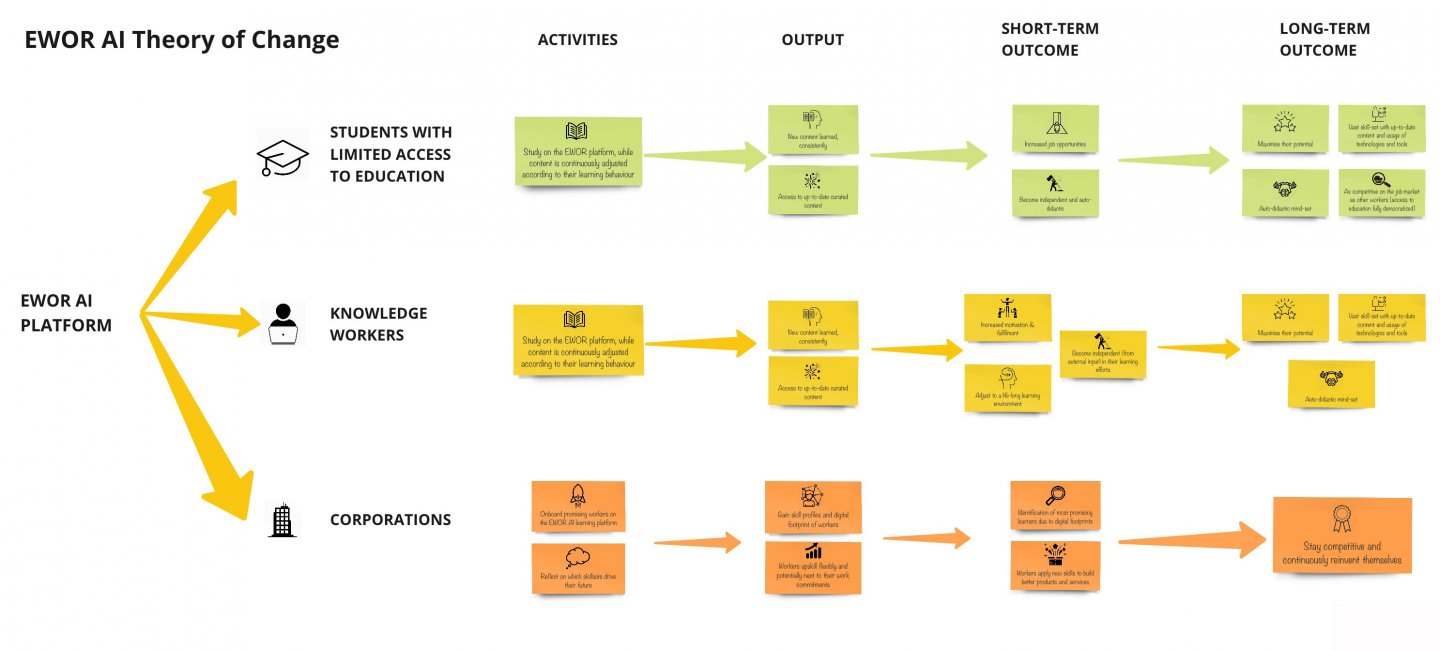
EWOR AI aims to connect learners with the right educational content at the right time. Through its unique content aggregation and preference discovery engine, EWOR AI presents the individual with a viable personalized learning journey.
EWOR AI uses the same core technology to address different target groups of learners:
1) workers who aim to develop relevant skills and stay ahead in a fast-changing world;
2) corporations which seek to equip their workers with the newest relevant skills, and keep reinventing themselves;
3) students who have limited access to education.
The attached Theory of Change visual showcases how EWOR AI would impact the different levels of change across the three target groups.
We believe that EWOR's long-term outcome results in auto-didactic, life-long learning knowledge workers who maximize their potential and job security. We furthermore plan to have democratized education for the ones who did not have access to it. Finally, we will have brought companies to the forefront of innovation successfully.
- Rural
- Peri-Urban
- Urban
- Poor
- Low-Income
- Middle-Income
- Refugees & Internally Displaced Persons
- Minorities & Previously Excluded Populations
- Persons with Disabilities
- 4. Quality Education
- 8. Decent Work and Economic Growth
- 9. Industry, Innovation, and Infrastructure
- 10. Reduced Inequalities
- Germany
- Netherlands
- Switzerland
- United Kingdom
- Austria
- Chile
- Germany
- Netherlands
- Portugal
- Spain
- Switzerland
- United Kingdom
While our solution is based on a unified platform, we plan to offer different versions for the distinct customer groups: A free B2C version for learners everywhere, and an affordable B2B version for corporations.
For our B2C solution, four learners have currently gone through our complete three-months innovation education program, which used the aforementioned learning map and rigorously tracked user behavior. Moreover, forty people have tested the map for our statistical analysis. Within the next year, we plan to scale to 10,000 learners. The number 10,000 is derived from an analysis of our distribution partners, who reach an audience of 2.5 million people across Europe. Based on previous work with them, we calculated with a conversion rate of 0.004, which results in the numbers above.
We believe that during the first year, active marketing through our partners and search engine advertising will be crucial to fuel initial adoption. Afterward, we plan to focus on driving word of mouth through enhanced product optimization by advanced analytics and customer research.
For our B2B solution, we have convinced four corporations to partner with us to test our learning journeys for digital literacy. This will result in reaching 400 workers in the next year. Eventually, we are convinced that our product will appeal to the increasing number of knowledge workers worldwide and may thus, either in its white-labeled form for universities and corporations or in its pure web application, crack the billion-user mark.
During the next year, we aim to have finalized and validated the EWOR AI platform, as well as to have achieved initial adoption. This should render EWOR financially sustainable and lay a good foundation for approaching impact-focused venture capitalists and government grants to fuel our growth. During the next five years, we foresee two unique use cases of the platform.
Our first product will be a free tool (B2C), which helps learners from all over the world to compile individual learning journeys. This product stream is built to drive mass adoption and brand recognition across the globe and to aid especially individuals who struggle with digital literacy and access to higher education. Our goal is that this product will have spread to hundreds of millions of people in India, Africa, South East Asia, and South America.
Our second product focuses on the global advanced training market, which is ~370 BN $ in volume. Corporations have an increasing need to find relevant content to upskill their employees to stay competitive. Our goal is to close 100 large corporations within the next five years through which we can reach at least one million active users.
Our main focus during the next five years is to have achieved great adoption for both products and consistently improve the platform experience for our users, as well as to have a global reach. This will enable us to build the world’s most adopted education hyperlayer and serve billions of learners.
Barrier 1: Talent
In the immediate future, our main aim is to validate our AI-enabled learning journeys and prototype and validate our learning management system. While we have developed strong hypotheses, it is crucial to answering questions like “What is the optimal user interface?”, “Who are our loyal customers?” and “Are customers willing to pay for this solution?”. We aim to recruit a team of four engineers, one designer, one marketeer, and one commercial analyst by the end of the year to build, iterate, and learn until our solution is fully scalable.
Barrier 2: Access to paying customers
While we drive global adoption through our free web application for individuals, on our journey towards financial sustainability, one of our primary customers is corporations. Especially at the beginning, we know corporations are the most financially promising segment. To succeed, we need to gain access to tier-1 customers. For that, we need to convince them to replace current (manual and digital) training solutions with our application.
Barrier 3: Broad adoption of digital learning solutions
To maximize our impact, we hope to broaden our adoption reach to individuals that don’t have access to quality education in the first place to democratize education for groups left-behind. A potential barrier is reaching those individuals and creating a solution that would seamlessly fit their unique cultural setting.
Barrier 1: Talent
We are well-positioned to recruit the right talent to build a truly world-class solution. The primary talent pools that we are currently tapping into are (a) the University of Cambridge, the University of St. Gallen, and ETH Zurich, as well as (b) the Kairos Society Europe network (a talent network with 700 members). To fund our employees, we seek to take on outside investments. We have already received early interest from various Angel investors - and hope to gain further interest from the MIT Solve network.
Barrier 2: Access to paying customers
EWOR’s initial product - corporate innovation challenges - has proven to be a promising route to win initial customers for our technology platform. Several of the firms we previously worked with indicated a keen interest in piloting the new digital platform. This will not only provide valuable feedback but also serve as initial references for further customers. Once we have a proof of concept, we will leverage the team’s networks (e.g., previous employers, universities) to expand our customer base.
Barrier 3: Broad adoption of digital learning solutions
To reach a higher number of learners in-need, we partake in global opportunities to create visibility. Moreover, while we have defined clear hypotheses around our platform, only real-world implementation will show how users interact with it. To optimize the learning process, our venture follows a “lean startup” method. We hope to uncover cultural idiosyncrasies in our target markets and iterate/pivot our application until we find a clear product-market-fit.
- For-profit, including B-Corp or similar models
Full-time and part-time staff: None
Contractors and other workers: 7.
The current EWOR team works on EWOR next to their work or studies putting in an average amount of 20 hours per week. Some of this work is compensated financially.
First, with a Master’s degree at the University of Cambridge and a Bachelor’s degree at the University of St. Gallen, I have experienced many facets of formal education. Second, I’ve spent years exploring alternate educational pathways-I’ve coached the Jiangsu Olympic Math team, completed dozens of online courses and created my own online academy. Third, I have gained significant experience in building and coordinating strong teams, as described in prior passages.
Bernhard Gapp, a former BCG consultant, was a founding team member of Tracr, a blockchain platform that tracks diamonds from mine to finger. The venture has received funding from DeBeers, among others. Bernhard brings expertise in organisational strategy and financial modelling. Bernhard has consistently questioned current educational approaches and identified gaps in the system.
Sebastian Jaeger has been building successful AI applications since over a decade. He is uniquely positioned to deliver our technical solution. Chris Geis-Schrör, who has a Master’s Degree in data science and has mainly focused on developing predictive models for STILL-group, joins him.
Vinzenz von Teufenstein has obtained his bachelor’s and master’s degree at the University of St. Gallen, Switzerland. He has led the St. Gallen Symposium, where he led a group of 30 full-time workers to organize a mid-7-figure event. As our head of business development, he brings all skills necessary to sell the EWOR LMS to corporations.
For EWOR, three groups of partners are in particular focus.
First, university and community partnerships, including foundations, have helped and will help us to distribute our product to individual learners. We have partnered with 30 university clubs across Europe so far, who spread the word about our product.
Second, we target corporations that buy the EWOR LMS to empower their workers to gain relevant digital skills and stay competitive. We plan to receive both high-quality user adoption and financial sustainability, thanks to corporations. So far, we have partnered with SGS, a 100.000 employee company from Western Switzerland, and E-BIKE Technologies GmbH, a German-based SME to pilot our product. We have two further customers on board for our Summer prototype.
Third, we want to partner with research institutions to power the technical development of the product. We plan to establish an especially close relationship with the department of engineering at the University of Cambridge. We have already found valuable advisors and supporters at the machine learning office there.
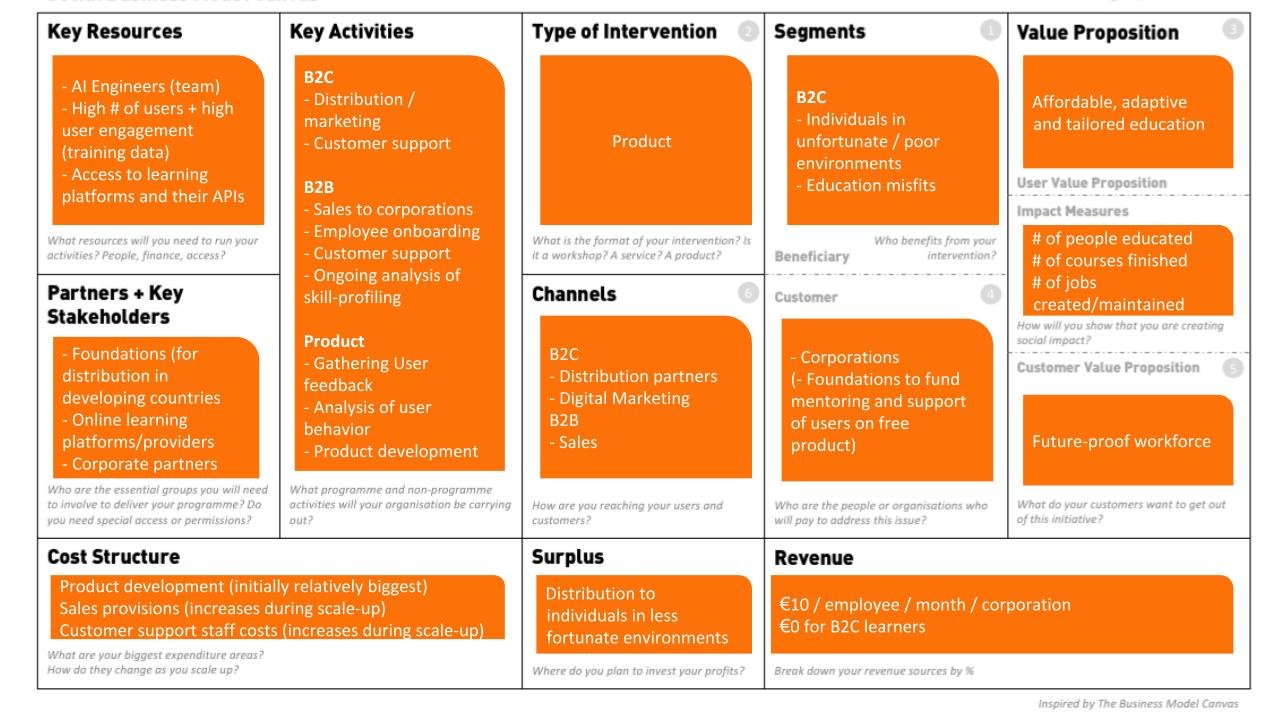
While our application will be based on a unified platform, we plan to address distinct customer groups.
EWOR AI (B2C): The basic version will be available to everyone for free (workers or students). Learners can download the app through the iOS App Store, the Google Play Store, or access it through a web browser. This version will offer all core features: A "learning journey" that connects learners with the right content at the right time. It will also make recommendations that help learners identify lateral fields they might be interested in. The corporate and the customer versions are released in parallel, with corporates covering the platform's development costs.
EWOR AI Corporate (B2B): Initially, we offer a paid version for corporations. This version lets corporations upload their own content (exclusively available to workers of their institution) and recommend further materials online. It also offers curated tracks for a company's skill needs (e.g., intrapreneurship or data science for business) and corporation-specific security features. Moreover, we track each employee's skill profile to help corporations find fitting candidates for difficult jobs easily. From our initial pilot cases with customers, this has been identified as one of the biggest benefits.
The large amount of training data gathered by our B2C model will help us improve the quality of the B2B product. Our B2C model will be distributed mainly through public relations and marketing efforts, while our B2B product will need a physical salesforce.
The Social Business Model Canvas is attached.
- Organizations (B2B)
We aim to develop our solution in two phases: (1) Development phase and (2) Distribution phase.
1) Development phase:
The path to an initial proof-of-concept requires extensive research and development efforts. Given our technology-focused approach, we will need a team of world-class engineers and designers to make our solution user-friendly and appealing. For that purpose, we plan to attract external funding from venture capital investors. Thus, we are looking for an investor that can provide not only funding but also expertise and a corporate network that will allow us to gain access to clients.
2) Distribution phase:
As soon as we have validated the product-market-fit, our organization will focus on the application's dissemination. In this phase, we will gradually sell our solution to paying customers (corporations). The proceeds will be used to expand the engineering team, sales force, and a broader support system. We will also offer a basic version for free to everyone, next to corporate usage, which would drive brand recognition and impact.
We envision that, as soon as the service gains sufficient traction, we will be able to cover further development and expansion costs through profits from our existing solution.
We were especially drawn to Solve because of its challenge of using innovative education for good jobs and inclusive entrepreneurship - two themes that are close to the hearts of the entire EWOR team. With our EWOR AI solution, we tackle both of them.
While our presence is strong in the DACH region (Germany, Switzerland, and Austria), we know that individuals and companies from the USA and beyond are particularly open to innovative approaches such as ours. Through Solve, we could reach a global audience of users and supporters, and spread global awareness of the unique personalized opportunities we provide for learners - both students and workers.
We are well aware that our social environment has a strong influence on who we are and become. At Solve, we hope to meet like-minded people and innovators from all over the world who will challenge our concepts and spark new ideas. Moreover, we find the Solve alumni network outstanding and hope, even though not explicitly mentioned on your website, that through merely becoming a Solver getting in touch with Priya Lakhani and other alumni who inspired us will become easier.
Lastly, we are convinced that Solve, thanks to its network and brand recognition, can help us attract further funding from the US and worldwide.
- Solution technology
- Product/service distribution
- Marketing, media, and exposure
Our team has significant experience in business modeling, raising money, and hiring outstanding talent. We believe that many of us have built outstanding networks, which will further help us with legal and business matters. This is why we have not selected these areas.
We believe that MIT and the Solve network are ideal for providing technological advice and mentorship. Many world-changing innovations have been developed at MIT, such as voice recognition by Ray Kurzweil or the world wide web by Tim Burners-Lee. There is no better place to rigorously dissect and improve our technology. We are also convinced that the Solve network may help us distribute our LMS to universities and institutions by both adding credibility to the EWOR product and actively reaching out to close partners.
Lastly, MIT Solve's strong brand and access to media, and thought leaders could help us market our product, especially our free B2C version.
MIT faculty can help us with our product's technical development. More specifically how to render our algorithms be more efficient; how to A/B test specific technological solutions and to implement new academic insights.
Moreover, we find the Solve alumni network outstanding. We hope to get in touch with other inspiring Solve Members in the Education and Good Job & Inclusive Entrepreneurship field, such as Priya Lakhani. We would be happy to both get feedback on our work and explore collaboration potentials.
Furthermore, we would like to partner with corporations who seek to empower their workers to gain relevant digital and tech skills and stay competitive within a fast-changing world.
Finally, once the product is finalized, we'd like to work alongside organizations (e.g., NGOs) who have a reach to learners who do not have access to (high-quality) formal education and would benefit from our product independently of their location.
EWOR's AI-powered solution connects learners with the right educational content at the right time to enable everyone's continuous personalized learning. Compared to people, our algorithm is not biased.
Today, even workers with decades of professional experience see their jobs wiped away by new technologies. Often, their lack of digital literacy keeps them unemployed. Our current education system, i.e., 'studying in the first part of our lives and working in the second part,' is partly responsible.
Paradoxically, high-quality content about new digital tools and methods is vastly available on the web. EWOR's AI-powered adaptive education recommender system scrapes, orders, and presents high-quality educational content to its learners.
EWOR AI's content-agnostic algorithm can assemble skill-transformation journeys for working adults to equip them with the most up-to-date digital skills. It can scrape and compile a Bachelor' s/Master's degree equivalents to help individuals build their professional competencies and resources. It does so efficiently and affordably.
EWOR AI's beachhead segment comprises tech entrepreneurs. As part of the prototype, we have offered individualized learning journeys for knowledge workers with initial success for one of the fastest-changing environments.
If we were to win the GM Prize on Good Jobs and Inclusive Entrepreneurship, we would use it to advance the solution's development process. The faster we can finalize the development, the sooner we can move into the distribution phase. Our vision encompasses reaching and enabling a billion learners worldwide, and we'd love to implement such a solution together with GM.
EWOR AI's content-agnostic algorithm finds many applications: It can scrape and compile Bachelor’s/Master’s degree equivalents from the web to raise literacy rates amongst adults. It can assemble skill-transformation journeys for corporate employees to equip them with the most up-to-date digital skills. EWOR AI tailors its content to the individual based on their strengths, preferences, goals, achievements, and in-app progress.
Today, even workers with decades of professional experience see their jobs wiped away by new technologies. Often, their lack of digital literacy keeps them unemployed. Our current education system, i.e., 'studying in the first part of our lives and working in the second part,' is partly responsible. Learning must become an everyday practice to cope with the fast-paced digitalized world. Paradoxically, high-quality content about new digital tools and methods is vastly available on the web, for example, via edX and KhanAcademy. EWOR's AI-powered adaptive education recommender system scrapes, orders, and presents high-quality educational content to enable everyone's continuous personalized learning.
EWOR has been in contact with a large Portuguese company regarding a potential corporate partnership. The company currently undergoes a leadership transformation and seeks to reinvent itself. They would like to use EWOR as a transformation engine for its reinvention. That implies a likely large-scale pilot in Portugal, which would help upskill workers and support company to stay at the innovation forefront.
If we won the Gulbenkian Award for Adult Literacy prize, we would use it to advance the solution's development process.
EWOR's AI-powered adaptive education recommender system connects learners with the right educational content at the right time to enable everyone's continuous personalized learning.
Today, even workers with decades of professional experience see their jobs wiped away by new technologies. Often, their lack of digital literacy keeps them unemployed. Our current education system, i.e., 'studying in the first part of our lives and working in the second part,' is partly responsible. Learning must become an everyday practice to cope with the fast-paced digitalized world.
Paradoxically, high-quality content about new digital tools and methods is vastly available on the web. EWOR's AI-powered solution scrapes, orders, and presents educational material to enable everyone's continuous personalized learning. Through that, EWOR democratizes education (by offering degree equivalents for individuals with limited access to high-quality education) and assembles skill-transformation journeys for workers to keep them up-speed with the fast-pace changes in the world.
EWOR leverages cutting-edge AI technology to benefit humanity. The solution is based on three core technologies: 1) content aggregator (gathers, modularizes, and orders publicly available education content), 2) preference discovery engine (through which the contents suit our learners' preferences, abilities, goals, and needs) and a 3) content designer (our learning management system). For the preference discovery engine we use a probabilistic matrix factorization model, such as the one used by Amazon, to identify which content has a high likelihood to be rated positively by the user. We pair our PMFs with generative, stochastic neural nets to improve performance (restricted Boltzmann machine (RBM)).
Finally, we can imagine using neuroscientific data for better learning individualization. The neuroscientific data would flow into the preference discovery engine, and alongside all the other relevant factors will help create the perfect learning journey for both students and workers.
If we were to win the AI for Humanity prize, we would use it to advance the solution's development process. The faster we can finalize the development, the sooner we can move into the commercialization phase. Our vision encompasses reaching and enabling a billion learners worldwide - both students and workers.
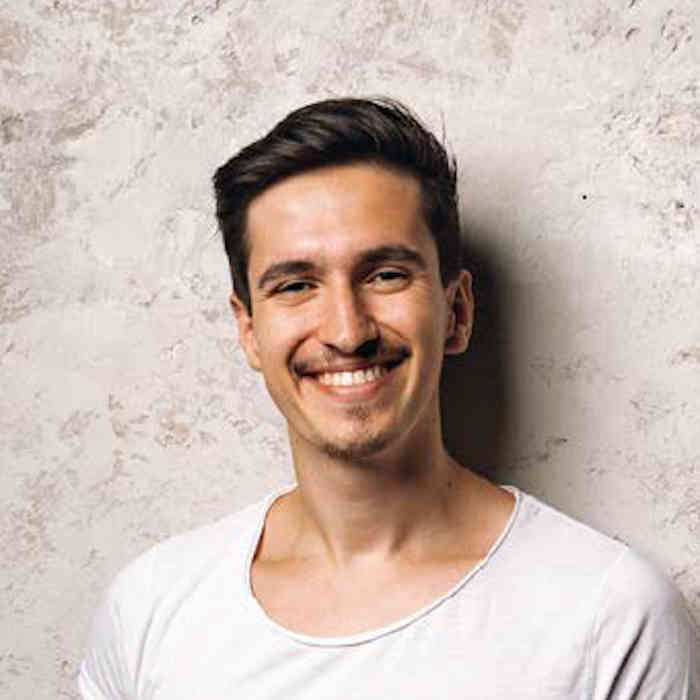
Founder