UAct Preterm Birth Predictor
About 300,000 of the 3.6 million annual preterm babies in India die due to complications. Globally, 15 million premature babies are born annually, with 80% of these in Asia and Sub-Saharan Africa having shortages of obstetricians. Primary health systems that serve the bulk of pregnancies, lack an effective and scalable preterm birth identification tool. Existing methods need a laboratory (blood/cervical fluid tests), or have poor sensitivity (cardiotocography), or they are not portable, capital-intensive and require expertise (traditional electrohysterography [EHG]).
Our solution, ‘UAct’, is a patented, wireless, portable, smartphone-integrated wearable sensor, which can auto-interpret uterine contraction data, accurately in real-time to predict preterm birth by ~26th week of pregnancy. The Solve prize will support a pilot (n~3000 rural women) of UAct through front-line staff from our existing mobile point-of-care antenatal checkups and remote-monitoring program, ‘CareMother’, to reduce the incidence or complications of prematurity, and address a long-standing maternal need.
In India, 43% of newborn deaths occur due to prematurity (also causing long-term developmental problems), which is linked to the quality of antenatal care (ANC). Studies have shown that leveraging technology to reduce antenatal service gaps, reporting results in-real time to doctors, remote medical consultation, and periodic labour monitoring can mitigate neonatal complications. However, complete ANC coverage is only 21%, and frontline workers, who serve the majority of rural pregnancies, do not have effective antenatal tools to identify the risk of premature labour.
Neonatal mortality rate in rural India is twice that in urban settings, which underscores the inequitable service access. Moreover, only 35,000 registered obstetricians working mainly in urban hospitals, are not available to the majority of pregnancies. Cardiotocography is among the existing solutions used in hospitals to detect term labour contractions, but it has a sensitivity of only 6% to predict premature birth. The traditional electrohysterography is accurate, but also bulky and expensive, and it needs substantial clincal expertise; while the modern-day blood tests and cervical fluid examinations need high-tech laboratory set-ups. Thus, only a segment of the higher-income urban women in India receive periodic uterine contraction monitoring to avoid (or better manage) a premature birth.
UAct is based on the proven technique of electrohysterography (EHG) that measures the electrical activity in the uterine muscle, thus accurately evaluating muscle behaviour closer-to-term. UAct consists of a battery-operated, wireless, portable processing unit that is connected to medical-grade adhesive (reusable) electrodes, unlike the full-fledged clinical set-up of a standard EHG. Raw signals from the electrodes are provided to the processing unit for amplification and post-processing, and transferred via Bluetooth to an Android application, which plots the graph of uterine contraction. Data is stored electronically over Cloud for remote monitoring. UAct is being validated in an urban hospital for efficacy against the standard cardiotocography.
The team is also developing a deep convolutional neural network to early identify preterm birth using the time-series based uterine contraction signal as the input. Publicly available Icelandic Electrohysterography labelled dataset is being used as the 'training' dataset. Models will be tested via the 'test' dataset generated by UAct through real patients. The most accurate model would be ported onto the smartphone application, to identify preterm labour by ~26th week of gestation. This will enable physicians/obstetricians to take prompt decisions and undertake interventions to delay the course of labour, or mitigate the complications of prematurity.
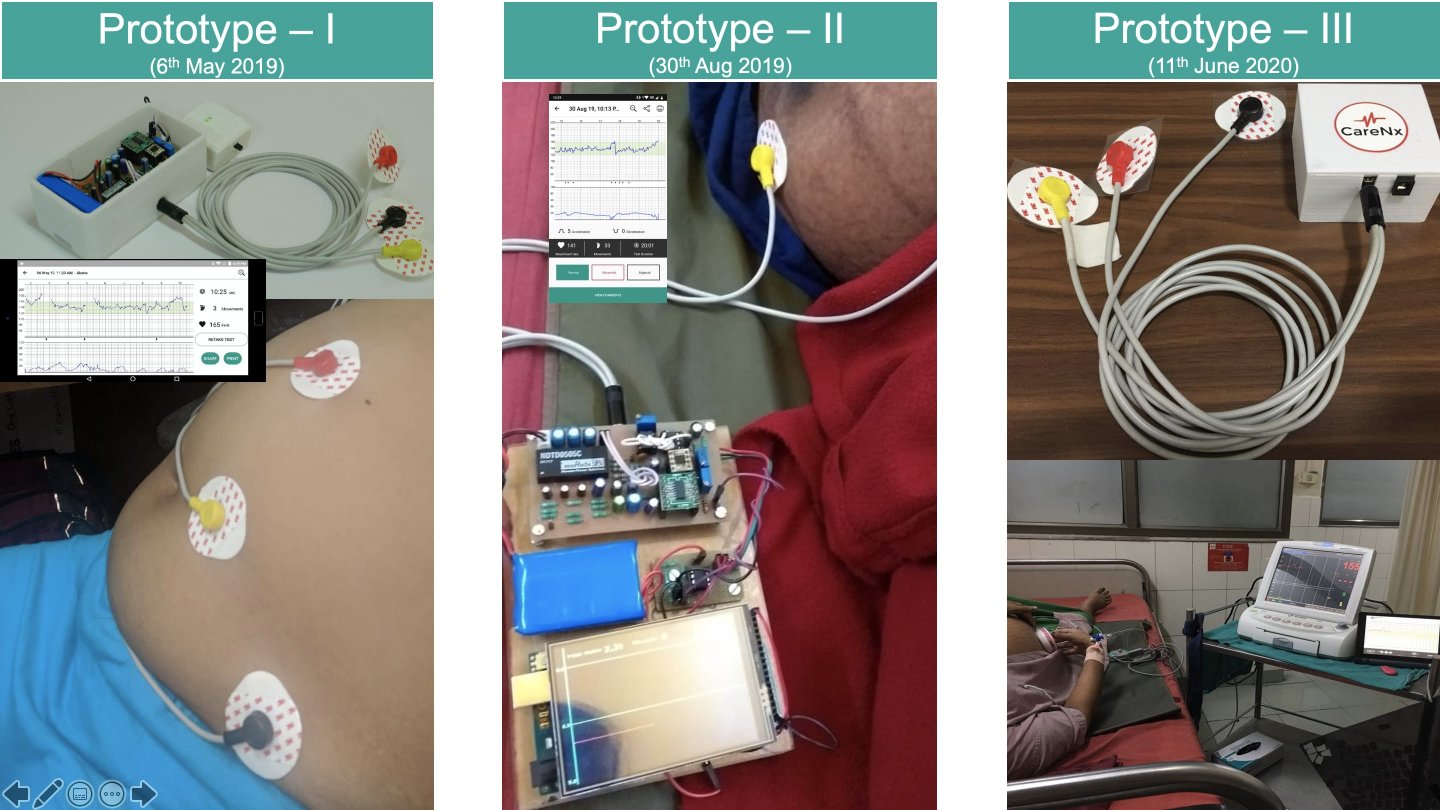
Our ‘CareMother’ program includes doorstep antenatal tests by frontline workers using portable screening kits and a smartphone application to share data with doctors in real-time. Since 2015, we have registered 30,000+ pregnancies (12000+ high-risk) via 300+ frontline workers across nine states of India, in rural, urban and tribal areas, with 15+ Government, non-profit and corporate partners. UAct will be implemented within CareMother, to focus on mothers reporting with preterm contractions, in two rural and two tribal settings (primary health centres and sub-centres). These sites include 4153 pregnancies (39.6% clinically high-risk). Indirect beneficiaries will include frontline workers and primary care doctors who will use UAct for timely management of prematurity (n~100 staff).
We conducted a needs assessment with 25 obstetricians in Mumbai and 22 rural health centres to understand the requirement of a solution having uterine contraction and fetal heart monitoring, and examined the use-case scenarios. We found that the cost and space constraints to set up conventional cardiotocography with insignificant returns, impracticality of traditional electrohysterography and the resulting absence of remote monitoring of critical uterine parameters, scarce resources for laboratories, and acute shortages of obstetricians created scope for an AI-based point-of-care sensor, and building referral linkages with remote obstetricians.
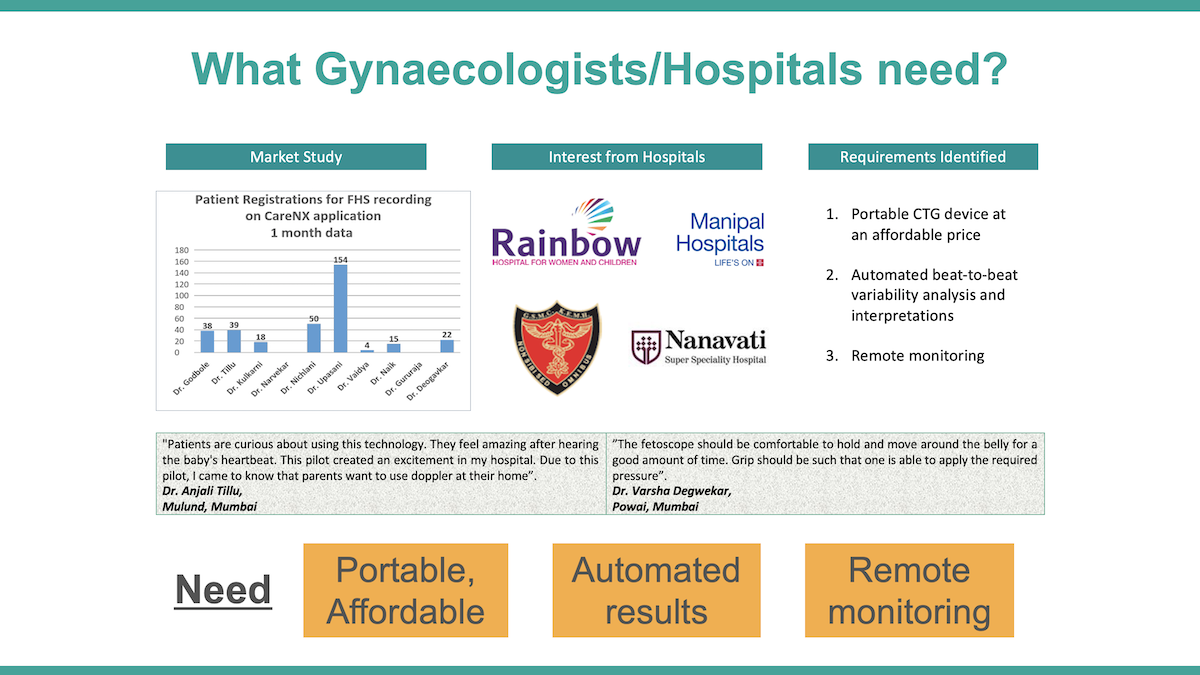
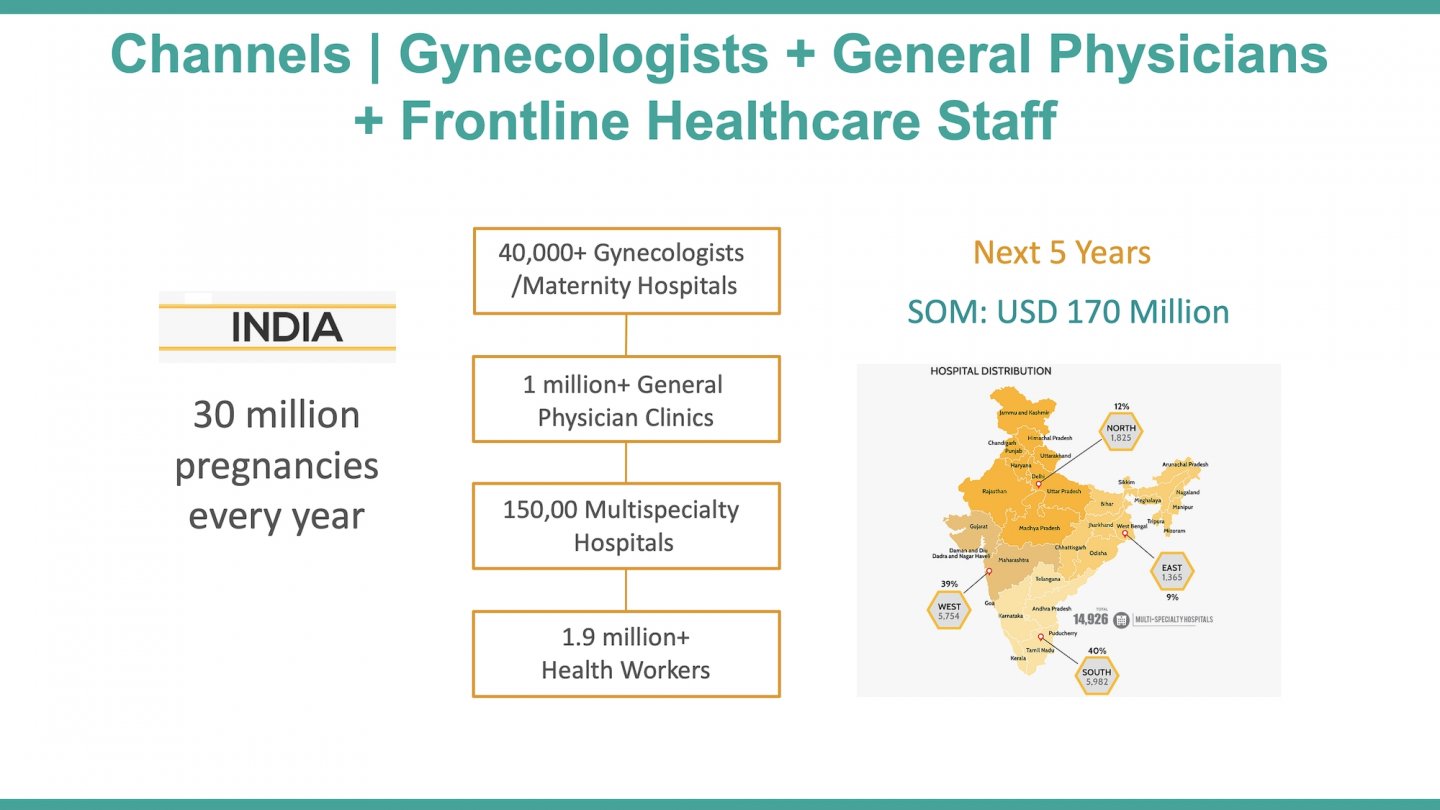
- Expand access to high-quality, affordable care for women, new mothers, and newborns
The Challenge is looking for technology-based solutions that promote the health and well-being of women, new mothers, and newborns everywhere. To that end, UAct is intended to expand the access of early identification of preterm birth and fetal distress, to improve neonatal health outcomes. The portable design makes it a viable cost-effective (40%) solution for adoption. Auto-identification of prematurity algorithm reduces the dependency on obstetricians for interpretation of results. UAct can be used by a general physician/frontline worker for decision support on-field - who are responsible for providing antenatal care to the majority of the Bottom of the Pyramid population.
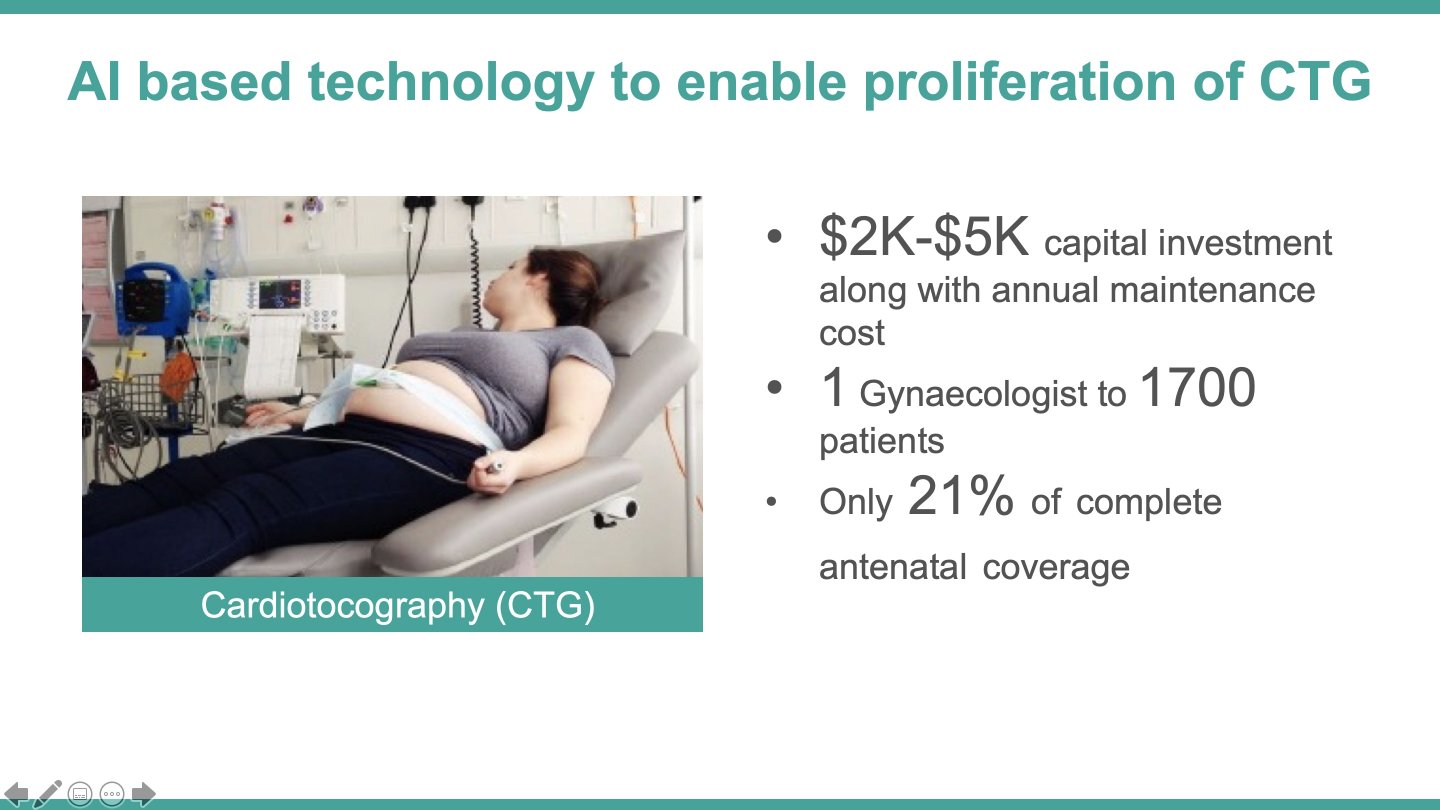
- Prototype: A venture or organization building and testing its product, service, or business model
- A new application of an existing technology
‘UAct’, is a wireless, portable wearable sensor which can auto-interpret uterine contraction data to predict preterm birth by ~26th week of pregnancy.
- UAct is based on electrohysterography (EHG) signals from abdomen to gauge progress of labour- which exhibits much more accuracy than market ready Tocodynamometry- strain gauge based monitors.
- UAct is the only device in the world which will leverage a deep convolutional neural network (CNN) classifier to enable first-level immediate prematurity interpretations with clinical grade accuracy and reduce the dependence on a gynecologist for diagnosis; this 'triages' a large number of patients. Even when EHG based newest CTG machines exist in the market (Novii GE Healthcare, Philips Avlon, Nemo Healthcare etc.), none of them provide early prematurity diagnosis- they cost somewhere from $5000-$7000- which is 7-fold more than UAct.
- Moreover, UAct has smartphone-based portability and targets ‘door-step’ community health workers, nurses and midwives and hence enables on-field diagnosis close to the mothers.
- Simple usage - health workers and midwives will only need to place a belt on the abdomen and start the device, without repositioning, and receive flagged fetal cardiac and associated risks along with prematurity identification, which they can report.
Therefore, UAct is an example of a renewed application of an existing technology with a novel algorithm, and a change in the process and business model to increase the solution's effectiveness for pregnant women.
- Uterine contractions are the outcome of uterine activity which indicate the progress of labour. Electrohysterogram (EHG) signals are non-invasive recordings of uterine contractions detected from the abdominal surface of pregnant mother. High intensity and periodic occurrence of uterine contractions before the 37th gestational week can lead to preterm birth. Therefore, these contractions leading to true labour, need to be differentiated from non-contractions (known as Braxton Hicks), termed as false labour.
- Present machine learning (ML) based techniques to early identify true labor are primarily based on the features such as Root Mean Square (RMS), median frequency (MDF) and peak frequency (PF), and non-linear features such as correlation dimension (CorrDim), sample entropy (SampEn) as they provides sufficient spectral information about the EHG contraction signal. However, the sensitivity and accuracy of such ML models suffer from the inherent limitation of feature selection techniques- in this case- given the large number and combinations of redundant and irrelevant features.
- To this end, UAct is based on a deep convolutional neural network (CNN) classifier- which is a competitive neural network capable of recognition and segmentation without need of additional feature extraction and selection- which takes time-series based abdominal EHG signal as input. The model is trained using Icelandic 16-electrode EHG database comprising of more than 14,000 segments. This classifier has achieved an accuracy of 96.25%, sensitivity of 95.08%, and specificity of 97.33% over the test dataset.
- Multiple predicate CTG devices based on EHG have been built over recent years superseding Toco (strain-gauge) technology which have FDA 501k premarket validation.
- Novii a wearable sensor by GE
- Philips Avlon
- NemoHealthcare
- Uterine contractions are the outcome of uterine activity which indicate the progress of labour. EHG signals are non-invasive recordings of uterine contractions detected from the abdominal surface of pregnant mother. High intensity and periodic occurrence of uterine contractions before the 37th gestational week can lead to preterm birth. Therefore, these contractions leading to true labour, need to be differentiated from non-contractions (known as Braxton Hicks), termed as false labour. There are numerous techniques proposed in the literature on how to distinguish Braxton Hicks from true labor and almost all of them have based upon machine learning based models which suffer greatly from inherent limitations of feature extraction and selection.
- To this end, UAct is based on a 17 layer deep convolutional neural network (CNN) classifier- which is a competitive neural network capable of recognition and segmentation without need of additional feature extraction and selection- which takes time-series based abdominal EHG signal as input. This classifier has achieved an accuracy of 96.25%, sensitivity of 95.08%, and specificity of 97.33% over the test dataset. The key insight was to make the optimisation of such a deep layer model tractable, we used residual connections and batch normalization. The depth increases both the non-linearity of the computation as well as the size of the context window for each classification decision.
- Artificial Intelligence / Machine Learning
- Biotechnology / Bioengineering
- Internet of Things
- Software and Mobile Applications
Implementation of UAct will involve the following 'Activities': a) community health workers (CHWs) screen the risk of preterm labour at homes or nearby centres; b) UAct captures data that can quantify the risk of preterm birth; c) the data is uploaded via cloud and shared with remote obstetricians; d) the obstetrician takes prompt decisions on a case-by-case basis; e) Treatment is communicated to the CHW/nearby primary health centre (PHC) doctor, who either deliver the intervention (tocolytics to delay labour, steroids for fetal lung maturation in case a premature birth is inevitable) or offer conservative treatment/monitor mothers' vital parameters, or the obstetrician asks for mother's referral to a higher centre.
The corresponding 'Inputs' include CHW training on UAct, device development with auto-interpretation capabilities, and a back-end analytics team.
The corrresponding outputs include an increased identification of high-risk conditions, greater access to quality medical care, decreased cost of seeking such care in view of the traditionally advanced assessments such as preterm risk identification, and creation of a technologically strengthened workforce.
The figure on the Logic Model provides reference data to support the linkages between outcomes and activities based on CareMother pilot studies: for example, we calculated a 33% greater identification of high-risk pregnancies after comparison with routine Government systems of surveillance in an urban slum, and a 50% decrease in healthcare costs in comparison with a medical van, based on program managers' interviews. A third-party evaluation of the CareMother program has deduced a 2x increase in access to 'quality antenatal care' at the door-step (due to the tests in the screening kit) compared to traditional Government antenatal contacts.
The corresponding outcomes include an increased incidence of identification of preterm birth and fetal distress risks and a decreased incidence of neonatal morbidity. We would need larger sample sizes (>10,000) of mothers to assess impact on neonatal mortality.
The corresponding impact level indicators overlap with some of the aforesaid outcomes, but they are measured at the population level such as neonatal mortality rate, level of third-trimester careseeking, 'escape' of mothers' risk from an untreated premature birth, and improved community-level access to remote care.
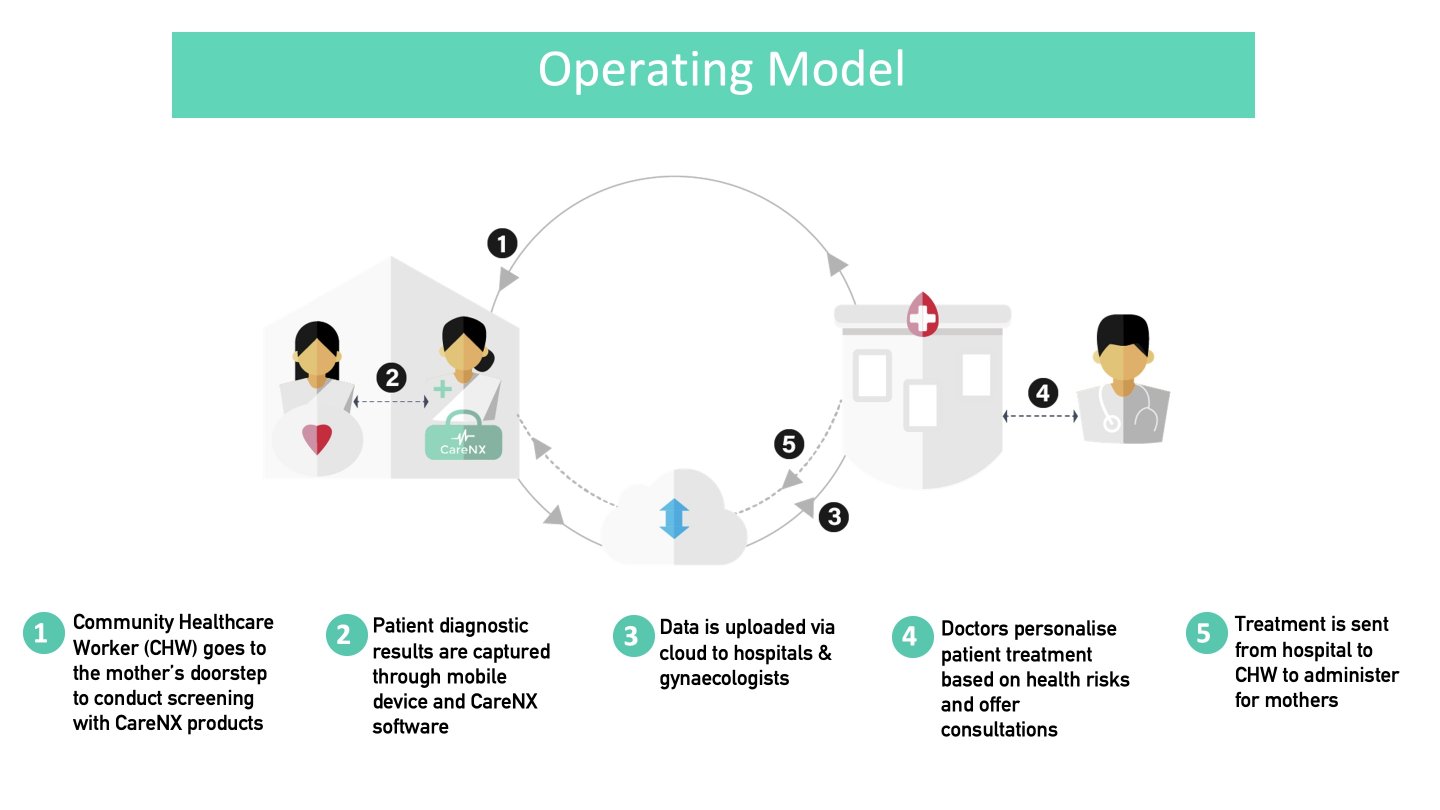
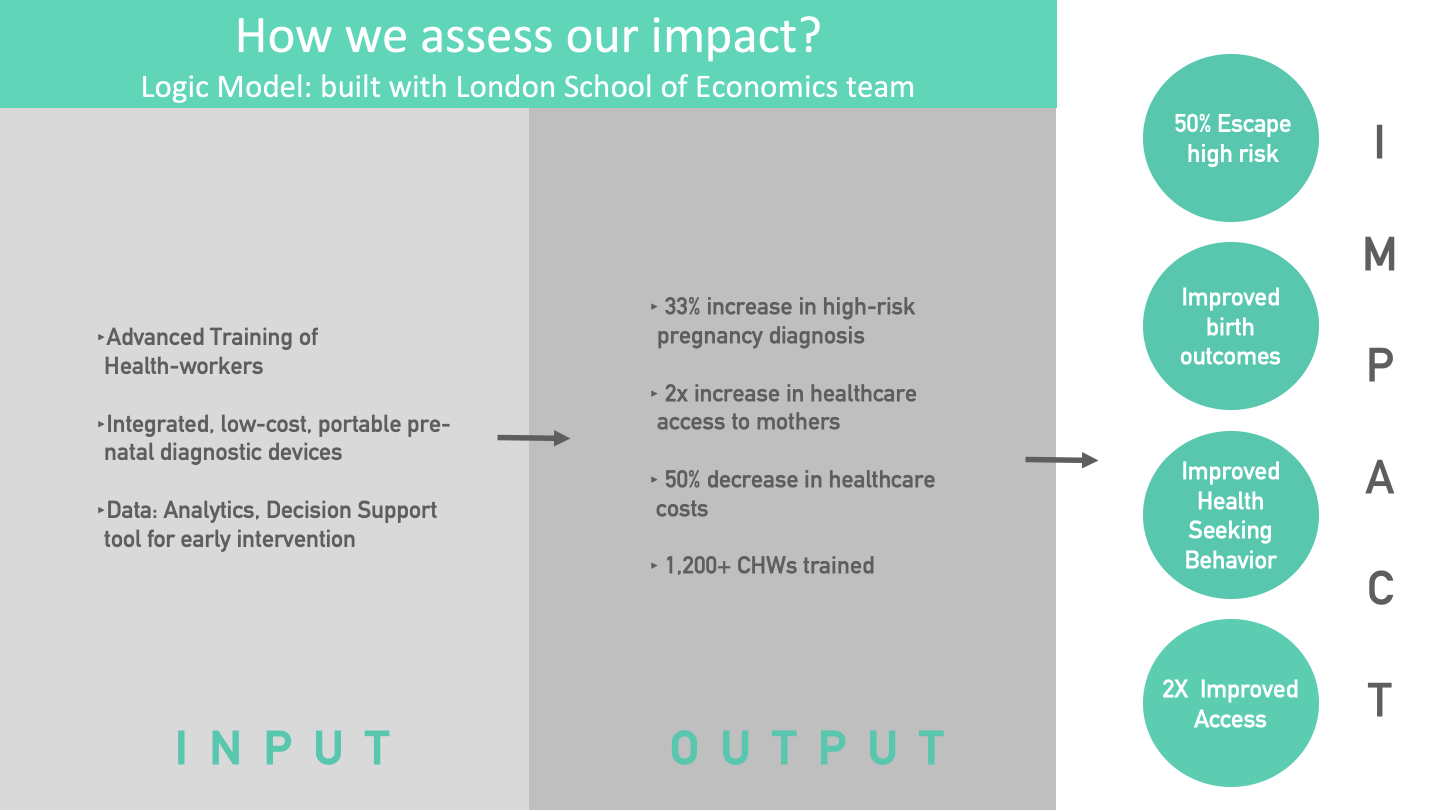
- Pregnant Women
- Infants
- Rural
- Peri-Urban
- Urban
- Poor
- Low-Income
- Middle-Income
- 3. Good Health and Well-Being
- India
- Afghanistan
- Bangladesh
- Nigeria
- Tanzania
Since 2015, ‘CareMother’ program has registered 30,000+ pregnancies(12000+ high-risk) via 260 frontline workers across nine states of India, in rural, urban and tribal areas, with 15+ Government, non-profit and corporate partners. UAct will be implemented within CareMother, to focus on mothers reporting with preterm contractions, in two rural and two tribal settings (primary health centres and sub-centres). These sites include 4153 pregnancies (39.6% clinically high-risk). Indirect beneficiaries will include frontline workers and primary care doctors who will use UAct for timely management of prematurity (n~100 staff).
Moreover, the present commercially available Fetosense solution is being used by 55+ maternity hospitals from sub-urban areas, having registered 3000+ pregnancies with a rate of 600+ pregnancies per month. UAct will be made available to selected facilities.
Considering both channels and their growth rate
- Year 1
- UAct with CareMother - rural - 100 front line staff - 3000 pregnancies
- UAct with Fetosense - semi-urban - 30 facilities - 7000 pregnancies
- Year 5
- We aim to install UAct at least 10% of maternity clinics (~5000), 1% of general physicians (~10000) and 5% of public healthcare facilities from urban and semi-urban areas (~7500)
- Considering adoption over time and monthly average coverage rate of 25 pregnancies per month - total beneficiaries will be around 6 million
- We also intend to scale UAct cross-countries, particularly south-south countries viz Bangladesh, Nepal, Kenya, Nigria and Tanzania. We already are working with partner organizations via CareMother in these countries and UAct innovation will solidify those partnerships further.
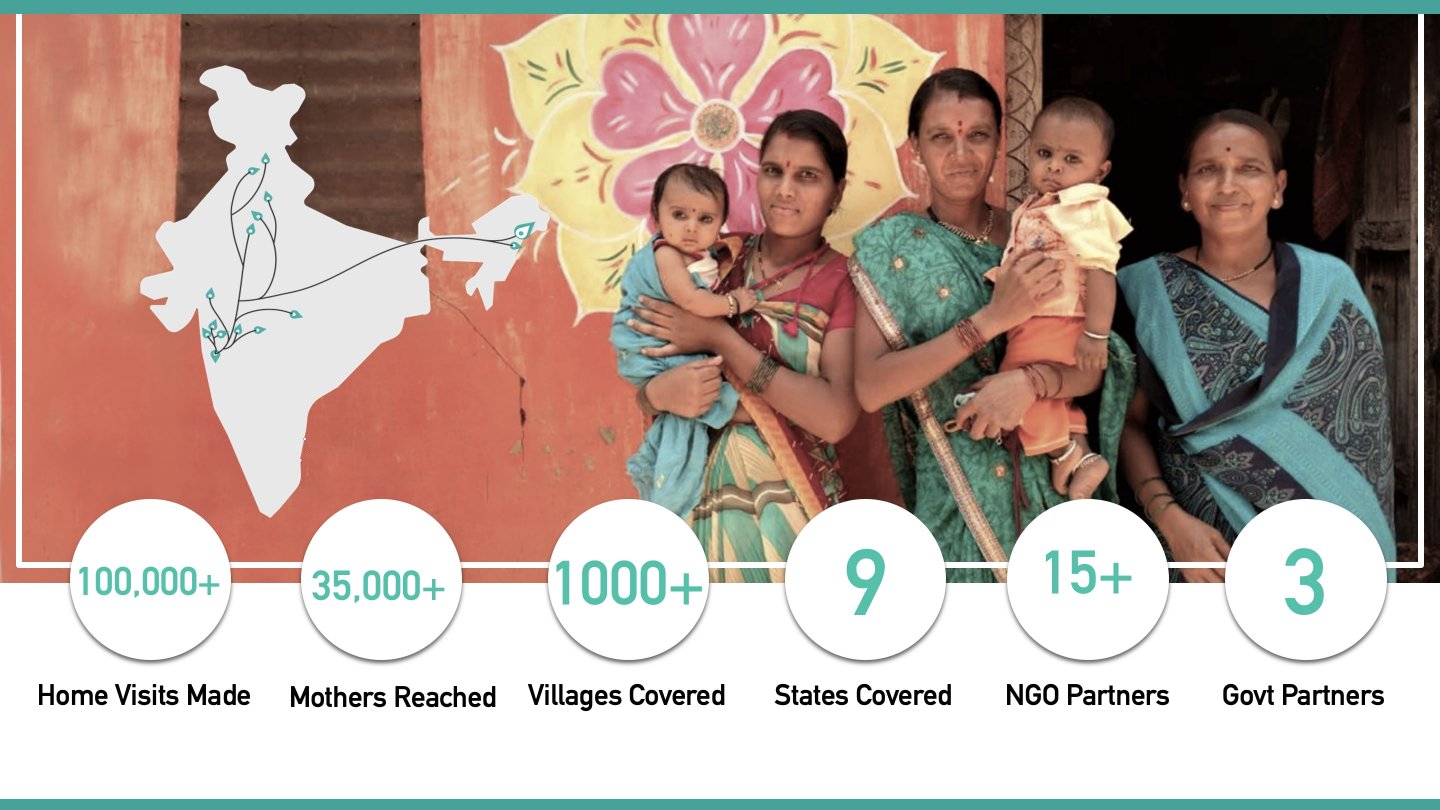
Within the next year, we aim to undertake a hospital- or clinics-based validation study over real patients in urban and rural settings to replicate the results obtained in our prior data validation in India. In parallel, we aim to complete the regulatory and compliance processes. Over a five-year period, we have the following goals:
- Undertake a two-year multi-centric (rural and urban) commercial pilot covering 14,000 pregnancies by deployment of 110 UAct devices, with its revenue-sharing business model, and leverage the strength of doctors, nurses and community health workers (CHWs) with remote monitoring by specialists.
- Leverage the results of the aforesaid pilot and collaborations to expand UAct's AI-based services to the majority of over 2 million+ frontline health workers by year-5, and forge partnerships with regional and/or central Government agencies to achieve the same.
- We aim to install UAct at least 10% of maternity clinics (~5000), 1% of general physicians (~10000) and 5% of public healthcare facilities from urban and semi-urban areas (~7500) of India. Considering adoption over time and monthly average coverage rate of 25 pregnancies per month - total beneficiaries will be around 6 million.
- Develop partnerships with companies having expertise in supply chain and distribution for mitigating scale-up barriers.
- We also intend to scale UAct cross-countries, particularly south-south countries viz Bangladesh, Nepal, Kenya, Nigria and Tanzania. We already are working with partner organizations via CareMother in these countries and UAct innovation will solidify those partnerships further.
Challenges for the next year:
- Regulatory Challenges: Medical devices can face regulatory challenges especially in cross-country settings and for performing validation studies. Necessary approvals need to be in place.
- Supply chain, local manufacturing and support: We need partner with our local manufacturing partner, establish supply chain and after sales support system in India.
Challenges for the next five years:
1. Market Challenges: Supply chain and distribution challenges will be pronounced in case of medical devices; brand building is a lengthy process and especially challenging in the medical field. Establishing distribution and support system with the per-test based revenue-sharing model will be important and herein, aligning the incentives of each party and maintaining quality of service for brand building will be crucial.
2. Operational Challenges: Queries by health staff of varying skill-sets using UAct on-the-ground will be frequent and would need to be addressed promptly, even though the solution is easy to use and has been customized for their usage. UAct users will also need to factor device calibration-related issues. Linguistic barriers will need to be resolved.
3. Financial Challenges: Sufficient data would need to be obtained on procurement and development costs and recurring material costs. Running capital cost will be required in order to install devices with recurring per-test based revenue sharing model.
- Regulatory Challenges: We have adhered to physical and metrological standardizations while developing previous Fetosense device in India, by comparing its output with a previously approved similar device. So far, we have obtained CE certification and we follow HIPPA, FHIR, ISO and NHM's health data privacy, storage and inter-operability standards for Fetosense. We will use same network and knowledge resources to get UAct regulated and compliant.
- Supply chain, local manufacturing and support: We will use the established partner network of Fetosense to manufacture UAct.
- Market Challenges: We have gained learnings from our partnership with the Federation of Obstetrical and Gynaecological Societies of India (FOGSI) and received their support for advocacy and exhibition exposure at conferences. To mitigate supply chain and distribution challenges, we will partner with medical device and pharma companies like GE's pan India distribution arm (Genworks), J&J, Zydus etc. We will promote doctor-targeted social media broadcasting for brand building.
- Operational Challenges: With a multi-lingual user manual, effective training, dedicated central team's monitoring and a helpline number, the health staff will be able to resolve any contingencies. For challenges arising out of new test configuration, the software will be remotely upgraded on the phone. In terms of calibration, UAct's self-calibration will help, as well as our continual effort to improve its accuracy relative to past performance (via back-end analytics).
- Financial Challenges: There is no direct anticipated financial risk due to a defined budget and our current funding sources. Procurement and development costs and recurring material costs have been tested.
- For-profit, including B-Corp or similar models
Full-time staff: 5
Part-time staff: 2
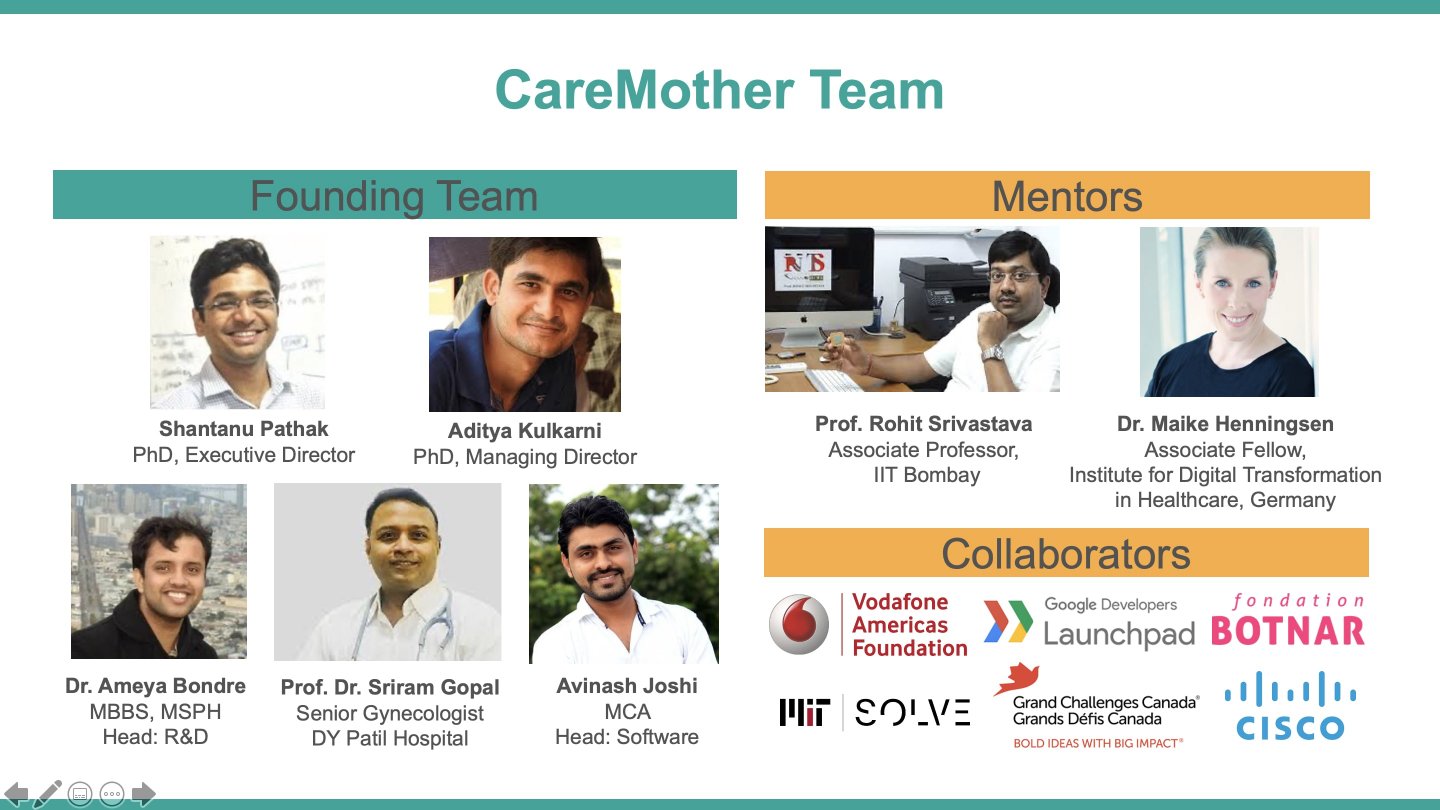
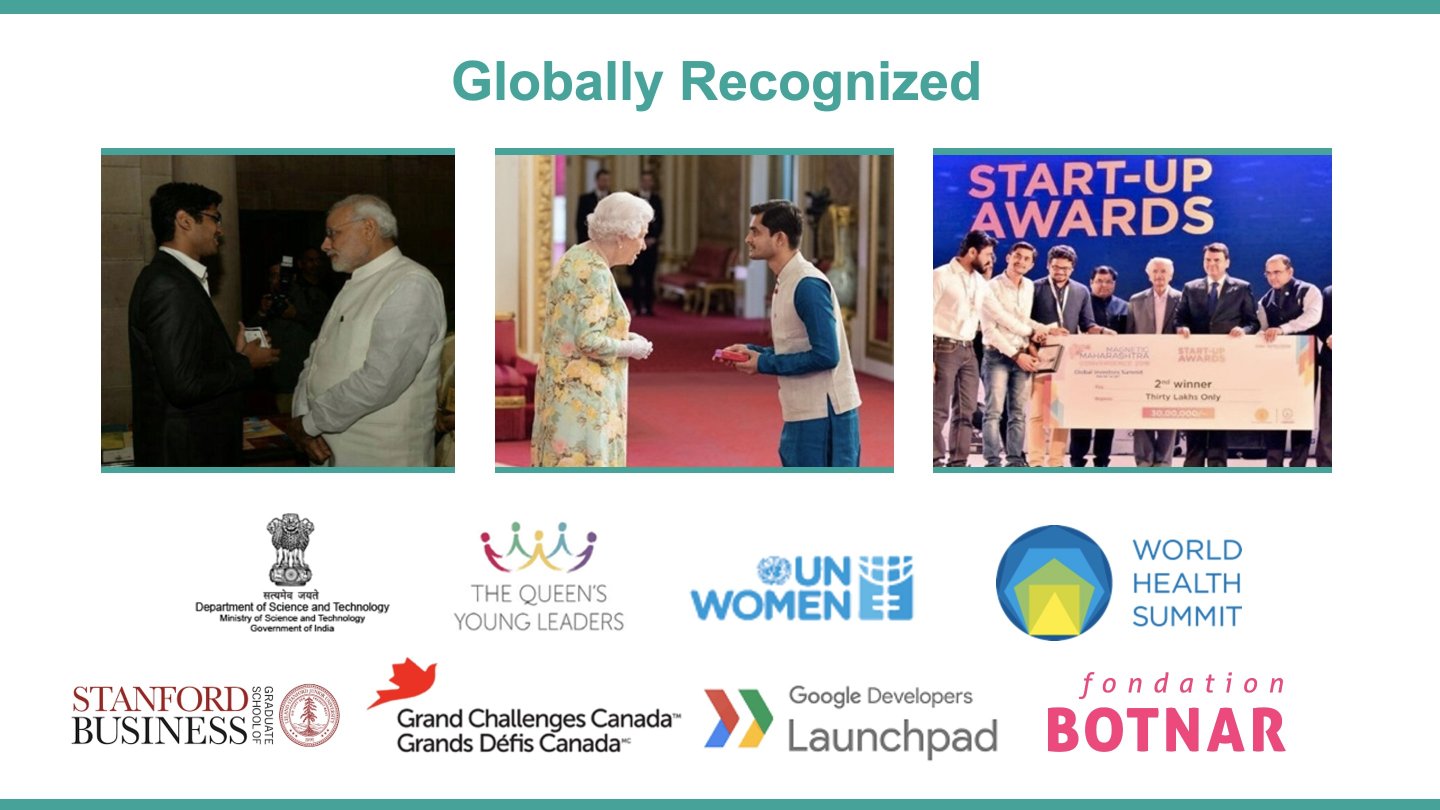
The project lead, Dr. Ameya Bondre is a public health graduate from Johns Hopkins University, Baltimore, with prior medical training from KEM Hospital, Mumbai. He is a Global Health Field Placement fellow at Hopkins with more than eight years of experience in quantitative and qualitative public health research in India, particularly in antenatal and postnatal health. Aditya and Shantanu (Founders of CareNX Innovations) have 7+ years of technology and business background, having trained at premium institutes like IITs, IIMs, Stanford, Oxford and Cambridge. The CareNX team is interdisciplinary with biomedical, engineering, computer science, clinical, and business skill-sets. Our mentors are from Indian Institute of Technology-Bombay, KEM Hospital, TATA Industries, Stanford University, Silicon Valley, and the DeepMind, UK. We have partners like the Federation of Obstetrics and Gynaecological Societies of India who launched our 'Fetosense' solution, Indian Council of Medical Research, Wadia Hospital, and KEM hospital for technical and clinical support.
In 2015, CareNX Innovations launched 'CareMother', a mobile antenatal care solution involving doorstep point-of-care testing, a smartphone application and remote monitoring by doctors. Today, this solution is being implemented in nine states of India by over 300 frontline workers serving 30,000+ pregnant women. 'Fetosense' is a wireless, portable, AI-based fetal heart monitor, which can also be used by a midwife/nurse for real-time transfer of results to the remote specialist. Since its launch in June 2019, Fetosense is now being used in 49 hospitals in India with 2072 registered mothers and 3118 fetal heart and distress monitoring tests.
CareNX Innovations currently has five groups of partners for development of artificial intelligence in preterm birth prediction and related public health research and practice:
- Indian Institute of Technology (IIT) Bombay on training in technology development (wireless sensors)
- D.Y. Patil Medical College, Hospital and Research Centre for device validation, clinical research and analysis of maternal health outcomes
- Federation of Obstetrics and Gynaecological Societies of India (FOGSI) for collaborations and linkages with doctors, hospitals and public health stakeholders.
- MIT D Lab and Dr. Richard Fletcher's team on development of artifical intelligence in UAct
- Grand Challenges Canada Stars of Global Health fund for the development of the device (from proof-of-concept to prototype)
Business model:
- Customer segments:
- Maternity Clinics
- General Physicians
- NGOs and Governments
- Pharmaceutical companies
- Value propositions:
- 7-fold reduction in the price as compared to market options
- Auto-interpretation of prematurity
- Portable & ease-of-use
- Digital record storage & remote monitoring
- Revenue streams:
- Fixed cost model: One time instalment cost + consumables (recurring) + AMC
- Pay-as-you-go model: One time deposit at instalment + Per test revenue sharing
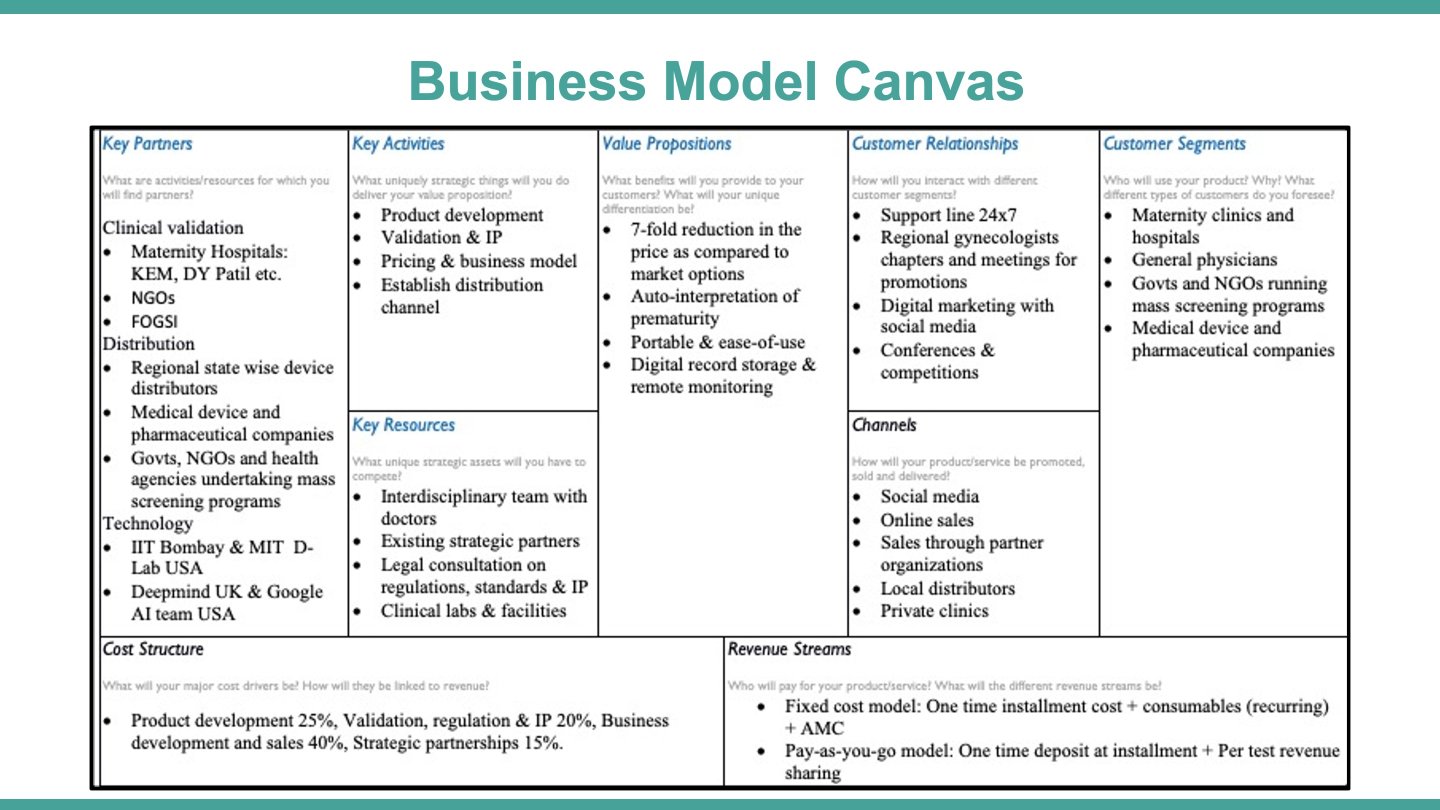
- Organizations (B2B)
Market strategy and sustainability:
- Go-to-market: With a fixed cost model, we project to sell 150 units of UAct to maternity clinics in tier-2/3 cities within year 1 of commercialisation. We will target single state of Maharashtra to begin with as we already have reach to 55+ hospitals through Fetosense. We also will leverage CareMother knowledge partner FOGSI and innovation credibility to have references for initial sale towards reputed facilities. In parallel, we will onboard state wise distributors to cover 5 states for commercialisation. Fixed cost of device is projected to return 40% of margin over the device cost, however it won't be sufficient to make UAct project self-sustainable in the first year considering operational cost involved. CareMother will rely on grant money to cover that cost.
- After the early deployment for few customers, we will roll out a pay-as-you-go model to enhance the growth. At this point we project 100 general physicians as customers with hub & spoke model. RoI on a single UAct device is expected to be 3 months- considering average volume of 40 tests per facility per month and $4 revenue per test.
- With above two approaches, UAct is projected to become sustainable with 250 device sale in one and half year with an average revenue of $1000 per device. CareMother will approach venture capitalists to enable aggressive growth thereafter.
- CareMother will cross-subsidise UAct for existing NGO/Govt partners from 9 states of India even if this channel is considered challenging in terms of turn around time.
We want CareMother and UAct to become an end-to-end solution that measures critical fetal risks and the likelihood of a premature birth, and informs the same to remote doctors in easiest possible ways via frontline workers. This will enable early intervention. To achieve that, we will need mentors from various disciplines, because our solution cuts across clinical, public health, technological and operational lines. We are confident to receive such mentoring from Solve’s diverse cross-sector community. We have prior Solver experience with our 'Fetosense' solution, embedded within the CareMother program. Mentoring is crucial as we aim to commercially validate an automated preterm birth prediction system over the next one year, and we want to avoid making technical and operational mistakes.
We would apply for the Innovation for Women Prize, AI for Humanity, BMGF Award and the Health Worker Innovation Prize as they closely relate to our work in maternal and fetal/neonatal health in challenging settings of India since 2015. If we receive funding through these prizes, we can expand our technological team to recruit more expertise in AI, Machine Learning, Data mining and Statistics, to strengthen our outcome analysis.
Since our work will involve validating a preterm birth prediction algorithm developed within a novel wireless sensor, in real-world antenatal care settings, we will also need experienced public health researchers to guide us in conducting the validation and analyzing the data. We intend to publish these results in a peer-reviewed journal to add credibility to this model, for future scale-up in India.
- Business model
- Solution technology
- Product/service distribution
- Funding and revenue model
- Board members or advisors
- Monitoring and evaluation
Below are the names of the organizations that we would like to partner with, and the details of the partnerships:
1. American International Health Alliance (AIHA): Mentoring on training and supervision of our frontline health workers under the CareMother program (who would use UAct), especially given the diversity of these workers in India (i.e. Government workers that are salaried or incentivised, workers under non-profit organizations, and those that are health facility-based)
2. Massachusetts Institute of Technology (MIT) faculty members for guidance on development and validation of artificial intelligence, wireless sensors, and preterm birth prediction
3. Johns Hopkins Bloomberg School of Public Health, for collaborating with senior public health researchers and statisticians with a focus on maternal-fetal and neonatal health
4. Women Deliver: Since this is a global advocacy organization for improving the health of women with a focus on health system strengthening, capacity-building of health workers and scale-up of evidence-based interventions, it will be useful to partner with them for communicating the results of our analysis, pitching the strengths of our solution at a global level and influencing policymakers in India and abroad through their networks.
5. Organizations and individuals that would help us pitch to the local and state agencies of the Government of India: These partnerships will be critical because we would want to scale up the solution through rural primary health centres and urban health posts, which are Government health centres manned by physicians and midwives/nurses, and closest to our beneficiaries.
Annually, 3.5 million premature babies are born in India. Widespread maternal distress is due to the deaths in these infants and the lifelong disabilities in those that survive, such as hearing, visual and learning problems. In 2005, the annual economic cost associated with preterm birth, even in the United States, was $26.2 billion, and the average hospital stay was 13 days. Therefore, women in developing countries face a catastrophic financial burden due to prematurity, particularly the rural and the urban slum populations.
Our solution, UAct, will utilize the aforesaid prize to predict premature birth and institute early intervention, to prevent prematurity or optimally manage the preterm baby in the first hours of life. UAct includes evidence-based uterine electrical activity monitoring (Verdenik, 2001) to accurately assess the pattern of uterine contractions. Since UAct is portable, wireless and AI-based, a frontline worker can use it to share uterine activity data with a remote doctor/obstetrician, via a smartphone. Thus, the doctor can take prompt decisions and advise on administration of tocolytic medications to prolong pregnancy and prevent prematurity, or give corticosteroids for lung maturation if the delivery should be done. The framework of AI-powered UAct, within our mobile antenatal care program (CareMother) will allow frontline workers to flag the possibility of premature birth and facilitate early intervention at the ~25,650 primary health centres in rural India.
The prize will support the commercial pilot (n~3000 rural women) of UAct, to reduce the incidence or complications of prematurity, and address a long-standing maternal need.
Most of the annual >40 million pregnancies in India are served by midwives at the ~150,000 subcentres and the physicians at ~25,600 primary health centres (rural). However, even the basic antenatal services reach only 21% of women (100 iron folic-acid tablets, four check-ups and one tetanus injection). Frontline workers lack sophisticated assessment and referral tools, especially for complications like preterm births that contribute to 43% of neonatal deaths in India. Existing technologies have poor accuracy, and they are expensive, not portable, and need clinical expertise. Therefore, most preterm births are delivered as it is, or managed with sub-optimal care at the grass-root level, or referred to far-off remote obstetricians (only ~30,000 in India) incurring delays in the process.
UAct is an evidence-based, wireless, portable, smartphone-integrated sensor, with AI-based capabilities to auto-interpret uterine contraction data, which can flag the risk of premature birth. Therefore, it can be used by a frontline worker, to relay the uterine contraction results to a remote obstetrician, for receiving prompt medical advice on preventing preterm labour, or instituting measures to reduce complications in a premature baby (by medications). Thus, UAct can transform the assessment capabilities of the massive frontline cadres of India to reduce the incidence/complications of preterm births (Note: 300,000 babies die annually in India due to prematurity).
Through the aforesaid prize, we aim to commercially validate UAct among 3000 rural pregnancies and in the process, train the 300+ frontline workers under our 'CareMother' program, which will be a value-addition to their job responsibilities.
Problem statement:
Preterm birth and its complications (such as asphyxia) contribute to more than half of the neonatal deaths in India, and these complications have roots in sub-optimal antenatal care. In spite of vast improvements in newborn survival in India, 3.5 million babies are born premature every year, and 10% of these, die due to complications. India's newborn mortality rate cannot reduce until prematurity, which contributes to 43% of newborn deaths, is addressed. Prematurity also poses long-term morbidities (such as developmental and cognitive-neurological deficits) in infants that survive.
While this situation is compounded by a scarce number of obstetricians in India (only ~30,000 registered), there is an untapped potential in the massive frontline workforce that manages the ~150,000 sub-centres (Auxiliary Nurse Midwives) and the ~25,650 primary health centres (physicians). These cadres attend to the majority of the annual >40 million pregnancies, but do not have sophisticated tools to identify the risk of premature birth at an early stage. Therefore, a premature birth is not effectively managed or delivered as it is at the grass-root level, and remote consultation with the far-off obstetricians is not leveraged. Part of the problem is the complexity of existing solutions such as the traditional electrohysterography that is practically absent in India (lack of portability, high cost, and usage requiring clinical expertise) and the poor sensitivity of current tools in predicting preterm birth, as in case of cardiotocography.
Our solution:
AI/ML plays a crucial role given the above scenario – frontline workers need an accurate and efficient tool that can enable them to identify the risk of a preterm birth, without the effort of manual interpretation, and remote obstetricians should provide prompt medical advice based on the data provided by such a tool. To meet this need, we have developed, UAct: a wireless, portable, evidence-based, non-invasive, smartphone-integrated sensor, which can assess the electrical activity of the uterine muscle. Based on the proven principle of electrohysterography (Verdenik et al, 2001), UAct can be placed on the maternal abdomen as a wearable (without maternal skin abrasion or preparation as needed in traditional electrohysterography) and measure uterine electrical signals to characterize their contraction patterns, correlating with the risk of preterm birth. Therefore, frontline workers can use UAct and report the prematurity risk via a smartphone application to a remote obstetrician, who can then convey critical decisions to delay/better manage a premature birth, or its complications (by medications).
We are building AI/ML capabilities in UAct based on evidence in literature, by developing a deep convolutional neural network to early identify preterm birth, using the time-series based uterine contraction signal as the input. Publicly available Icelandic Electrohysterography labelled dataset is being used as the training dataset. Models will be tested via the test dataset generated by UAct through real patients. The most accurate model would be ported onto the smartphone application, to identify preterm labour by ~26th week of gestation. This will enable physicians and obstetricians to take early decisions and undertake interventions to delay the course of labour, or mitigate the complications of prematurity.
In addition, we are also building autointerpretation capabilities using the established Dawes-Redman criteria, in our existing, validated fetal heart monitor, Fetosense (incorporated within our CareMother program, MIT Solver 2019), to identify fetal distress at an early stage, which contributes to neonatal asphyxia. Fetosense has similar operational advantages as UAct and it can be easily used by frontline workers. In fact, since its launch in June 2019, Fetosense is now being used in 49 hospitals in India with 2072 registered mothers, and 3118 fetal heart and distress monitoring tests have been completed.
Taken together, UAct and Fetosense will become a single, integrated end-to-end solution to empower frontline workers at primary health centres and subcentres in India for early identification of prematurity, for early intervention by remote doctors/obstetricians. We are positioned to validate this integrated solution within our existing CareMother program, through a team of 300+ frontline workers deploying door-step point-of-care antenatal tests and using a smartphone application for remote consultation with doctors. CareMother is currently being implemented in nine states of India, serving 30,000+ pregnant women, with 15+ government, non-profit and corporate implementing partners.
Utilization of the Prize:
Therefore, the AI for Humanity prize comes at a critical time for our team to further strengthen the auto-interpretaton capacity of the integrated solution of UAct and Fetosense, validate it commercially with a large population of rural pregnancies (planned n=3000) and evaluate neonatal health outcomes. The prize would also enable the training and capacity-building of our CareMother frontline workers to use sophisticated tools such as UAct+Fetosense, within the scope of the CareMother program's 'doorstep' (i.e. home-based or nearby centre-based) antenatal tests.
Through this prize, we aim to bridge the acute gaps in coverage and quality of antenatal care services, by integrating solutions that are designed to reduce prematurity and asphyxia in India.
Problem statement:
Preterm birth and its complications (such as asphyxia) contribute to more than half of the neonatal deaths in India, and these complications have roots in sub-optimal antenatal care. In spite of vast improvements in newborn survival in India, 3.5 million babies are born premature every year, and 10% of these, die due to complications. India's newborn mortality rate cannot reduce until prematurity, which alone contributes to 43% of newborn deaths, is addressed. Prematurity also poses long-term morbidities (such as developmental and cognitive-neurological deficits) in infants that survive. While this situation is compounded by a scarce number of obstetricians in India (only ~30,000 registered), there is an untapped potential in the massive frontline workforce of the public sector that manages the ~150,000 sub-centres (Auxiliary Nurse Midwives) and the ~25,650 primary health centres (physicians). These cadres attend to the majority of the annual >40 million pregnancies, but do not have sophisticated tools to identify the risk of premature birth at an early stage. Therefore, premature births are not effectively managed at the grass-root level, and remote consultation with the far-off obstetricians is not leveraged. Part of the problem is the complexity of existing solutions such as the traditional electrohysterography that is practically absent in India (lack of portability, high cost, and usage requiring clinical expertise) and the poor sensitivity of current tools in predicting preterm birth, as in case of cardiotocography.
Proposed Solution:
AI/ML plays a crucial role given the above scenario – frontline workers need an accurate and efficient tool that can enable them to identify the risk of a preterm birth, without the effort of manual interpretation, and remote obstetricians should provide prompt medical advice based on the data provided by such a tool.
To meet this need, we have developed 'UAct', a wireless, portable, evidence-based, non-invasive, smartphone-integrated sensor, which can assess the electrical activity of the uterine muscle. Based on the proven principle of electrohysterography (Verdenik et al, 2001), UAct can be placed on the maternal abdomen as a wearable (without maternal skin abrasion or preparation as needed in traditional electrohysterography) and measure uterine electrical signals to characterize their contraction patterns, correlating with the risk of preterm birth. Therefore, frontline workers can use UAct and report the prematurity risk via a smartphone application to a remote obstetrician, who can then convey critical decisions to delay/better manage a premature birth, or its complications (for example, by medications).
We are building AI/ML capabilities in UAct based on evidence in literature, by developing a deep convolutional neural network to early identify preterm birth, using the time-series based uterine contraction signal as the input. Publicly available Icelandic Electrohysterography labelled dataset is being used as the 'training dataset'. Models will be tested via the 'test dataset' generated by UAct through real patients. The most accurate model would be ported onto the smartphone application, to identify preterm labour by ~26th week of gestation. This will enable physicians and obstetricians to take early decisions and undertake interventions to delay the course of labour, or mitigate the complications of prematurity.
In addition, we are also building autointerpretation capabilities using the established Dawes-Redman criteria, in our existing, validated fetal heart monitor, Fetosense (MIT Solver 2019), to identify fetal distress at an early stage, which contributes to neonatal asphyxia. Fetosense has similar operational advantages as UAct and it can be easily used by frontline workers. Since its launch in June 2019, Fetosense is now being used in 49 hospitals in India (by nurses and obstetricians) with 2072 registered mothers and 3118 fetal heart and distress monitoring tests.
Taken together, UAct and Fetosense will become a single, integrated end-to-end solution to empower frontline workers across primary health centres and subcentres in India for early identification of prematurity, for early intervention by remote doctors/obstetricians.
Linkage of 'CareMother' with government agencies:
We are positioned to validate this integrated solution (UAct+Fetosense)
within our existing CareMother program, through a team of 300+ frontline workers (Auxiliary Nurse Midwives [ANMs] and ASHAs in the public sector, and independent community cadres under non-profits) deploying door-step point-of-care antenatal tests, and using a smartphone application for remote consultation with doctors. CareMother is currently being implemented in nine states of India with 15+ government, non-profit and corporate implementing partners.
CareMother aims to improve the quality of the traditional antenatal check-up conducted under Government programs, and works with implementing partners to achieve the same. The traditional visits involve counseling on health and nutrition practices and assessment of basic parameters (height, weight, pulse and blood pressure). These visits are supposed to have screening tests (for antenatal high-risk conditions) at sub-centres and primary health centres, but often have a varying availability in these tests, partly contributed by inadequate supplies. Moreover, these visits are devoid of a digital 'high-risk case management system', tracking the high-risk disorders over time and remote consultation by doctors - and they are consequently lacking more advanced tests such as preterm birth and fetal risk identification.
CareMother aims to bridge this critical service gap via three ‘mobile’ components: the moving grass-roots level community-based health worker, a portable screening kit having essential antenatal test material (for measuring mother's height, weight, haemoglobin, blood sugar, blood pressure, urine protein and sugar, fundal height, temperature, pulse-oxygenation levels and fetal heart rate), and a smartphone application to store and transfer data in real-time to primary care doctors. CareMother performs these tests both at homes (tribal) and nearby centres (rural, urban) based on the context. And, CareMother, as discussed above, can incorporate UAct+Fetosense within its programmatic activites.
CareMother’s framework shifts antenatal high-risk identification and management from the facility i.e. a relatively far-off primary health center or hospital, to the ‘door-step’ i.e. homes/nearby sub-centers (rural) or health posts (urban). The structure and format of the CareMother application, case-management protocols and content of the screening kit are based on existing protocols of the Indian Government's National Health Mission (NHM). In order to flag high-risk pregnancies for remote consultation and management, the CareMother application uses the Pradhan Mantri Matritva Suraksha Abhiyan’s (PMSMA) high-risk identification criteria, an initiative by the Government of India based on WHO guidelines.
Therefore, under the ambit of CareMother, UAct+Fetosense is in a strong position to meet the persisting need of an early identification and intervention system for addressing prematurity and birth asphyxia, and reduce neonatal morbidity and mortality. The existing integration of CareMother with the Government health system reinforces our position to expand services provided by UAct+Fetosense (Refer enclosed figure).
Utilisation of BMGF funded award:
The Bill and Melinda Gates Foundation funded award comes at a critical time for our team to further strengthen the auto-interpretaton capacity of the integrated solution of UAct and Fetosense, and validate it commercially with a population of rural and tribal pregnancies - with an initial targeted sample of 3000, subject to further expansion for assessment of outcomes such as neonatal morbidity and mortality.
The award would also enable the training and capacity-building of our CareMother frontline workers (currently 300+) to use sophisticated tools such as UAct+Fetosense, within the scope of the CareMother program's doorstep (home-based or nearby centre-based) antenatal tests. These workers predominantly include the Government staff (ANMs and ASHAs).
Through this award, we aim to bridge the acute gaps in coverage and quality of antenatal care services, and integrate solutions that are designed to reduce neonatal prematurity and asphyxia in India.
Global challenge:
Prematurity is the reason why newborn babies continue to die and suffer health and developmental risks in the long-term, causing substantial healthcare expenditure for families and severe maternal distress, disproportionately affecting the lower socio-economic segments. India contributes to ~25% of the global preterm births (~15 million annual preterm neonates worldwide).
Problem statement:
Preterm birth and its complications (such as birth asphyxia) contribute to more than half of the neonatal deaths in India, and these complications have roots in sub-optimal antenatal care. In spite of vast improvements in newborn survival in India, >3.5 million babies are born premature every year, and 10% of these, die due to complications. India's newborn mortality rate cannot reduce until prematurity, which alone contributes to 43% of newborn deaths, is addressed. Prematurity also poses long-term morbidities (such as developmental and cognitive-neurological deficits) in infants that survive. While this situation is compounded by a scarce number of obstetricians in India (only ~30,000 registered), there is an untapped potential in the massive frontline workforce of the public sector that manages the ~150,000 sub-centres (Auxiliary Nurse Midwives) and the ~25,650 primary health centres (physicians). These cadres attend to the majority of the annual >40 million pregnancies, but do not have sophisticated tools to identify the risk of premature birth at an early stage. Therefore, premature births are not effectively managed at the grass-root level, and remote consultation with the far-off obstetricians is not leveraged. Part of the problem is the complexity of existing solutions such as the traditional electrohysterography that is practically absent in India (lack of portability, high cost, and usage requiring clinical expertise) and the poor sensitivity of current tools in predicting preterm birth, as in case of cardiotocography.
Proposed Solution:
AI/ML plays a crucial role given the above scenario – frontline workers need an accurate and efficient tool that can enable them to identify the risk of a preterm birth, without the effort of manual interpretation, and remote obstetricians should provide prompt medical advice based on the data provided by such a tool.
To meet this need, we have developed 'UAct', a wireless, portable, evidence-based, non-invasive, smartphone-integrated sensor, which can assess the electrical activity of the uterine muscle. Based on the proven principle of electrohysterography (Verdenik et al, 2001), UAct can be placed on the maternal abdomen as a wearable (without maternal skin abrasion or preparation as needed in traditional electrohysterography) and measure uterine electrical signals to characterize their contraction patterns, correlating with the risk of preterm birth. Therefore, frontline workers can use UAct and report the prematurity risk via a smartphone application to a remote obstetrician, who can then convey critical decisions to delay/better manage a premature birth, or its complications (for example, by medications).
We are building AI/ML capabilities in UAct based on evidence in literature, by developing a deep convolutional neural network to early identify preterm birth, using the time-series based uterine contraction signal as the input. Publicly available Icelandic Electrohysterography labelled dataset is being used as the 'training dataset'. Models will be tested via the 'test dataset' generated by UAct through real patients. The most accurate model would be ported onto the smartphone application, to identify preterm labour by ~26th week of gestation. This will enable physicians and obstetricians to take early decisions and undertake interventions to delay the course of labour, or mitigate the complications of prematurity.
In addition, we are also building autointerpretation capabilities using the established Dawes-Redman criteria, in our existing, validated fetal heart monitor, Fetosense (MIT Solver 2019), to identify fetal distress at an early stage, which contributes to neonatal asphyxia. Fetosense has similar operational advantages as UAct and it can be easily used by frontline workers. Since its launch in June 2019, Fetosense is now being used in 49 hospitals in India (by nurses and obstetricians) with 2072 registered mothers and 3118 fetal heart and distress monitoring tests.
Taken together, UAct and Fetosense will become a single, integrated end-to-end solution to empower frontline workers across primary health centres and subcentres in India for early identification of prematurity, for early intervention by remote doctors/obstetricians.
Linkage of 'CareMother' with government agencies:
We are positioned to validate this integrated solution (UAct+Fetosense) within our existing CareMother program, through a team of 300+ frontline workers (Auxiliary Nurse Midwives [ANMs] and ASHAs in the public sector, and independent community cadres under non-profits) deploying door-step point-of-care antenatal tests, and using a smartphone application for remote consultation with doctors. CareMother is currently being implemented in nine states of India with 15+ government, non-profit and corporate implementing partners.
CareMother aims to improve the quality of the traditional antenatal check-up conducted under Government programs, and works with implementing partners to achieve the same. The traditional visits involve counseling on health and nutrition practices and assessment of basic parameters (height, weight, pulse and blood pressure). These visits are supposed to have screening tests (for antenatal high-risk conditions) at sub-centres and primary health centres, but often have a varying availability in these tests, partly contributed by inadequate supplies. Moreover, these visits are devoid of a digital 'high-risk case management system', tracking the high-risk disorders over time and remote consultation by doctors - and they are consequently lacking more advanced tests such as preterm birth and fetal risk identification.
CareMother aims to bridge this critical service gap via three ‘mobile’ components: the moving grass-roots level community-based health worker, a portable screening kit having essential antenatal test material (for measuring mother's height, weight, haemoglobin, blood sugar, blood pressure, urine protein and sugar, fundal height, temperature, pulse-oxygenation levels and fetal heart rate), and a smartphone application to store and transfer data in real-time to primary care doctors. CareMother performs these tests both at homes (tribal) and nearby centres (rural, urban) based on the context. And, CareMother, as discussed above, can incorporate UAct+Fetosense within its programmatic activites.
CareMother’s framework shifts antenatal high-risk identification and management from the facility i.e. a relatively far-off primary health center or hospital, to the ‘door-step’ i.e. homes/nearby sub-centers (rural) or health posts (urban). The structure and format of the CareMother application, case-management protocols and content of the screening kit are based on existing protocols of the Indian Government's National Health Mission (NHM). In order to flag high-risk pregnancies for remote consultation and management, the CareMother application uses the Pradhan Mantri Matritva Suraksha Abhiyan’s (PMSMA) high-risk identification criteria, an initiative by the Government of India based on WHO guidelines.
Therefore, under the ambit of CareMother, UAct+Fetosense is in a strong position to meet the persisting need of an early identification and intervention system for addressing prematurity and birth asphyxia, and reduce neonatal morbidity and mortality. The existing integration of CareMother with the Government health system reinforces our position to expand services provided by UAct+Fetosense (Refer enclosed figure).
Utilisation of BMGF funded award:
The Bill and Melinda Gates Foundation funded award comes at a critical time for our team to further strengthen the auto-interpretaton capacity of the integrated solution of UAct and Fetosense, and validate it commercially with a population of rural and tribal pregnancies - with an initial targeted sample of 3000, subject to further expansion for assessment of outcomes such as neonatal morbidity and mortality.
The award would also enable the training and capacity-building of our CareMother frontline workers (currently 300+) to use sophisticated tools such as UAct+Fetosense, within the scope of the CareMother program's doorstep (home-based or nearby centre-based) antenatal tests. These workers predominantly include the Government staff (ANMs and ASHAs).
Through this award, we aim to bridge the acute gaps in coverage and quality of antenatal care services, and integrate solutions that are designed to reduce neonatal prematurity and asphyxia in India.
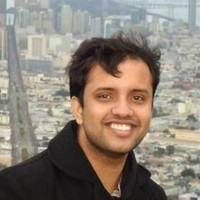
Head of Clinical Research and Development
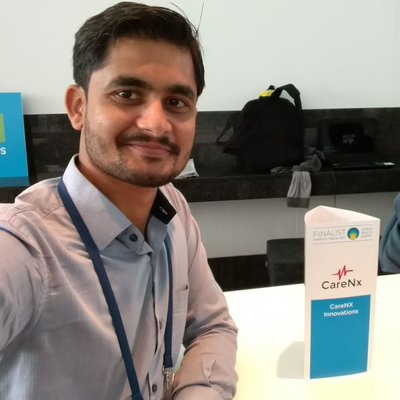
Founder & CEO